Generative versus Discriminative Prototype Based Classification.
ADVANCES IN SELF-ORGANIZING MAPS AND LEARNING VECTOR QUANTIZATION(2014)
摘要
Prototype-based models such as learning vector quantization (LVQ) enjoy a wide popularity because they combine excellent classification and generalization ability with an intuitive learning paradigm: models are represented by few characteristic prototypes, the latter often being located at class typical positions in the data space. In this article we investigate inhowfar these expectations are actually met by modern LVQ schemes such as robust soft LVQ and generalized LVQ. We show that the mathematical models do not explicitly optimize the objective to find representative prototypes. We demonstrate this fact in a few benchmarks. Further, we investigate the behavior of the models if this objective is explicitly formalized in the mathematical costs. This way, a smooth transition of the two partially contradictory objectives, discriminative power versus model representativity, can be obtained.
更多查看译文
关键词
Cost Function, Gaussian Mixture Model, Generalization Ability, Learn Vector Quantization, Model Representativity
AI 理解论文
溯源树
样例
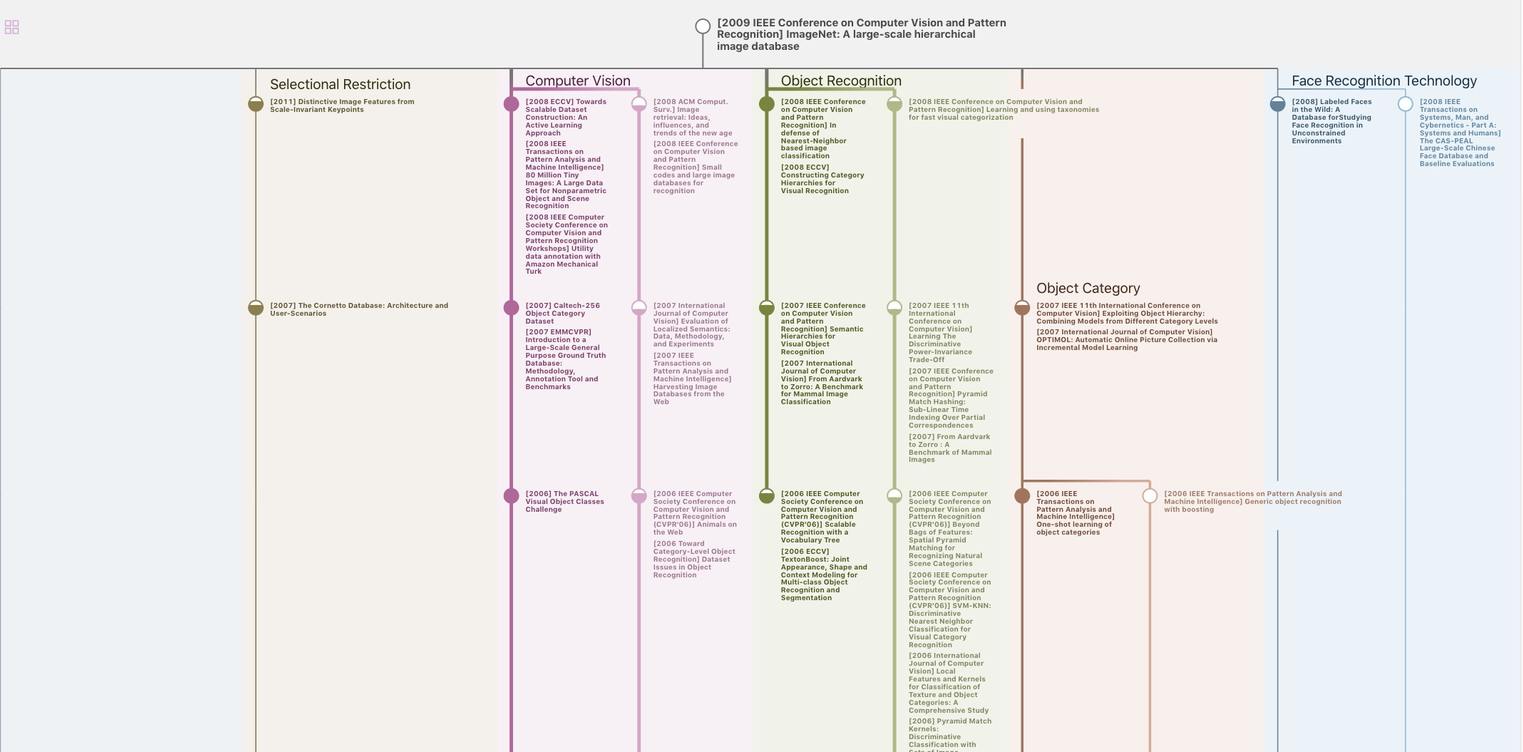
生成溯源树,研究论文发展脉络
Chat Paper
正在生成论文摘要