Object shape categorization in RGBD images using hierarchical graph constellation models based on unsupervisedly learned shape parts described by a set of shape specificity levels
Intelligent Robots and Systems(2014)
摘要
We present an approach for object class learning using a part-based shape categorization in RGB-augmented 3D point clouds captured from cluttered indoor scenes with a Kinect-like sensor. We propose an unsupervised hierarchical learning procedure which allows to symbolically classify shape parts by different specificity levels of detailedness of their surface-structural appearance. Further, a hierarchical graphical model is learned that reflects the constellation of classified parts from the set of specificity levels learned in the previous step. Finally an energy minimization inference procedure is applied on the hierarchical graphical model to obtain the corresponding shape category of an object instance consisting of a set of shape parts. Experiments show, due to the proposed classification by learning the hierarchy of shape parts combined with generating the hierarchical graphical constellation model of shape parts, the additional evidence on different levels of shape detailedness is a major factor that leads to a more robust and accurate categorization compared to a flat approach. The experiments are conducted for shape categories (sack, barrel and parcel) which typically appear in visuoperceptual-challenging scenarios of logistic processes.
更多查看译文
关键词
control engineering computing,graph theory,image classification,image colour analysis,image sensors,inference mechanisms,robot vision,shape recognition,unsupervised learning,Kinect-like sensor,RGB-augmented 3D point clouds,RGBD images,cluttered indoor scenes,energy minimization inference procedure,hierarchical graph constellation models,hierarchical graphical model,object class learning,object shape categorization,part-based shape categorization,shape category,shape detailedness,shape parts classification,shape specificity levels,surface-structural appearance,unsupervised hierarchical learning,unsupervisedly learned shape parts
AI 理解论文
溯源树
样例
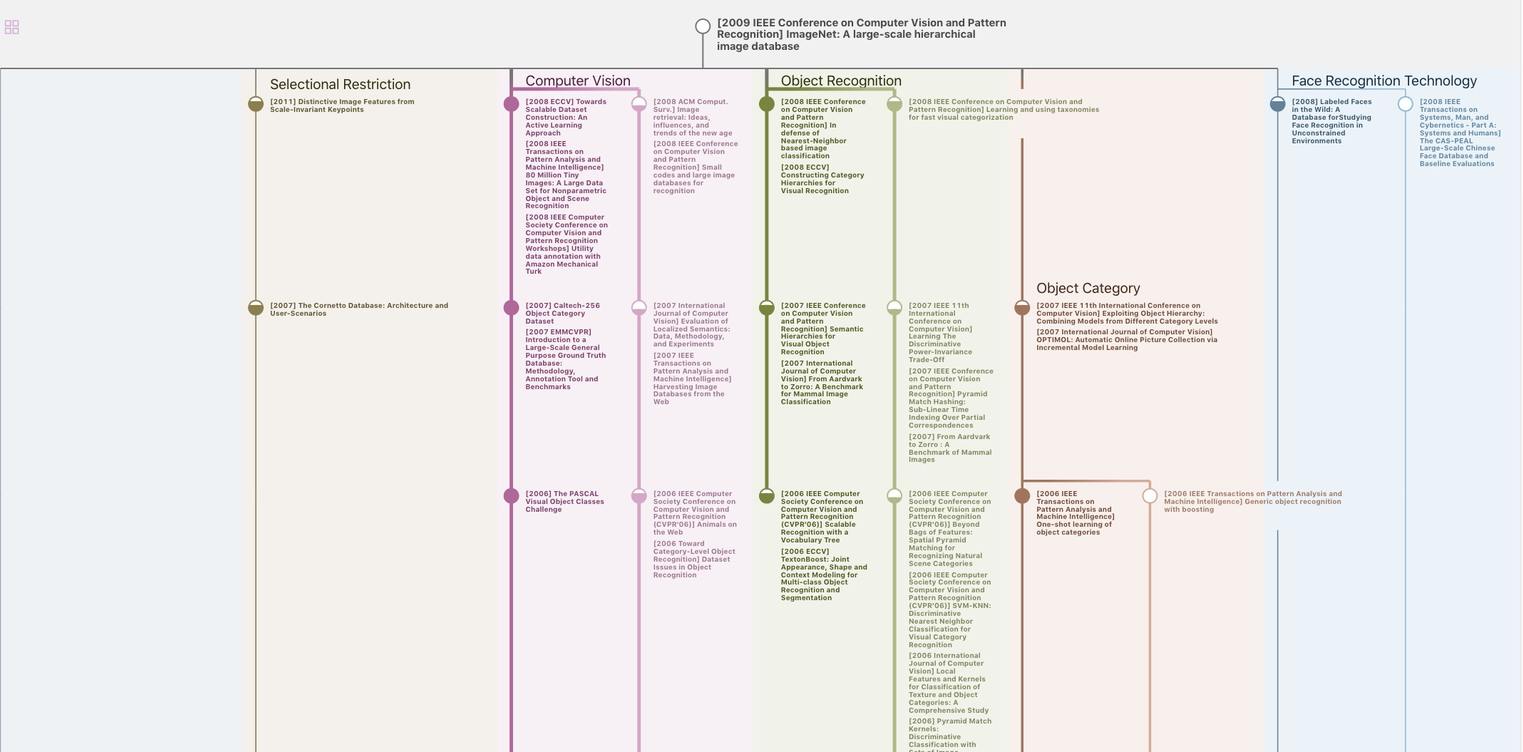
生成溯源树,研究论文发展脉络
Chat Paper
正在生成论文摘要