Estimating Dynamics On-the-Fly Using Monocular Video For Vision-Based Robotics
Mechatronics, IEEE/ASME Transactions (2014)
摘要
Estimating the physical parameters of articulated multibody systems (AMBSs) using an uncalibrated monocular camera poses significant challenges for vision-based robotics. Articulated multibody models, especially ones including dynamics, have shown good performance for pose tracking, but require good estimates of system parameters. In this paper, we first propose a technique for estimating parameters of a dynamically equivalent model (kinematic/geometric lengths as well as mass, inertia, damping coefficients) given only the underlying articulated model topology. The estimated dynamically equivalent model is then employed to help predict/filter/gap-fill the raw pose estimates, using an unscented Kalman filter. The framework is tested initially on videos of a relatively simple AMBS (double pendulum in a structured laboratory environment). The double pendulum not only served as a surrogate model for the human lower limb in flight phase, but also helped evaluate the role of model fidelity. The treatment is then extended to realize physically plausible pose-estimates of human lower-limb motions, in more-complex uncalibrated monocular videos (from the publicly available DARPA Mind's Eye Year 1 corpus). Beyond the immediate problem-at-hand, the presented work has applications in creation of low-order surrogate computational dynamics models for analysis, control, and tracking of many other articulated multibody robotic systems (e.g., manipulators, humanoids) using vision.
更多查看译文
关键词
kalman filters,computer vision,nonlinear filters,pose estimation,robot dynamics,topology,ambss,articulated model topology,articulated multibody robotic systems,computational dynamics models,double pendulum,dynamics on-the-fly estimation,human lower-limb motions,monocular video,pose tracking,unscented kalman filter,vision-based robotics,articulated multibody dynamics,estimation,system identification
AI 理解论文
溯源树
样例
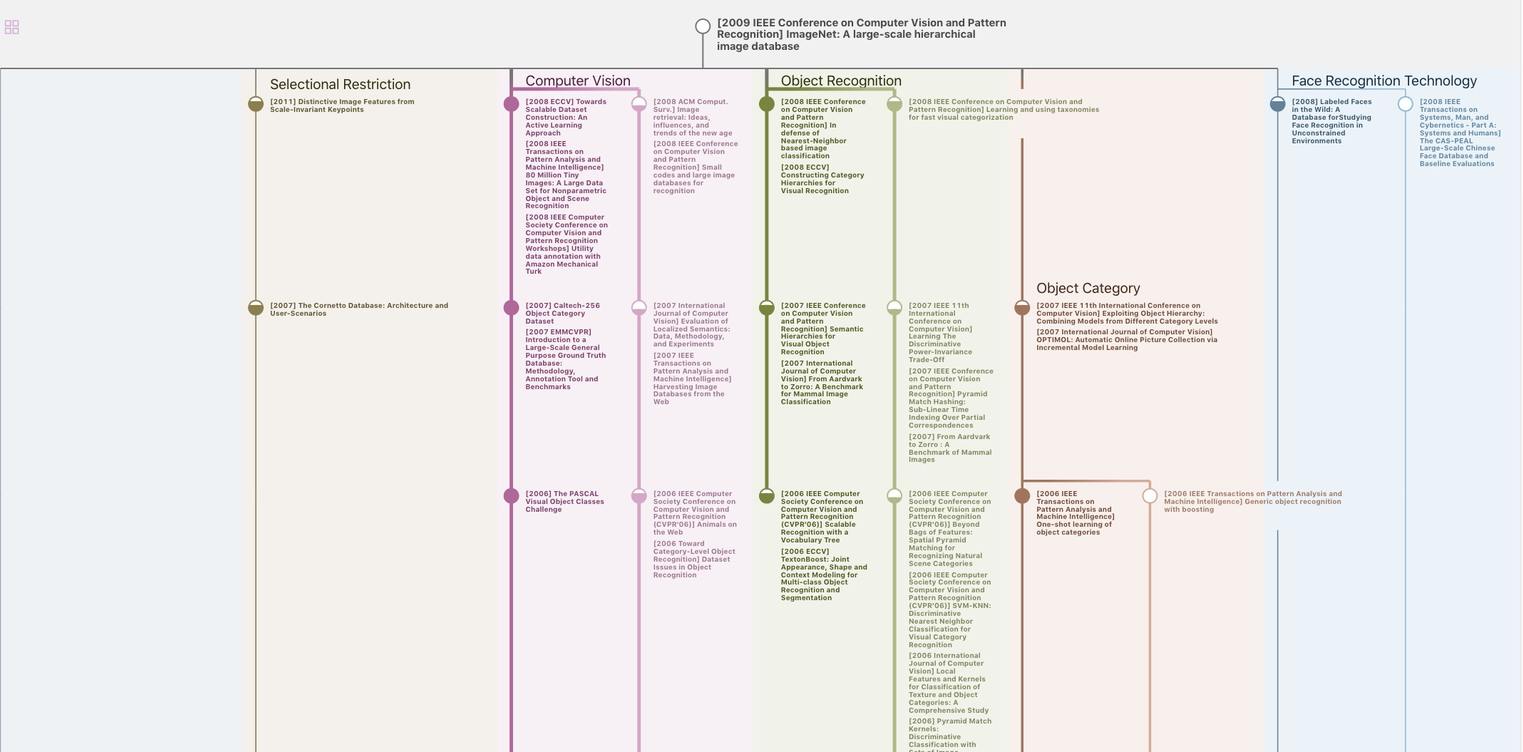
生成溯源树,研究论文发展脉络
Chat Paper
正在生成论文摘要