Compressing VG-RAM WNN memory for lightweight applications
Neural Networks(2014)
摘要
The Virtual Generalizing Random Access Memory Weightless Neural Network (VG-RAM WNN) is an effective machine learning technique that offers simple implementation and fast training. One disadvantage of VG-RAM WNN, however, is the test time for applications with many training samples, i.e. large multi-class classification applications. In such cases, the test time tends to be high, since it increases with the size of the memory of each neuron. In this paper, we present a new methodology for handling such applications using VG-RAM WNN. By employing data clustering techniques to reduce the overall size of the neurons' memory, we were able to reduce the network's memory footprint and the system's runtime, while maintaining a high and acceptable classification performance. We evaluated the performance of our VG-RAM WNN system with compressed memory on the problem of traffic sign recognition. Our experimental results showed that, after compression, the system was able to run at very fast response times in standard computers. Also, we were able to load and run the system at interactive rates in small low-power systems, experiencing only a small reduction in classification performance.
更多查看译文
关键词
image recognition,learning (artificial intelligence),neural nets,random-access storage,VG-RAM WNN memory system,compressed memory,data clustering techniques,machine learning technique,traffic sign recognition,virtual generalizing random access memory weightless neural network,Clustering Techniques,Traffic Sign Recognition,Virtual Generalizing Random Access Memory Weightless Neural Networks
AI 理解论文
溯源树
样例
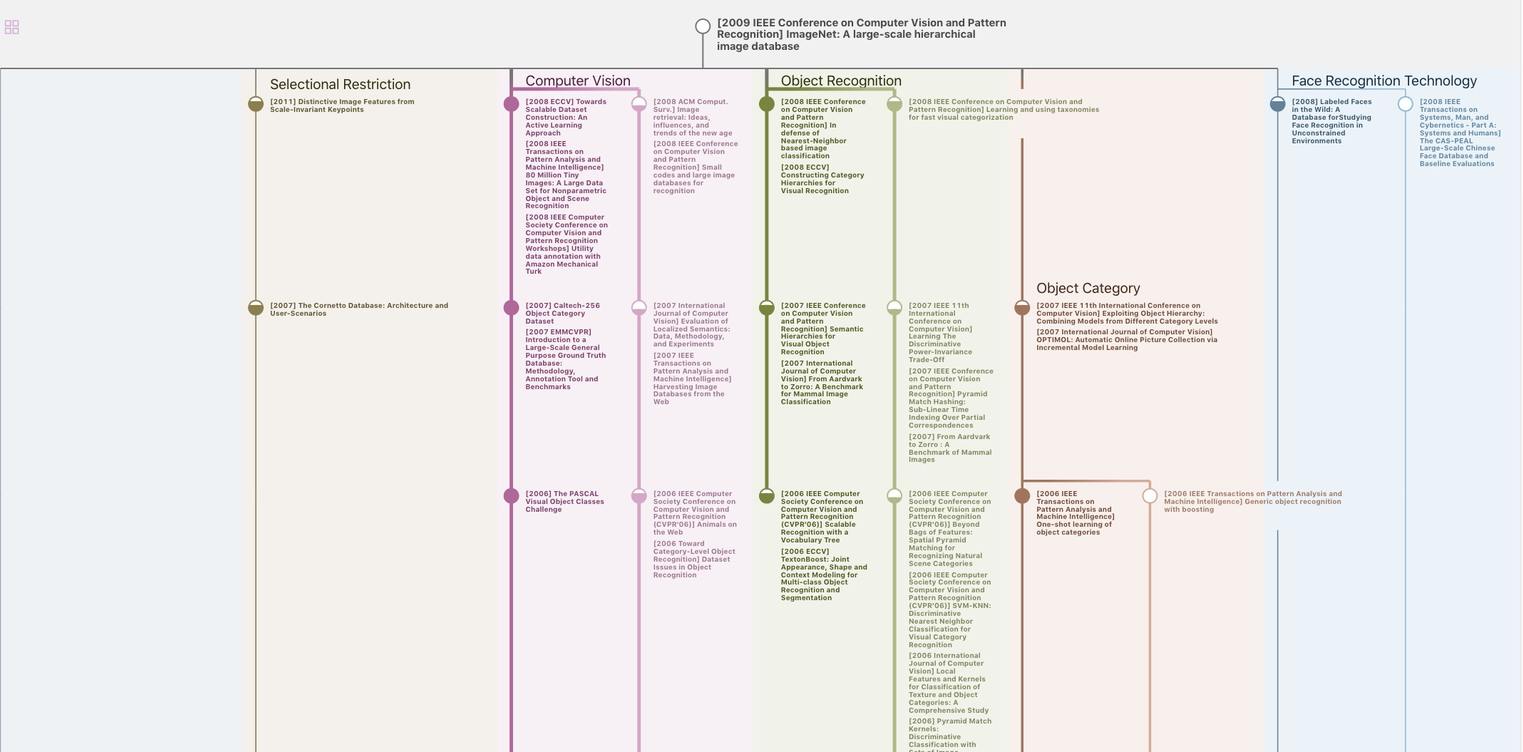
生成溯源树,研究论文发展脉络
Chat Paper
正在生成论文摘要