Self adaptive skipping levels based on the l1-tracker
Signal Processing(2014)
摘要
Object tracking is a critical task which could be used in many applications. The minimum error based l1-tracker is one of the classical tracking algorithms. However, the l1-tracker tracks the target frame-by-frame without considering the continuity of the video. In this paper, a novel improved l1-tracker, Self Adaptive Skipping Levels (SASL), has been proposed. The proposed method takes into consideration the situations in which the matching rate is on tracking by the l1-tracker. If the matching rate is high, it shows that the scene of the video is changing slowly, and then some adjacent frames are skipped for reducing the time consuming. On the other hand, if the matching rate is low, we think the scene of the video changes sharply. In this situation, we have to reduce the skipping level to ensure the matching rate. The experimental results show the excellent performance in comparison with the traditional frame to frame algorithm.
更多查看译文
关键词
object tracking,video surveillance,sasl,matching rate,minimum error based l1-tracker,self adaptive skipping levels,visual surveillance,visual tracking,self adaptive skipping level,smooth of the video,lighting,visualization,prediction algorithms,algorithm design and analysis,accuracy
AI 理解论文
溯源树
样例
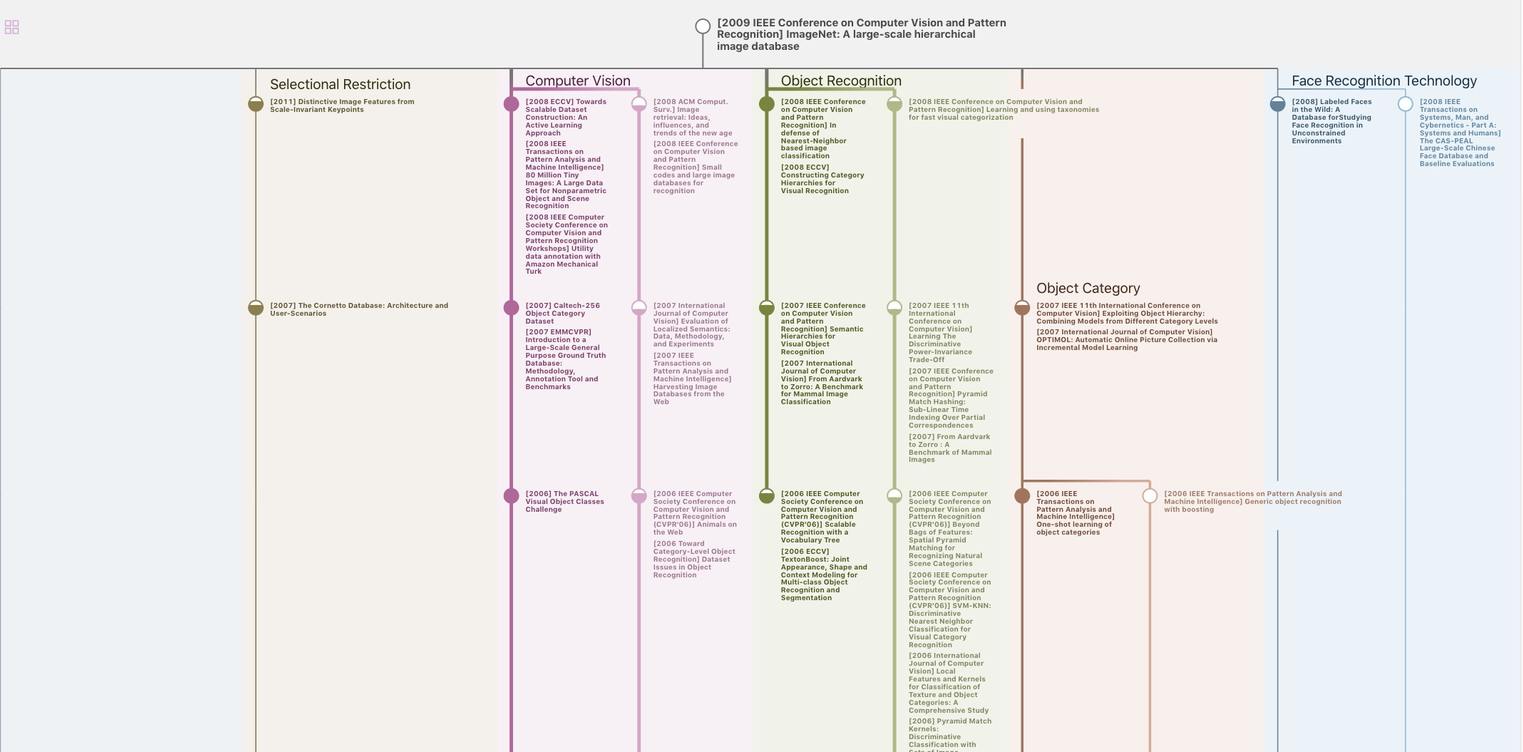
生成溯源树,研究论文发展脉络
Chat Paper
正在生成论文摘要