Learning of usage of tools based on interaction between humans and robots
Cyber Technology in Automation, Control, and Intelligent Systems(2014)
摘要
To perform a tool-using task by manipulating some target object, a robot needs to a) determine the desired effects of the task b) determine a suitable tool c) generate the correct position and orientation in which tool should be placed relative to the target object and d) determine the action to subsequently manipulate the tool after it is placed. Thus, learning to use tool requires robot to determine casual dependencies that exist among the spatial constraints of the environment, desired effects, functional features of the tool, actions and structural constraints of the tool. But learning of these casual dependencies by performing tool manipulation tasks using self-exploration or pre-programming by user is computationally expensive and resource intensive process. Thus, to address such a problem, learning of tool use in an online, incremental and interactive manner is proposed, where robots learn tool-use using trial-and-error-and-interaction loop. To deal with uncertainties in the domain knowledge, robot learning and inference process, probabilistic semantics of Bayesian network(BN) is used. BN allows making probabilistic queries to human user based on internal state of the robot and also allows incorporating user feedback with a degree of confidence. The concept of interaction based learning and experimental scenarios are presented.
更多查看译文
关键词
belief networks,control engineering computing,human-robot interaction,inference mechanisms,probability,task analysis,Bayesian network,domain knowledge,human-robot interaction,inference process,probabilistic queries,probabilistic semantics,robot learning,structural constraints,target object,tool manipulation tasks
AI 理解论文
溯源树
样例
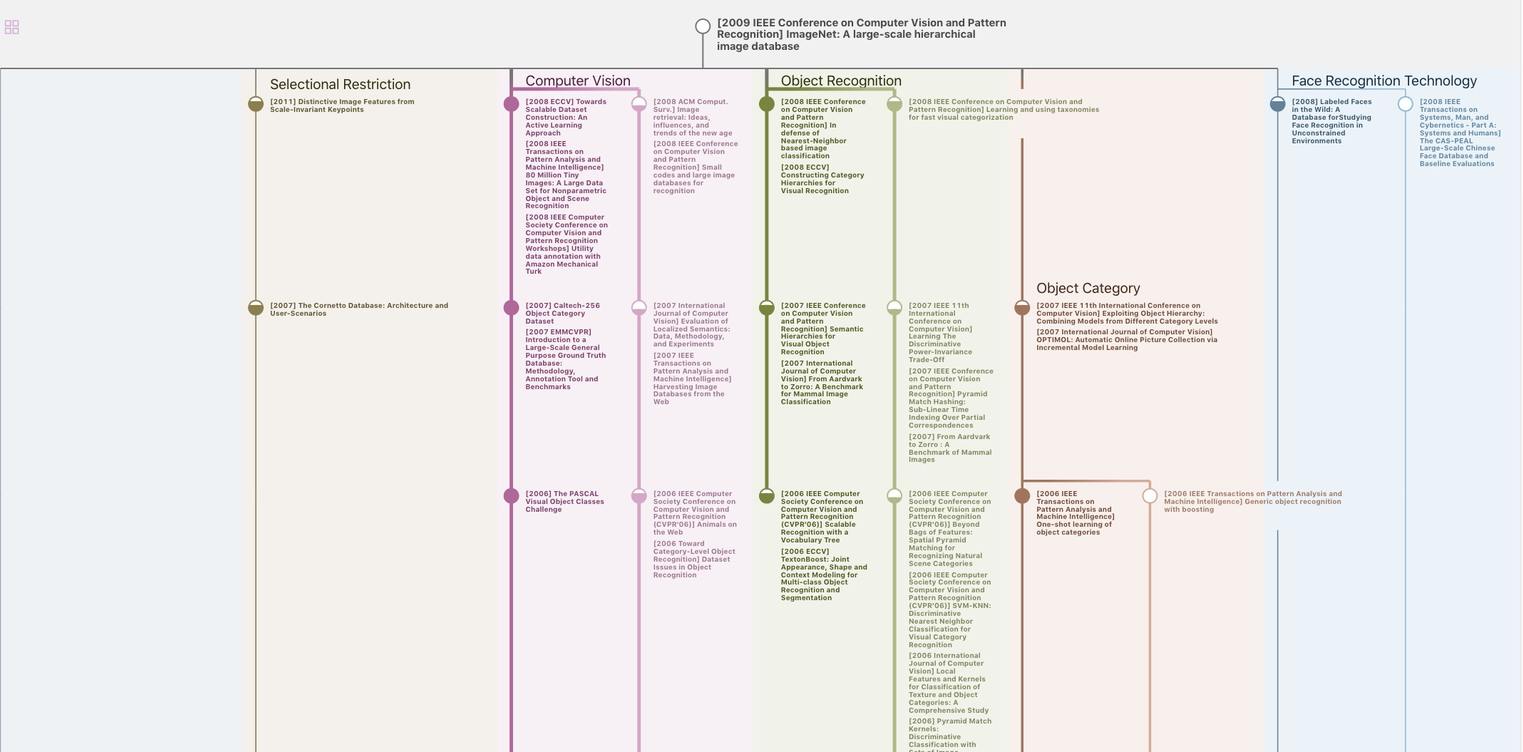
生成溯源树,研究论文发展脉络
Chat Paper
正在生成论文摘要