Low-rank tensor decomposition based dynamic network tracking
Signal and Information Processing(2014)
摘要
Lots of data is generated around us in today's big data age. Much of this data is time-varying or dynamic, such as the social network connections that change across time or dynamic functional brain connectivity networks constructed across multiple subjects. In these dynamic (time-varying) networks, it is important to reduce the large amount of data into a few meaningful descriptors. One way to achieve this goal is to detect change points or anomalies in the connectivity patterns across time. Recently, there has been an interest in describing the time-varying network activity as a tensor and detecting the anomalies in terms of the changes in the subspaces of the tensor along each mode [1], [2]. However, the current approaches to tensor decomposition are not robust to non-Gaussian noise, outliers, and corruption in the data. For this reason, a robust low-rank tensor recovery algorithm similar to robust principal components analysis (RPCA) has been recently proposed. In this paper, we employ higher order robust PCA (HoRPCA) for tracking dynamic networks in time and detecting anomalies using a subspace distance measure. The proposed approach assumes that most real life networks are low-rank in nature and considers a low-rank plus sparse tensor decomposition at each time point. The subspaces corresponding to each mode and each time point are described through a projection operator and the subspace distance is quantified through a Hausdorff distance measure. The proposed framework is evaluated on both simulated networks and dynamic functional connectivity brain networks.
更多查看译文
关键词
Big Data,principal component analysis,Hausdorff distance measure,HoRPCA,big data age,connectivity patterns,dynamic functional brain connectivity networks,dynamic network tracking,higher order robust PCA,low rank plus sparse tensor decomposition,low rank tensor decomposition,nonGaussian noise,outliers,robust low rank tensor recovery algorithm,robust principal components analysis,simulated networks,social network connections,subspace distance measure,time-varying network activity,dynamic networks,functional connectivity,robust PCA,tensor decomposition
AI 理解论文
溯源树
样例
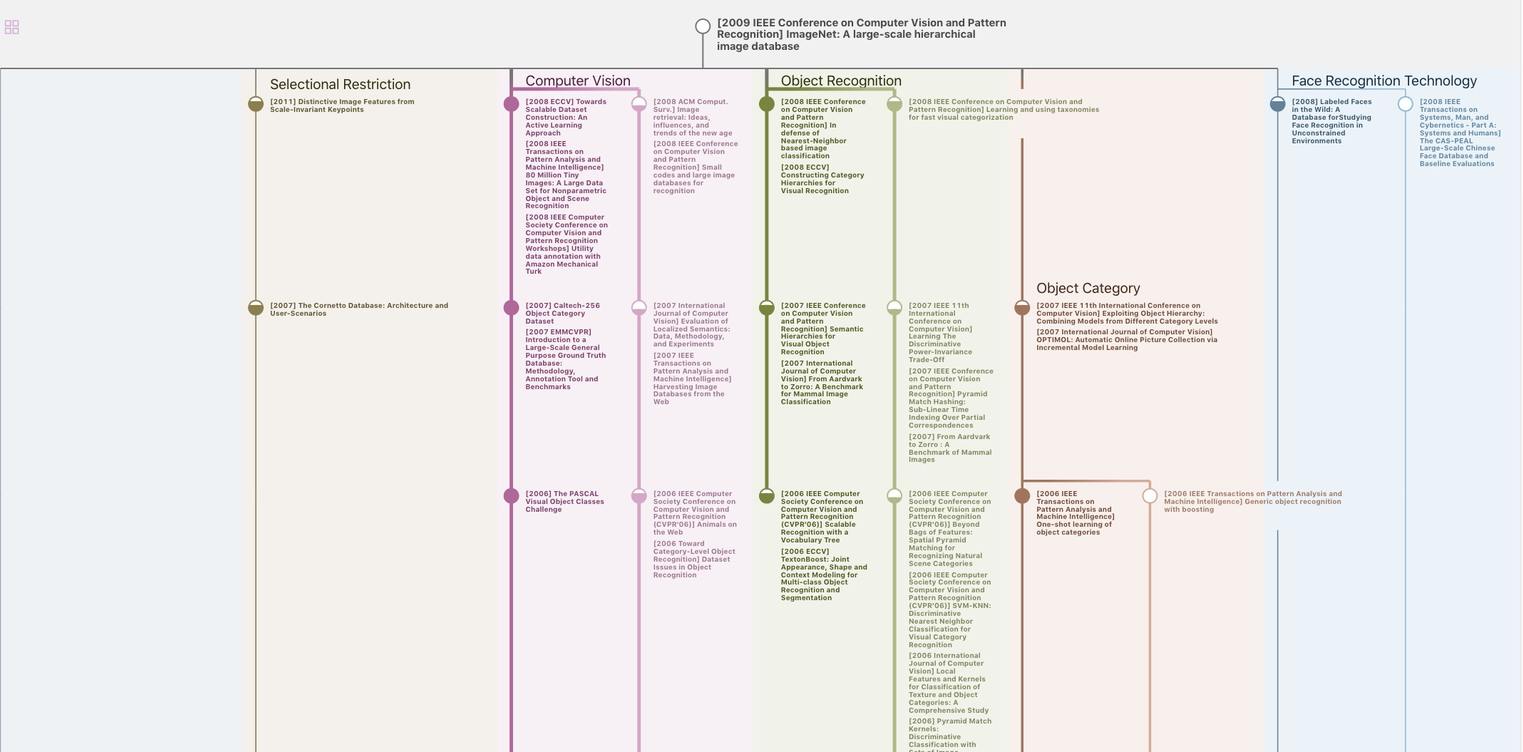
生成溯源树,研究论文发展脉络
Chat Paper
正在生成论文摘要