A Knee Point Driven Evolutionary Algorithm for Many-Objective Optimization
Evolutionary Computation, IEEE Transactions (2015)
摘要
Evolutionary algorithms have shown to be promising in solving many-objective optimization problems, where the performance of these algorithms heavily depends on whether solutions that can accelerate convergence towards the Pareto front and maintain a high degree of diversity will be selected from a set of non-dominated solutions. In this work, we propose a knee point driven evolutionary algorithm to solve many-objective optimization problems. Our basic idea is that knee points are naturally most preferred among non-dominated solutions if no explicit user preferences are given. A bias towards the knee points in the nondominated solutions in the current population is shown to be an approximation of a bias towards a large hypervolume, thereby enhancing the convergence performance in manyobjective optimization. In addition, as at most one solution will be identified as a knee point inside the neighborhood of each solution in the non-dominated front, no additional diversity maintenance mechanisms need to be introduced in the proposed algorithm, considerably reducing the computational complexity compared to many existing multi-objective evolutionary algorithms for many-objective optimization. Experimental results on 16 test problems demonstrate the competitiveness of the proposed algorithm in terms of both solution quality and computational efficiency.
更多查看译文
关键词
evolutionary multi-objective optimization,convergence,diversity,hypervolume,knee point,many-objective optimization,sociology,evolutionary computation,measurement,pareto optimization
AI 理解论文
溯源树
样例
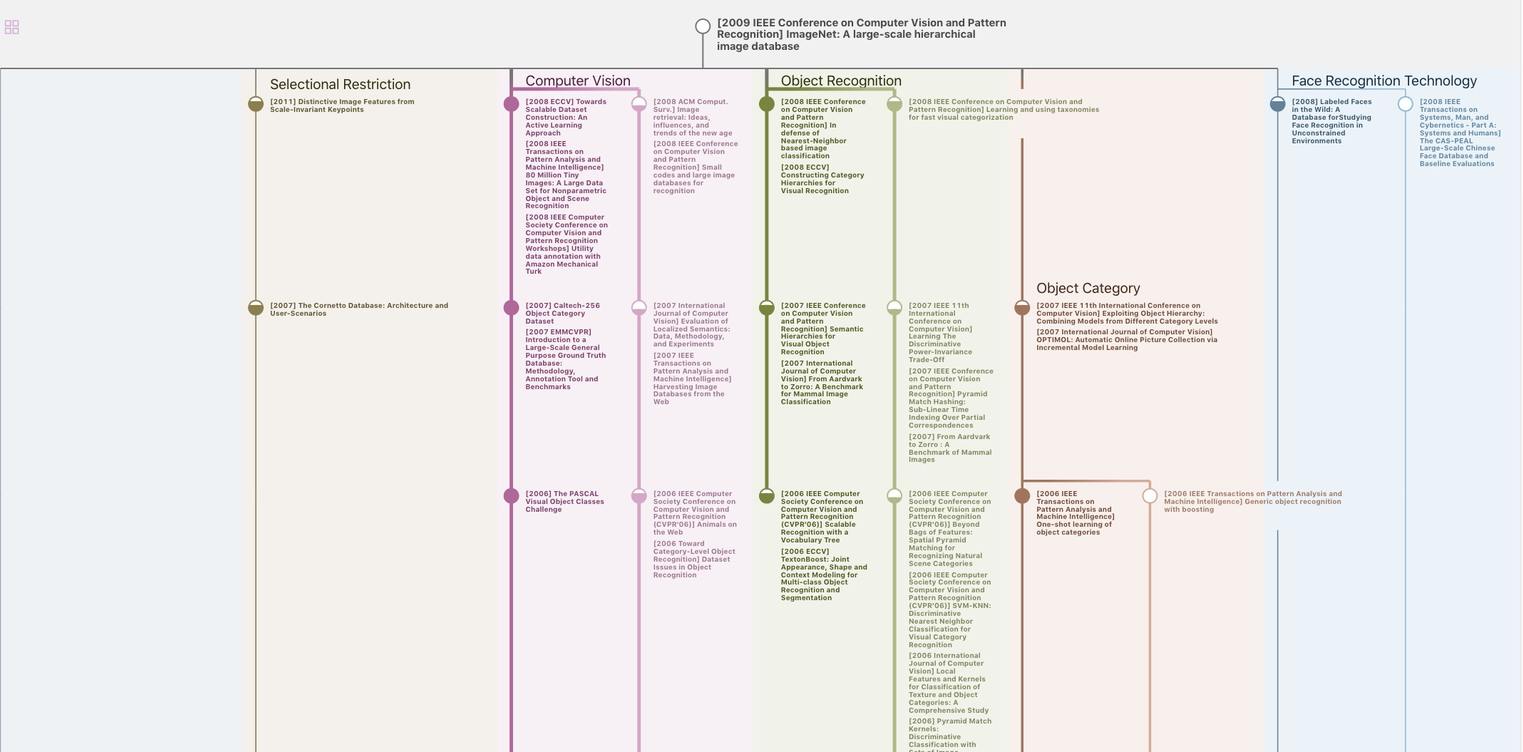
生成溯源树,研究论文发展脉络
Chat Paper
正在生成论文摘要