Applications of Recurrent Neural Network Language Model in Offline Handwriting Recognition and Word Spotting
ICFHR(2014)
摘要
The recurrent neural network language model (RNNLM) is a discriminative, non-Markovian model that can capture long-span word history in natural language. It has been proved to be successful in automatic speech recognition and machine translation. In this work, we applied RNNLM to the n-best rescoring stage of the state-of-the-art BBN Byblos OCR (optical character recognition) system for handwriting recognition.1 With RNNLM scores as additional features, our system achieved significant improvement (p <; 0.001), a 3.5% relative reduction on OCR word error rate, compared with a high baseline that uses n-gram language model for rescoring. We have also developed a novel method to integrate the OCR n-best RNNLM scores into the word posterior probabilities in OCR confusion networks, which resulted in consistent observable improvements in word spotting for OCR'ed handwritten documents, as measured by both mean average precision (MAP) and detection-error tradeoff (DET) curves.
更多查看译文
关键词
keyword search,nonMarkovian model,recurrent neural network language model,optical character recognition system,OCR handwritten documents,word processing,OCR word error rate,information retrieval,recurrent neural networks,DET curves,BBN Byblos OCR,word posterior probabilities,language translation,detection-error tradeoff curves,optical character recognition,recurrent neural nets,long-span word history,offline handwriting recognition,RNNLM scores,handwriting recognition,word spotting,OCR confusion networks,document image processing,machine translation,MAP,mean average precision,automatic speech recognition
AI 理解论文
溯源树
样例
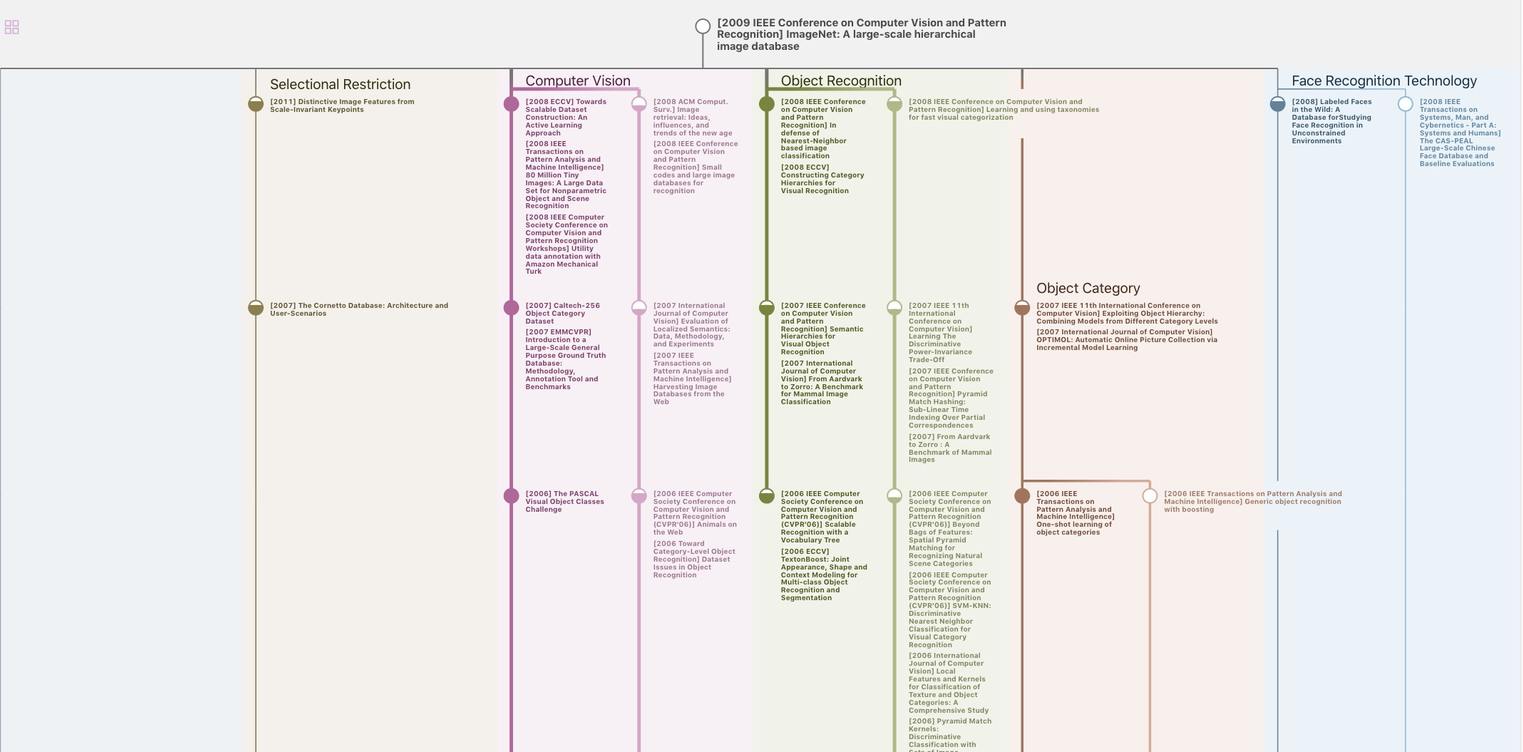
生成溯源树,研究论文发展脉络
Chat Paper
正在生成论文摘要