ACN: An associative classifier with negative rules
CSE(2008)
摘要
Classification using association rules has added a new dimension to the ongoing research for accurate classifiers. Experiments have shown that these classifiers are significantly more accurate than decision tree classifiers. The idea behind most of the existing approaches has been the mining of positive class association rules from the training set and then selecting a subset of the mined rules for future predictions. However, in most cases, it is found that the final classifier contains some weak and inaccurate rules that were selected for covering some training instances for which no better rules were available. These rules make poor predictions of unseen test instances and only for these rules, the overall classification accuracy is drastically reduced. The idea of this paper is to eliminate these weak and inaccurate positive rules as far as possible by accurate negative rules. The generation of negative associations from datasets has been attacked from different perspectives by various authors and this has proved to be a very computationally expensive task. This paper approaches the problem of generating negative rules from a classification perspective, how to generate a sufficient number of high quality negative rules efficiently so that classification accuracy is enhanced. We extend the a priori algorithm for this and show that our classifier ldquoassociative classifier with negative rulesrdquo (ACN) is not only time-efficient but also achieves significantly better accuracy than four other state-of-the-art classification methods by experimenting on benchmark UCI datasets.
更多查看译文
关键词
testing,association rules,decision tree classifier,decision trees,classification algorithms,computer science,databases,data mining,association rule,training data,accuracy,law,training set
AI 理解论文
溯源树
样例
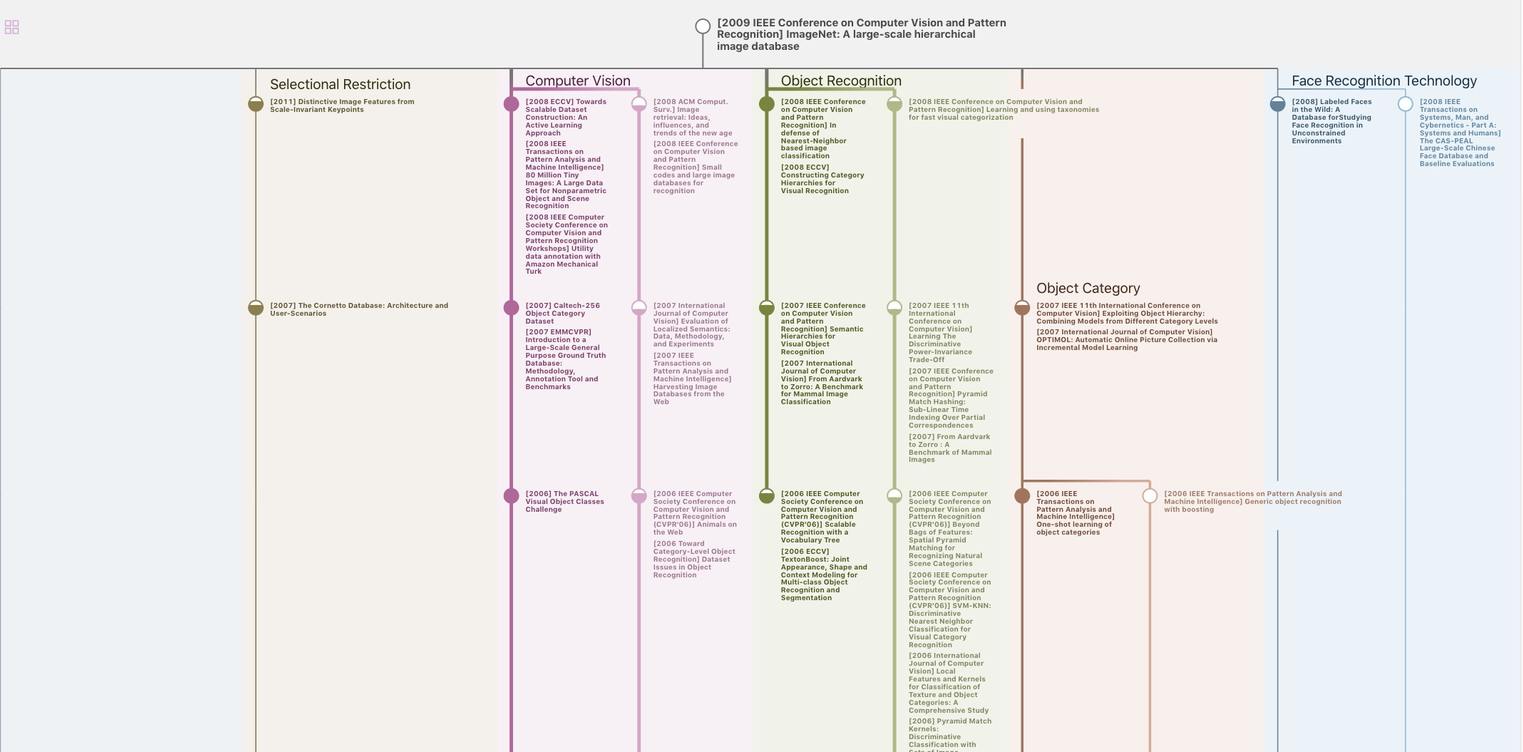
生成溯源树,研究论文发展脉络
Chat Paper
正在生成论文摘要