General Non-Orthogonal Constrained ICA
Signal Processing, IEEE Transactions (2014)
摘要
Constrained independent component analysis (C-ICA) algorithms have been an effective way to introduce prior information into the ICA framework. The work in this area has focus on adding constraints to the objective function of algorithms that assume an orthogonal demixing matrix. Orthogonality is required in order to decouple—isolate—the constraints applied for each individual source. This assumption limits the optimization space and therefore the separation performance of C-ICA algorithms. We generalize the existing C-ICA framework by using a novel decoupling method that preserves the larger optimization space for the demixing matrix. In addition, this framework allows for the constraining of either the sources or the mixing coefficients. A constrained version of the extended Infomax algorithm is used as an example to show the benefits obtained from the non-orthogonal constrained framework we introduce.
更多查看译文
关键词
blind source separation,independent component analysis,matrix algebra,maximum likelihood estimation,C-ICA algorithms,constrained independent component analysis algorithms,extended Infomax algorithm,mixing coefficients,novel decoupling method,optimization space,orthogonal demixing matrix,prior information,Constrained ICA,decoupled,maximum likelihood
AI 理解论文
溯源树
样例
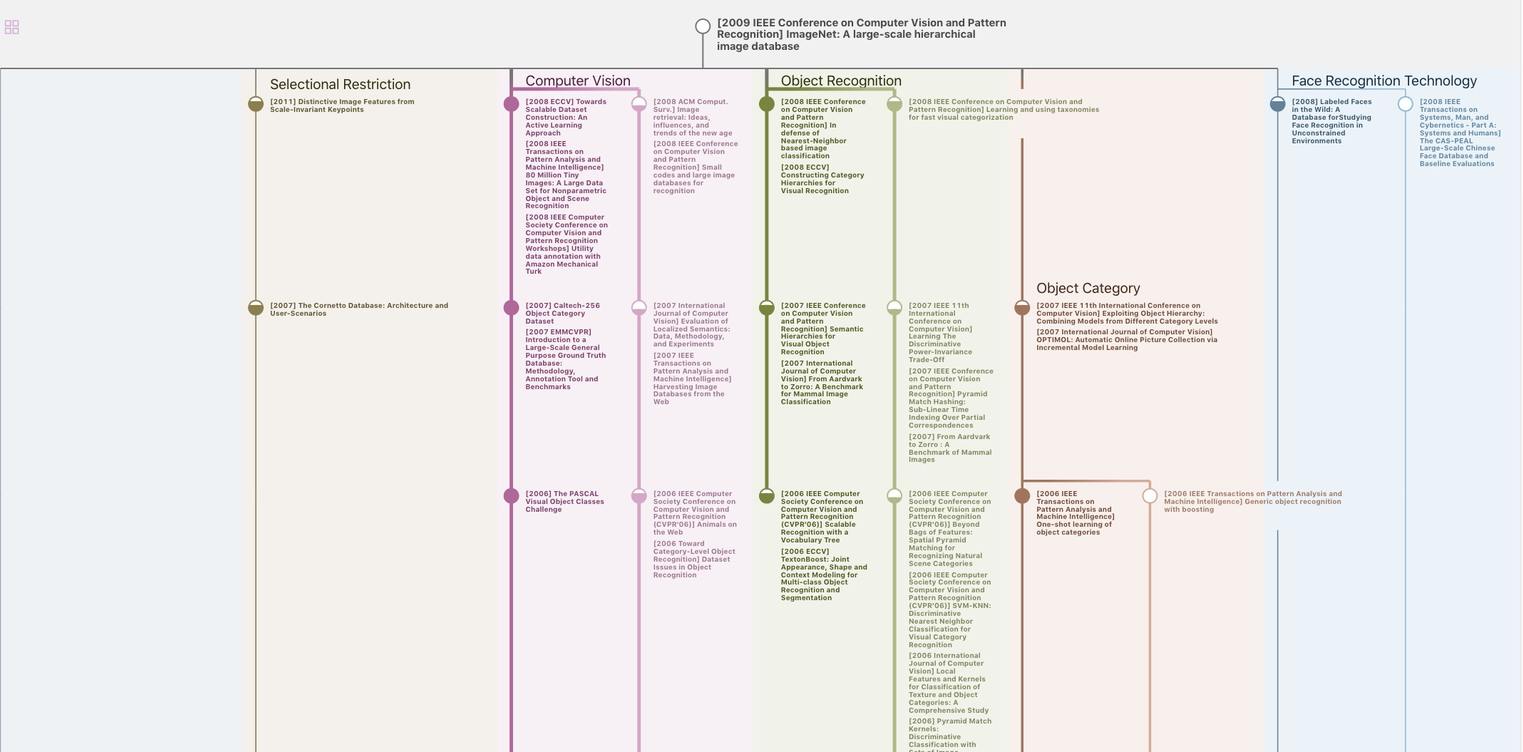
生成溯源树,研究论文发展脉络
Chat Paper
正在生成论文摘要