Effectiveness of PLP-based phonetic segmentation for speech synthesis
Acoustics, Speech and Signal Processing(2014)
摘要
In this paper, use of Viterbi-based algorithm and spectral transition measure (STM)-based algorithm for the task of speech data labeling is being attempted. In the STM framework, we propose use of several spectral features such as recently proposed cochlear filter cepstral coefficients (CFCC), perceptual linear prediction cepstral coefficients (PLPCC) and RelAtive SpecTrAl (RASTA)-based PLPCC in addition to Mel frequency cepstral coefficients (MFCC) for phonetic segmentation task. To evaluate effectiveness of these segmentation algorithms, we require manual accurate phoneme-level labeled data which is not available for low resourced languages such as Gujarati (one of the official languages of India). In order to measure effectiveness of various segmentation algorithms, HMM-based speech synthesis system (HTS) for Gujarati has been built. From the subjective and objective evaluations, it is observed that Viterbi-based and STM with PLPCC-based segmentation algorithms work better than other algorithms.
更多查看译文
关键词
cepstral analysis,hidden Markov models,maximum likelihood estimation,speech synthesis,CFCC,Gujarati,HMM-based speech synthesis system,HTS,MFCC,RASTA-based PLPCC,STM-based algorithm,Viterbi-based algorithm,cochlear filter cepstral coefficients,mel frequency cepstral coefficients,perceptual linear prediction cepstral coefficients,phonetic segmentation task,relative spectral-based PLPCC,spectral transition measure-based algorithm,speech data labeling,Hidden Markov Model (HMM),PLPCC,Spectral Transition Measure (STM)
AI 理解论文
溯源树
样例
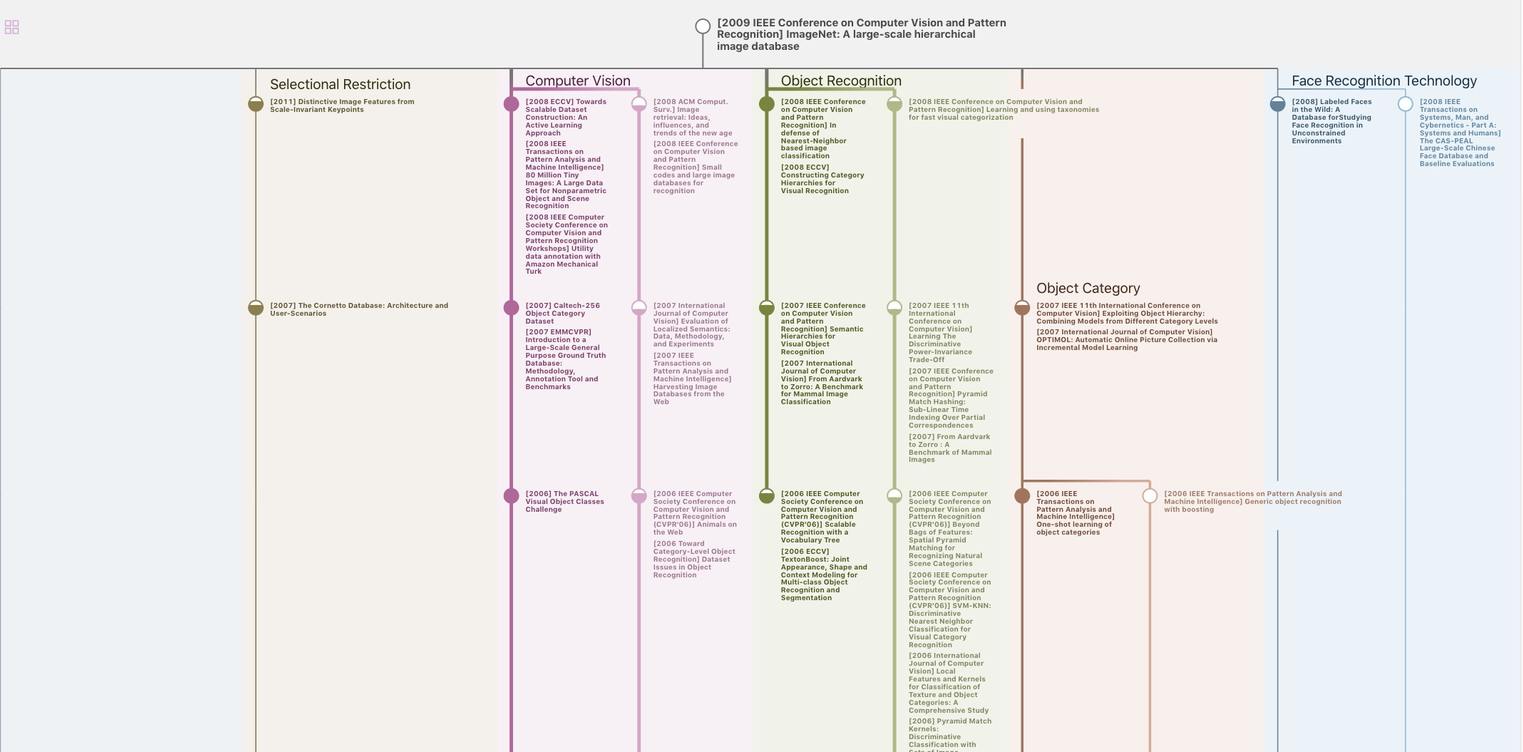
生成溯源树,研究论文发展脉络
Chat Paper
正在生成论文摘要