Underwater magnetic target localization and characterization using a three-axis gradiometer
Taipei(2014)
摘要
Magnetometers and magnetic gradiometers are commonly used to detect ferromagnetic targets. When the distance to the target is large compared to its size, the target can be modeled as a dipolar source and can then be characterized by a single vector: its magnetic moment. The estimation of the magnetic moment of the target can be used to reduce the false alarm rate. We consider a total-field three-axis gradiometer which measures the gradient of the magnitude of the magnetic field along three orthogonal axes. We show that for an ideal gradiometer, the inversion problem separates into two linear problems and can therefore be solved without any initial estimation of the parameters. Moreover, the method can be applied directly on the data gathered by the gradiometer, without any grid interpolation and in real time. For the considered gradiometer, a better estimation of the parameters can be obtained if the detailed geometry of the gradiometer (location of the magnetometers) is taken into account. We show that the method can be extended to magnetometers, vertical total-field gradiometers, horizontal total-field gradiometers and tensor gradiometers. Finally, we analyze the effect of various survey parameters (gradiometer altitude, inter-track distance and target magnetic moment) on the accuracy of the estimated parameters in the presence of noise (magnetic noise and error on the position of the gradiometer).
更多查看译文
关键词
gradient methods,interpolation,magnetic fields,magnetic moments,magnetic noise,magnetometers,object detection,parameter estimation,tensors,data gathering,false alarm rate reduction,ferromagnetic target detection,grid interpolation,inversion problem,linear problem,magnetic field magnitude gradient measurement,magnetic moment estimation,magnetometer noise,orthogonal axes,three-axis magnetic gradiometer geometry,underwater magnetic target characterization,underwater magnetic target localization
AI 理解论文
溯源树
样例
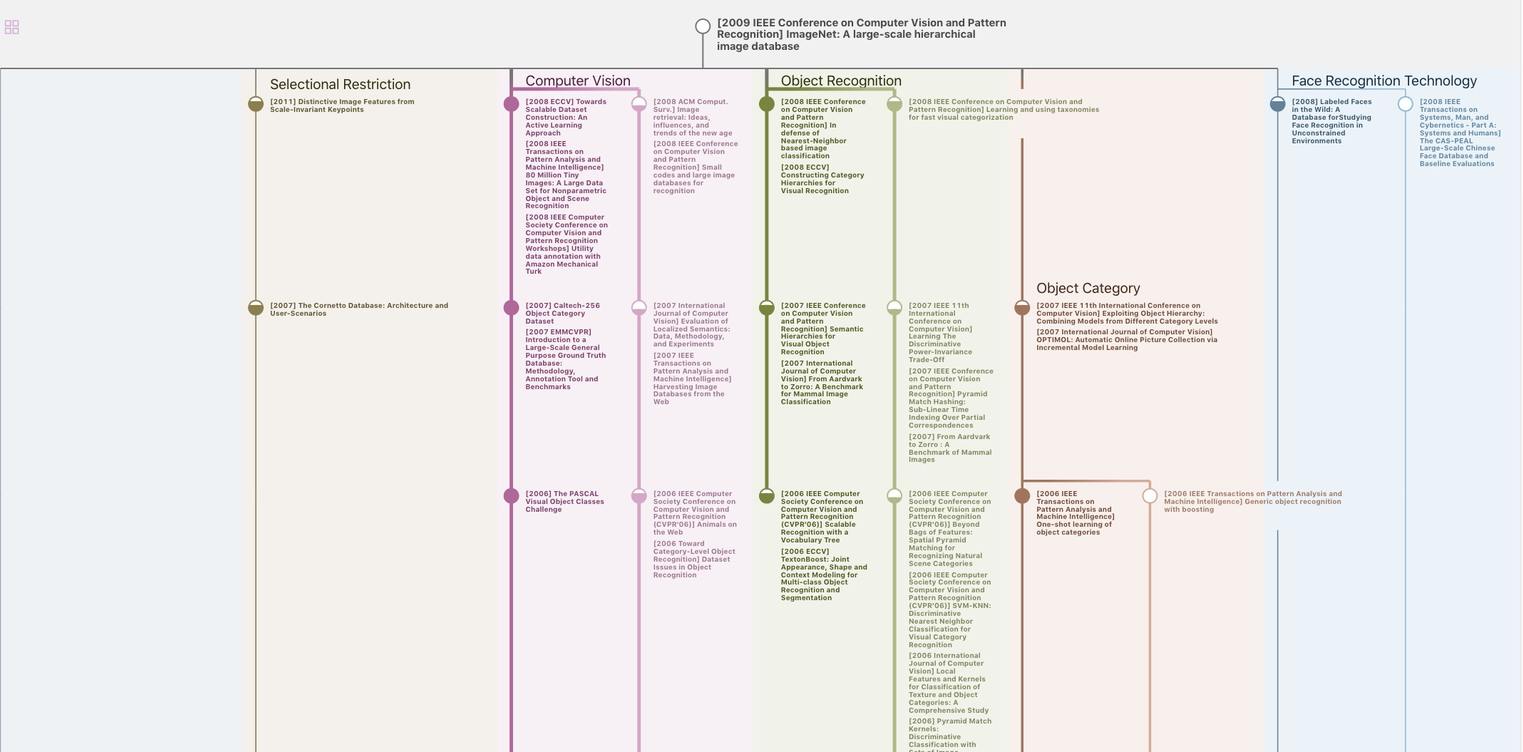
生成溯源树,研究论文发展脉络
Chat Paper
正在生成论文摘要