TRISTAN: Real-time analytics on massive time series using sparse dictionary compression
BigData Conference(2014)
摘要
Large-scale critical infrastructures such as transportation, energy, or water distribution networks are increasingly equipped with smart sensor technologies. Low-latency analytics on the resulting times series would open the door to many exciting opportunities to improve our grasp on complex urban systems. However, sensor-generated time series often turn out to be noisy, non-uniformly sampled, and misaligned in practice, making them ill-suited for traditional data processing. In this paper, we introduce TRISTAN (massive TRIckletS Time series ANalysis), a new data management system for efficient storage and real-time processing of fine-grained time series data. TRISTAN relies on a dedicated, compressed sparse representation of the time series using a dictionary. In contrast to previous approaches, TRISTAN is able to execute most analytics queries on the compressed data directly, and supports efficient and approximate query answering based on the most significant atoms of the dictionary only. We present the overall architecture of our system and discuss its performance on several smarter city datasets, showing that TRISTAN can achieve up to 20:1 compression ratios and 250x speedup compared to a state-of-the-art system.
更多查看译文
关键词
Big Data,intelligent sensors,real-time systems,storage management,time series,TRISTAN,data management system,data processing,data storage,fine-grained time series data,large-scale critical infrastructures,low-latency analytics,massive tricklets time series analysis,real-time analytics,real-time processing,smart sensor technologies,sparse dictionary compression,dictionary compression,matching pursuit,tricklets
AI 理解论文
溯源树
样例
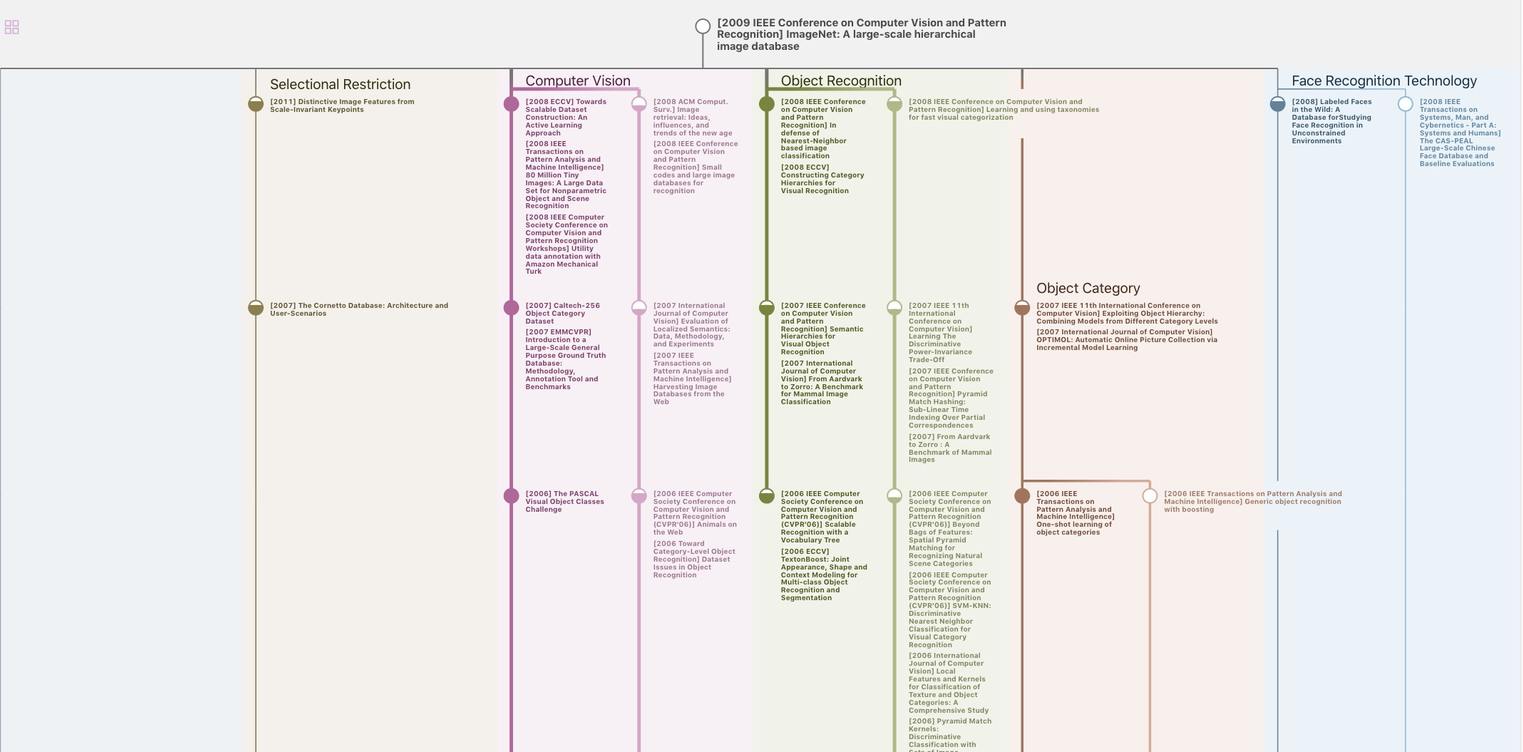
生成溯源树,研究论文发展脉络
Chat Paper
正在生成论文摘要