Video-based traffic data collection system for multiple vehicle types
Intelligent Transport Systems, IET (2014)
摘要
Traffic data of multiple vehicle types are important for pavement design, traffic operations and traffic control. A new video-based traffic data collection system for multiple vehicle types is developed. By tracking and classifying every passing vehicle under mixed traffic conditions, the type and speed of every passing vehicle are recognised. Finally, the flows and mean speeds of multiple vehicle types are output. A colour image-based adaptive background subtraction is proposed to obtain more accurate vehicle objects, and a series of processes like shadow removal and setting road detection region are used to improve the system robustness. In order to improve the accuracy of vehicle counting, the cross-lane vehicles are detected and repeated counting for one vehicle is avoided. In order to reduce the classification errors, the space ratio of the blob and data fusion are used to reduce the classification errors caused by vehicle occlusions. This system was tested under four different weather conditions. The accuracy of vehicle counting was 97.4% and the error of vehicle classification was 8.3%. The correlation coefficient of speeds detected by this system and radar gun was 0.898 and the mean absolute error of speed detection by this system was only 2.3 km/h.
更多查看译文
关键词
image classification,image colour analysis,image fusion,object tracking,road traffic,traffic engineering computing,video signal processing,blob space ratio,classification error reduction,colour image-based adaptive background subtraction,cross-lane vehicle,data fusion,mean absolute error,mixed traffic condition,passing vehicle classification,passing vehicle speed,passing vehicle tracking,passing vehicle type,pavement design,road detection setting,shadow removal process,speed detection,traffic control,traffic operation,vehicle counting,vehicle occlusion,vehicle type,video-based traffic data collection system,accuracy
AI 理解论文
溯源树
样例
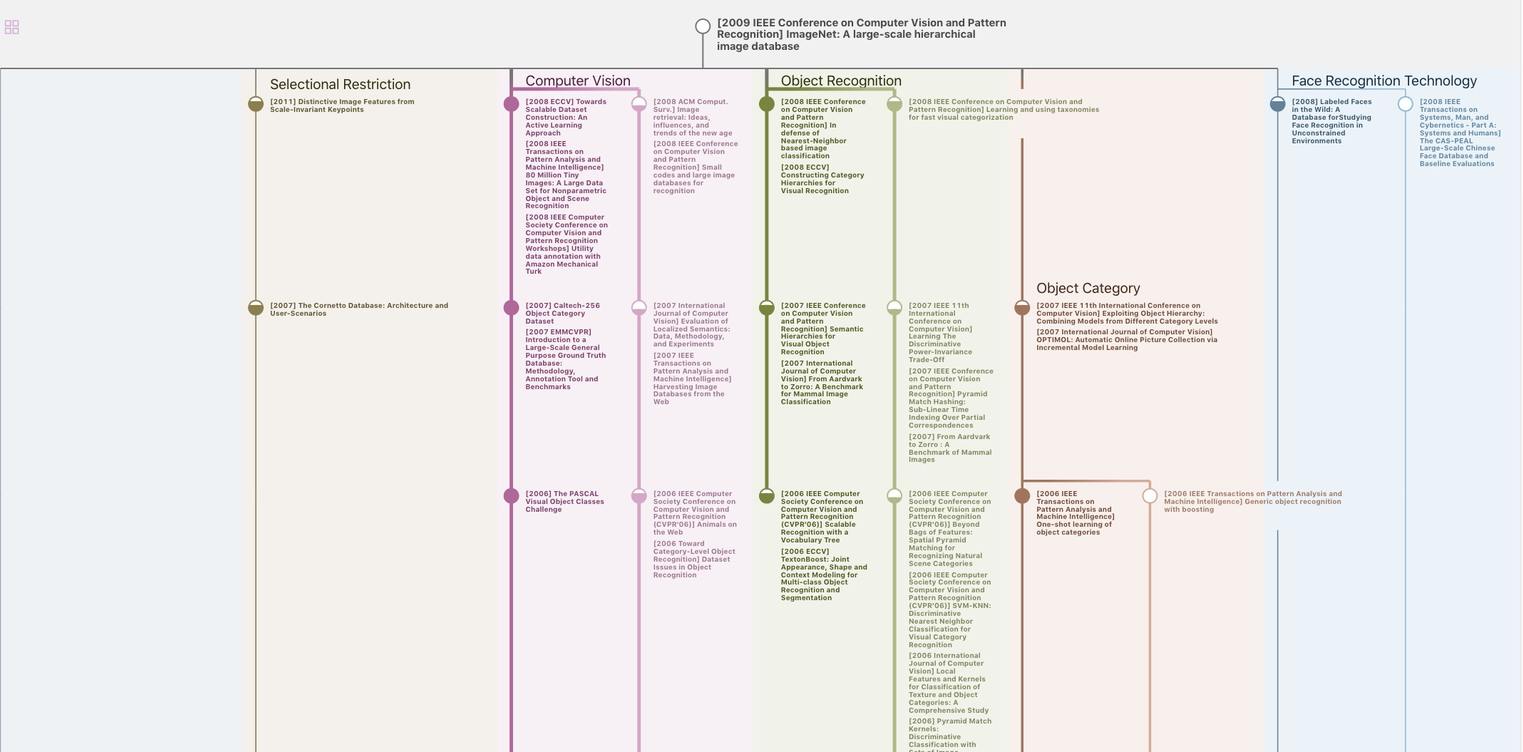
生成溯源树,研究论文发展脉络
Chat Paper
正在生成论文摘要