Handwritten Signatures Recognizer by Its Envelope and Strokes Layout Using HMM's
Clinical NeurophysiologySCI 3区
Abstract
A method for the automatic recognition of offline handwritten signatures using both global and local features is described. As global features, we use the envelope of the signature sequenced as polar coordinates; and as local features we use points located inside the envelope that describe the density or distribution of signature strokes. Each feature is processed as a sequence by a hidden Markov Model (HMM) classifier. The results of both classifiers are linearly combined, obtaining a recognition ratio of 95.15% with a database of 60 handwritten signatures
MoreTranslated text
Key words
handwriting recognition,handwritten character recognition,hidden Markov models,image recognition,HMM,automatic recognition,global features,handwritten signature database,handwritten signature recognizer,hidden Markov model classifier,local features,offline handwritten signature recognition,polar coordinates,recognition ratio,signature strokes,stroke layout
PDF
View via Publisher
AI Read Science
AI Summary
AI Summary is the key point extracted automatically understanding the full text of the paper, including the background, methods, results, conclusions, icons and other key content, so that you can get the outline of the paper at a glance.
Example
Background
Key content
Introduction
Methods
Results
Related work
Fund
Key content
- Pretraining has recently greatly promoted the development of natural language processing (NLP)
- We show that M6 outperforms the baselines in multimodal downstream tasks, and the large M6 with 10 parameters can reach a better performance
- We propose a method called M6 that is able to process information of multiple modalities and perform both single-modal and cross-modal understanding and generation
- The model is scaled to large model with 10 billion parameters with sophisticated deployment, and the 10 -parameter M6-large is the largest pretrained model in Chinese
- Experimental results show that our proposed M6 outperforms the baseline in a number of downstream tasks concerning both single modality and multiple modalities We will continue the pretraining of extremely large models by increasing data to explore the limit of its performance
Try using models to generate summary,it takes about 60s
Must-Reading Tree
Example
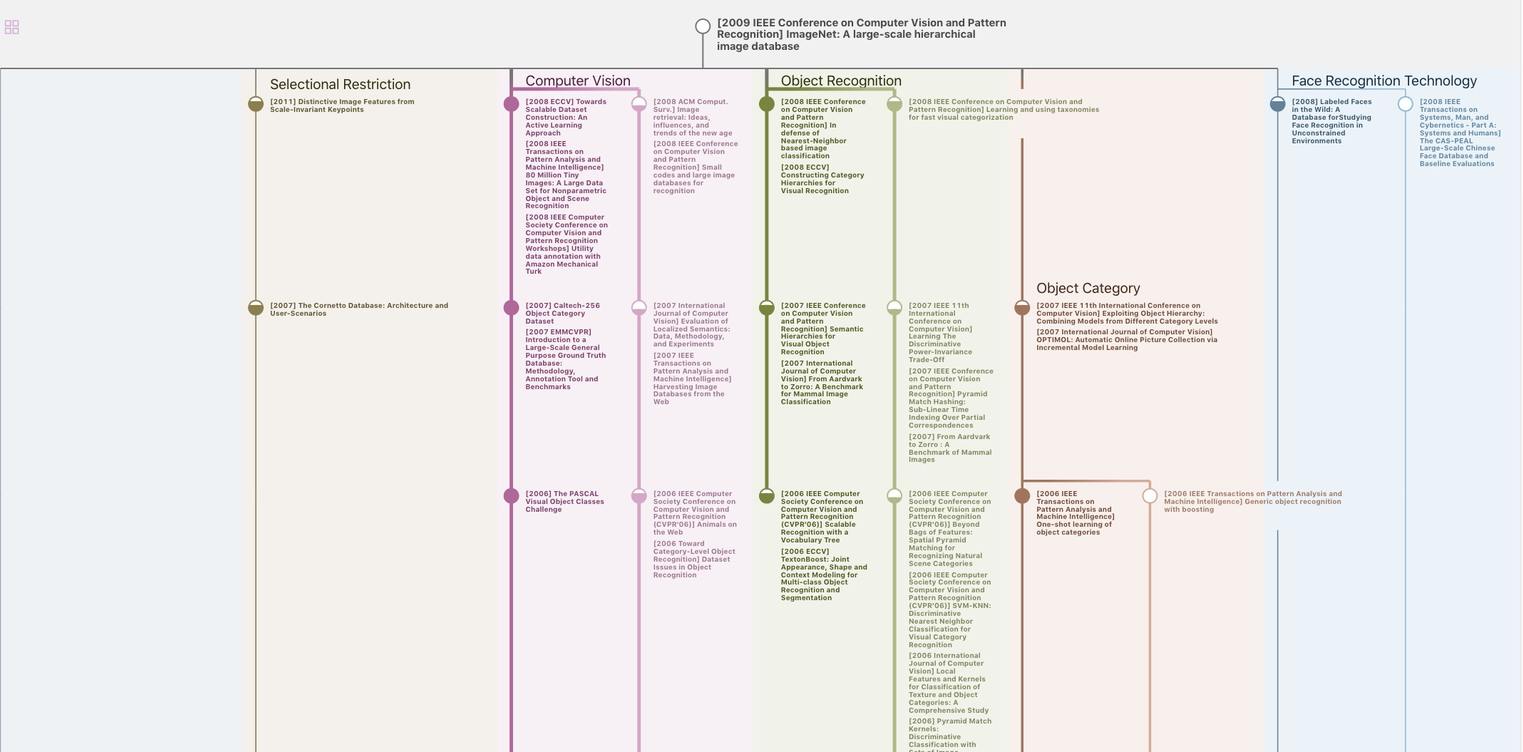
Generate MRT to find the research sequence of this paper
Data Disclaimer
The page data are from open Internet sources, cooperative publishers and automatic analysis results through AI technology. We do not make any commitments and guarantees for the validity, accuracy, correctness, reliability, completeness and timeliness of the page data. If you have any questions, please contact us by email: report@aminer.cn
Chat Paper
去 AI 文献库 对话