Constructive learning of recurrent neural networks
San Francisco, CA(1993)
摘要
It is difficult to determine the minimal neural network structure for a particular automaton. A large recurrent network in practice is very difficult to train. Constructive or destructive recurrent methods might offer a solution to this problem. It is proved that one current method, recurrent cascade correlation, has fundamental limitations in representation and thus in its learning capabilities. A preliminary approach to circumventing these limitations by devising a simple constructive training method that adds neurons during training while still preserving the powerful fully recurrent structure is given. Through simulations it is shown that such a method can learn many types of regular grammars which the recurrent cascade correlation method is unable to learn
更多查看译文
关键词
grammars,learning (artificial intelligence),recurrent neural nets,constructive training method,fully recurrent structure,minimal neural network structure,recurrent cascade correlation,recurrent neural networks,regular grammars,recurrent neural network,learning artificial intelligence,signal processing,neural networks,predictive models,upper bound,neural network,convergence
AI 理解论文
溯源树
样例
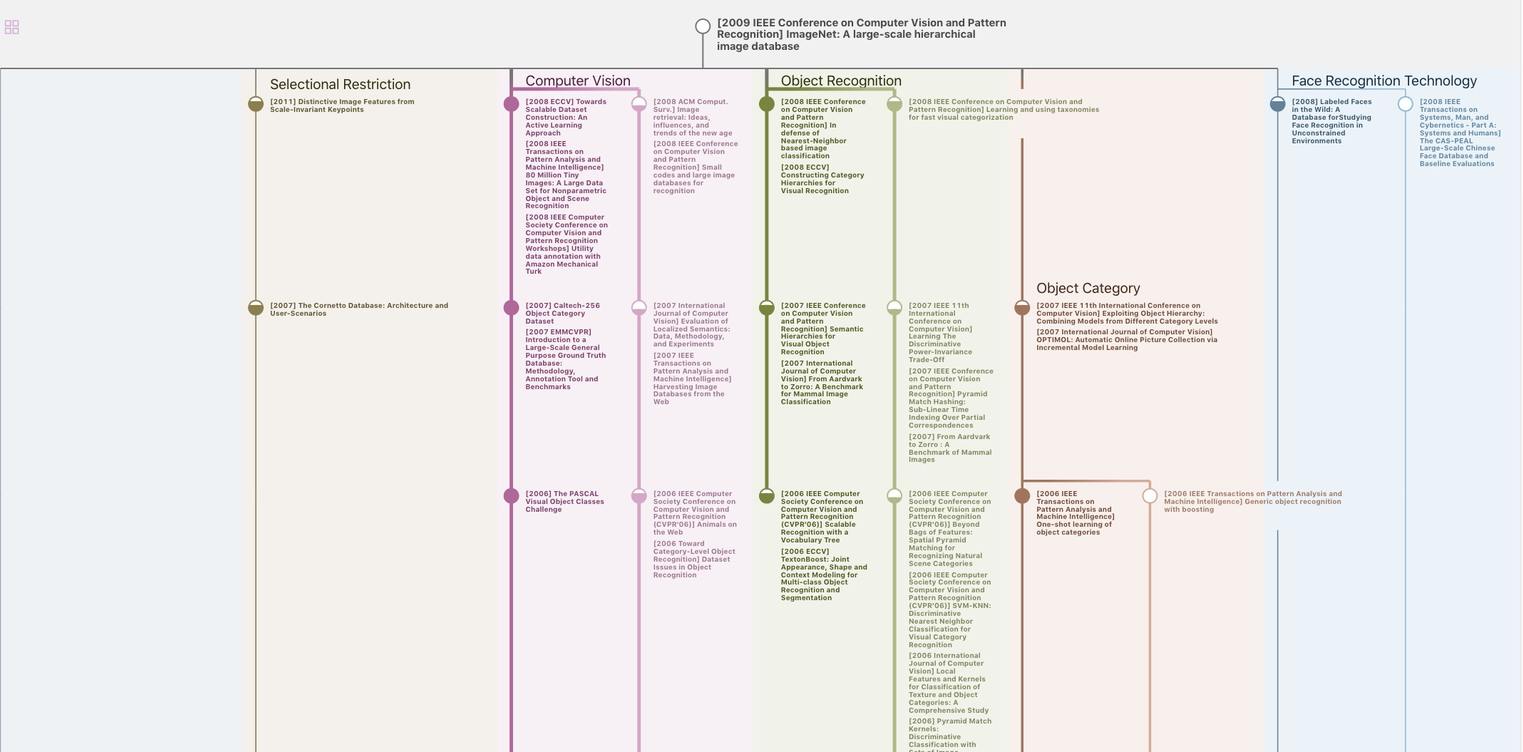
生成溯源树,研究论文发展脉络
Chat Paper
正在生成论文摘要