Manifold Adaptive Label Propagation for Face Clustering.
IEEE transactions on cybernetics(2015)
摘要
In this paper, a novel label propagation (LP) method is presented, called the manifold adaptive label propagation (MALP) method, which is to extend original LP by integrating sparse representation constraint into regularization framework of LP method. Similar to most LP, first of all, MALP also finds graph edges from given data and gives weights to the graph edges. Our goal is to find graph weights matrix adaptively. The key advantage of our approach is that MALP simultaneously finds graph weights matrix and predicts the label of unlabeled data. This paper also derives efficient algorithm to solve the proposed problem. Extensions of our MALP in kernel space and robust version are presented. The proposed method has been applied to the problem of semi-supervised face clustering using the well-known ORL, Yale, extended YaleB, and PIE datasets. Our experimental evaluations show the effectiveness of our method.
更多查看译文
关键词
clustering,label propagation (lp),semi-supervised learning,sparse matrices,clustering algorithms,vectors,optimization,face,linear programming
AI 理解论文
溯源树
样例
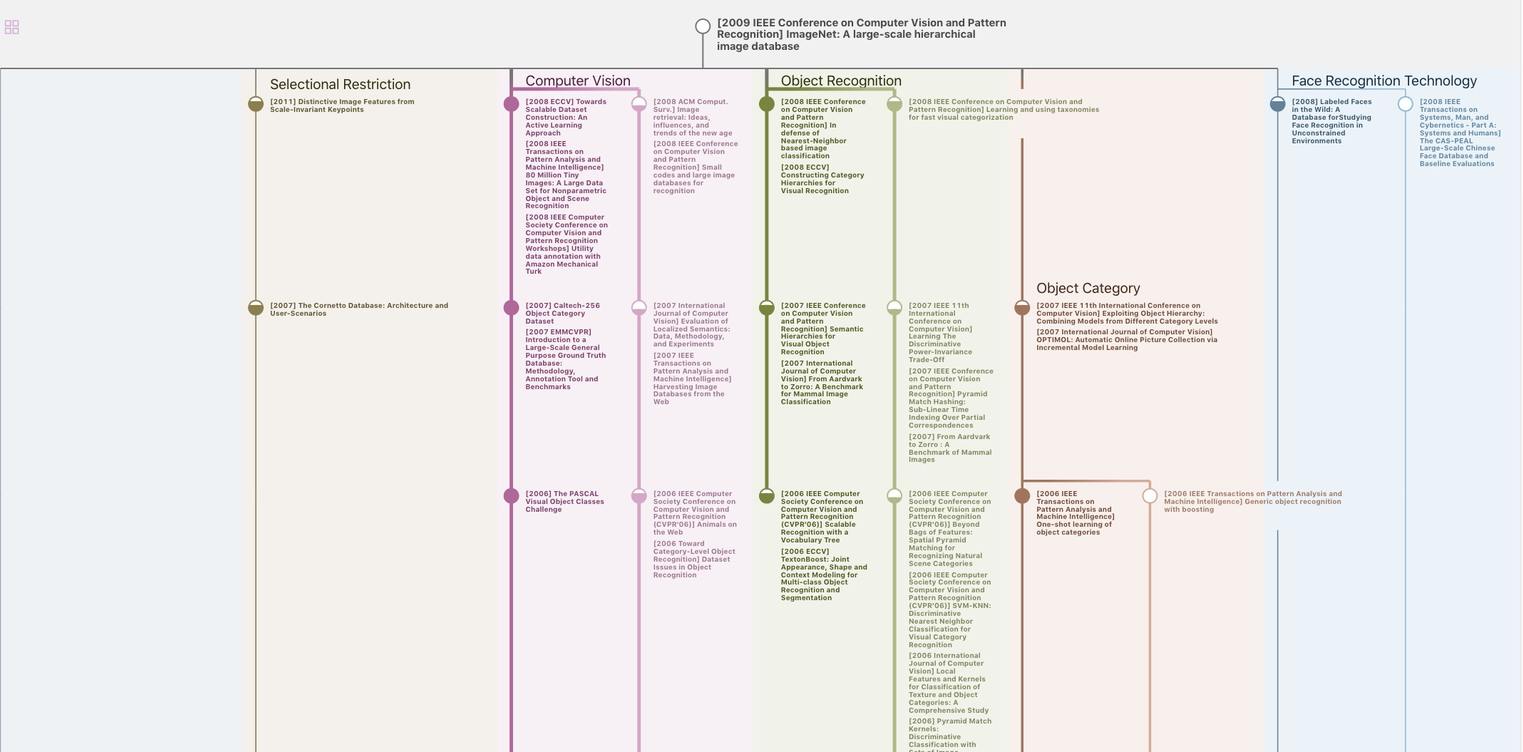
生成溯源树,研究论文发展脉络
Chat Paper
正在生成论文摘要