Non-parametric Bayesian learning with deep learning structure and its applications in wireless networks
Signal and Information Processing(2014)
摘要
In this paper, we present an infinite hierarchical non-parametric Bayesian model to extract the hidden factors over observed data, where the number of hidden factors for each layer is unknown and can be potentially infinite. Moreover, the number of layers can also be infinite. We construct the model structure that allows continuous values for the hidden factors and weights, which makes the model suitable for various applications. We use the Metropolis-Hastings method to infer the model structure. Then the performance of the algorithm is evaluated by the experiments. Simulation results show that the model fits the underlying structure of simulated data.
更多查看译文
关键词
belief networks,learning (artificial intelligence),radio networks,telecommunication computing,deep learning structure,infinite hierarchical nonparametric Bayesian model,metropolis-hastings method,nonparametric Bayesian learning,performance evaluation,wireless networks,Indian Buffet Process,Metropolis-Hastings algorithm,deep learning,non-parametric Bayesian learning
AI 理解论文
溯源树
样例
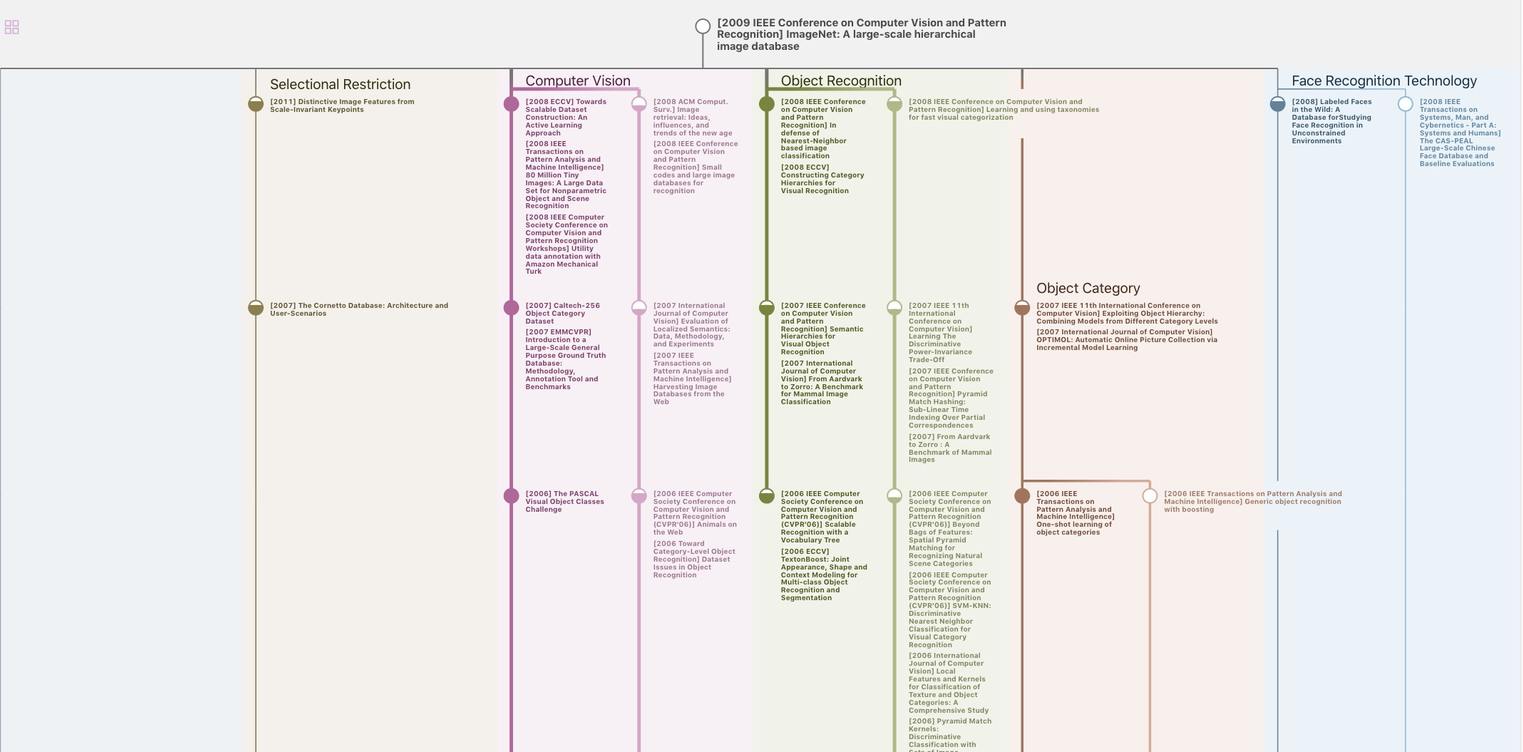
生成溯源树,研究论文发展脉络
Chat Paper
正在生成论文摘要