Direct sub-word confidence estimation with hidden-state conditional random fields
Acoustics, Speech and Signal Processing(2014)
摘要
The estimation of accurate confidence scores for sub-word-level units within automatic speech recognition (ASR) system transcriptions is investigated in this work. This is achieved through the application of linear-chain and hidden-state conditional random field (CRF) models to the task. A method for evaluating the significance of results quoted in terms of the normalised cross entropy (NCE) is also introduced. Instead of using sub-word-level information to improve wordlevel confidence scores, sub-word and word-level predictor features are combined to improve the accuracy of confidence scores in each sub-word being correct. The use of CRFs to model transitions between consecutive correct/incorrect sub-words yields large performance improvements. The scale of these gains is shown to increase further with the application of hidden-state CRFs. This is attributed to the fact that the hidden states make it possible for longer-span runs of consecutive correct/incorrect sub-words to be modelled, with these runs also not being constrained by word-level boundaries.
更多查看译文
关键词
feature extraction,speech recognition,statistical analysis,ASR system,NCE,automatic speech recognition,confidence scores,direct sub-word confidence estimation,hidden-state CRF model,hidden-state conditional random fields,linear-chain CRF model,normalised cross entropy,sub-word predictor features,word-level boundaries,word-level predictor features,Hidden-state conditional random fields,confidence estimation,sub-words
AI 理解论文
溯源树
样例
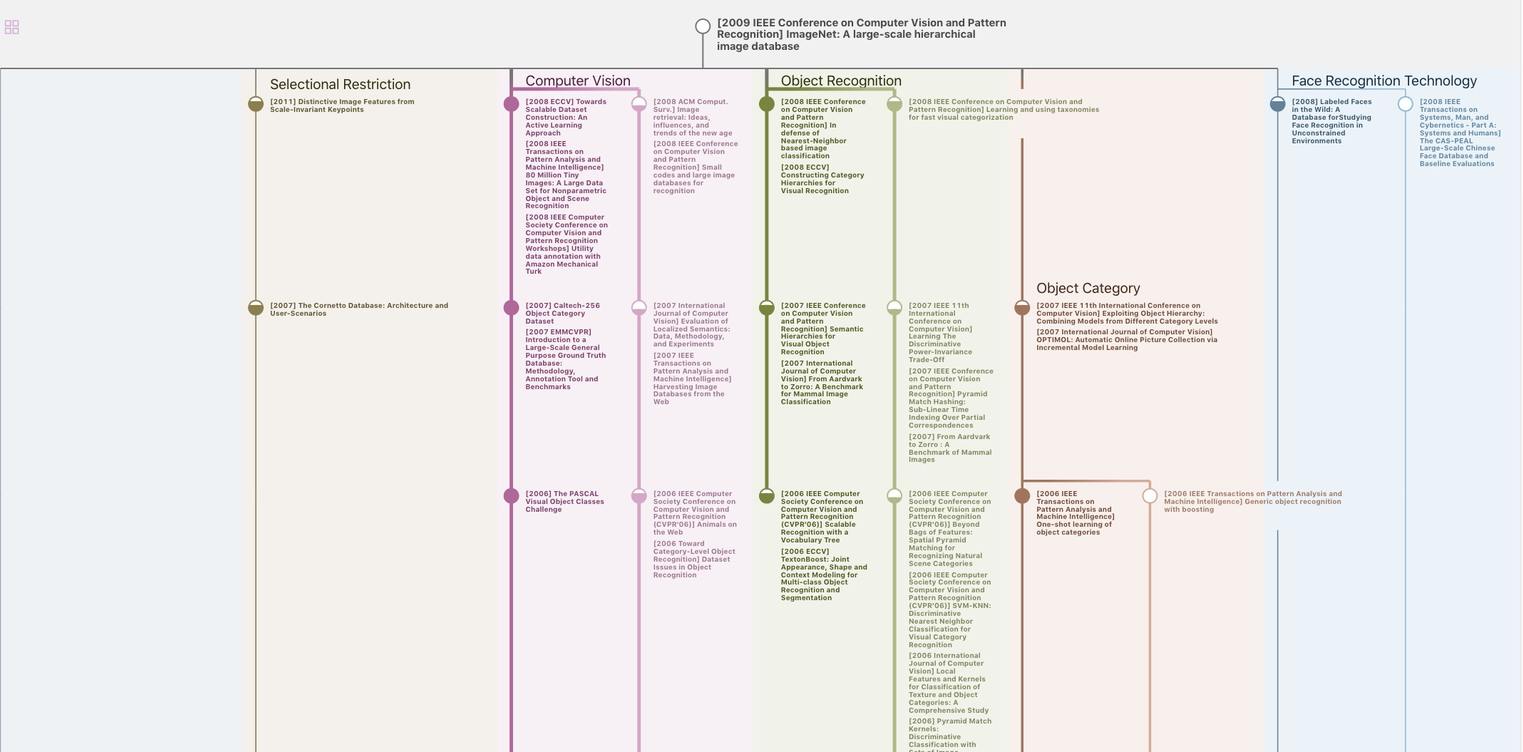
生成溯源树,研究论文发展脉络
Chat Paper
正在生成论文摘要