Privacy-preserving function computation by exploitation of friendships in social networks
Acoustics, Speech and Signal Processing(2014)
摘要
We study the problem of privacy-preserving computation of functions of data that belong to users in a social network under the assumption that users are willing to share their private data with trusted friends in the network. We demonstrate that such trust relationships can be exploited to significantly improve the tradeoff between the privacy of users' data and the accuracy of the computation. Under a one-hop trust model we design an algorithm for partitioning the users into circles of trust and develop a differentially private scheme for computing the global function using results of local computations within each circle. We quantify the improvement in the privacy-accuracy tradeoff of our scheme with respect to other mechanisms that do not exploit inter-user trust. We verify the efficiency of our algorithm by implementing it on social networks with up to one million nodes. Applications of our method include surveys, elections, and recommendation systems.
更多查看译文
关键词
data privacy,recommender systems,social networking (online),trusted computing,differentially private scheme,friendships exploitation,interuser trust,one-hop trust model,privacy-accuracy tradeoff,privacy-preserving function computation,private data sharing,recommendation systems,social networks,trust relationships,trusted friends
AI 理解论文
溯源树
样例
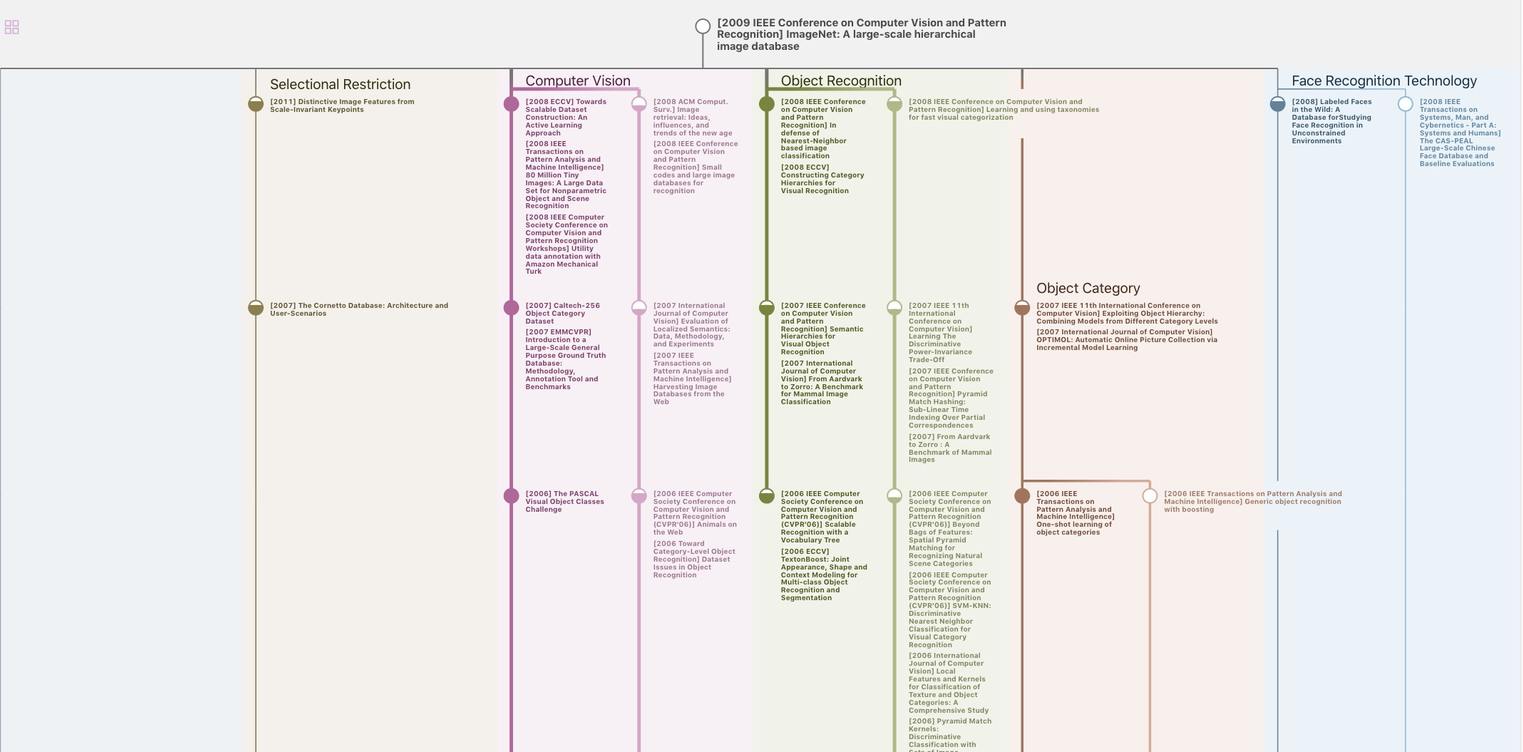
生成溯源树,研究论文发展脉络
Chat Paper
正在生成论文摘要