Introduction To The Sam-S M* And Mam-S M* Families
Neural Networks, 2005. IJCNN '05. Proceedings. 2005 IEEE International Joint Conference(2005)
摘要
In this paper, two new families of constructive Self-Organizing Maps (SOMs), SAM-SOM* and MAM-SOM*, are proposed. These families are specially useful for information retrieval from large databases, high-dimensional spaces and complex distance functions which usually consume a long time. They are generated by incorporating Spatial Access Method (SAM) and Metric Access Method (MAM) into SOM with the maximum insertion rate, i.e. the case when a new unit is created for each pattern presented to the network. In this specific case, the network presents interesting advantages and acquires new properties which are quite different of traditional SOM.In a constructive SOM, if new units are rarely inserted into network, the training algorithm would probably need a long time to converge. On the other hand, if new units are inserted frequently, the training algorithm would not have enough time to adapt these units to the data distribution. Besides, training time is increased because the search for the winning neuron is traditionally performed sequentially.The use of SAM and MAM combined with SOM open the possibility of training constructive SOM with as much units as existing patterns with less time and interesting advantages compared with both models: Kobonen network SOM and SAM-SOM model (SOM using SAM). Advantages and drawbacks of these new families are also discussed. These new families are useful to improve both SOM and SAM techniques.
更多查看译文
关键词
learning (artificial intelligence),self-organising feature maps,kohonen network,constructive self-organizing map,data distribution,metric access method,spatial access method,training algorithm,distance function,information retrieval,learning artificial intelligence,access method
AI 理解论文
溯源树
样例
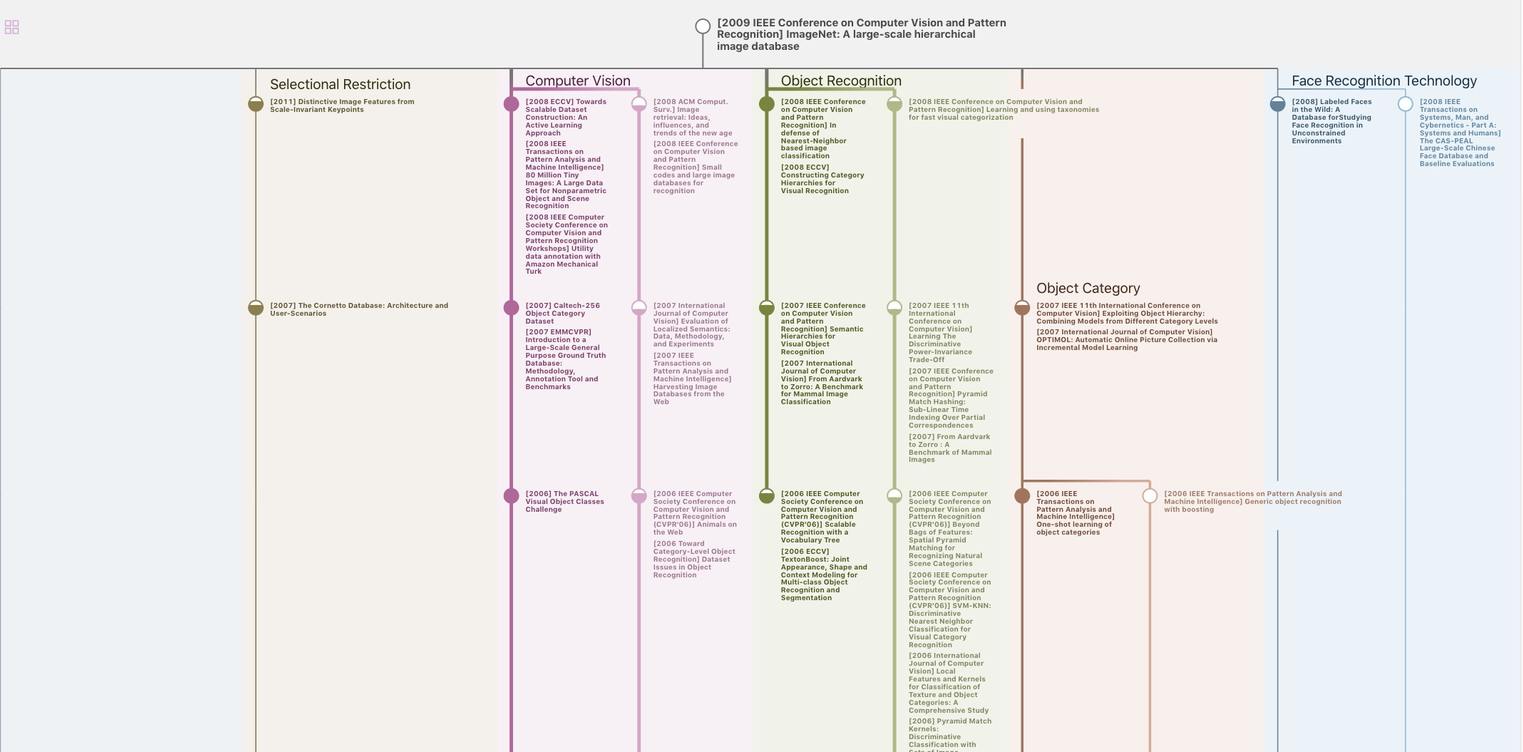
生成溯源树,研究论文发展脉络
Chat Paper
正在生成论文摘要