Interactive Learning in Continuous Multimodal Space: A Bayesian Approach to Action-Based Soft Partitioning and Learning
IEEE T. Autonomous Mental Development(2012)
摘要
A probabilistic framework for interactive learning in continuous and multimodal perceptual spaces is proposed. In this framework, the agent learns the task along with adaptive partitioning of its multimodal perceptual space. The learning process is formulated in a Bayesian reinforcement learning setting to facilitate the adaptive partitioning. The partitioning is gradually and softly done using Gaussian distributions. The parameters of distributions are adapted based on the agent's estimate of its actions' expected values. The probabilistic nature of the method results in experience generalization in addition to robustness against uncertainty and noise. To benefit from experience generalization diversity in different perceptual subspaces, the learning is performed in multiple perceptual subspaces-including the original space-in parallel. In every learning step, the policies learned in the subspaces are fused to select the final action. This concurrent learning in multiple spaces and the decision fusion result in faster learning, possibility of adding and/or removing sensors-i.e., gradual expansion or contraction of the perceptual space-, and appropriate robustness against probable failure of or ambiguity in the data of sensors. Results of two sets of simulations in addition to some experiments are reported to demonstrate the key properties of the framework.
更多查看译文
关键词
reinforcement learning,probability distribution,uncertainty,bayesian learning,intelligent sensors,bayesian methods,expected value,learning artificial intelligence,bayesian approach,mathematical model,gaussian distribution,bayesian method
AI 理解论文
溯源树
样例
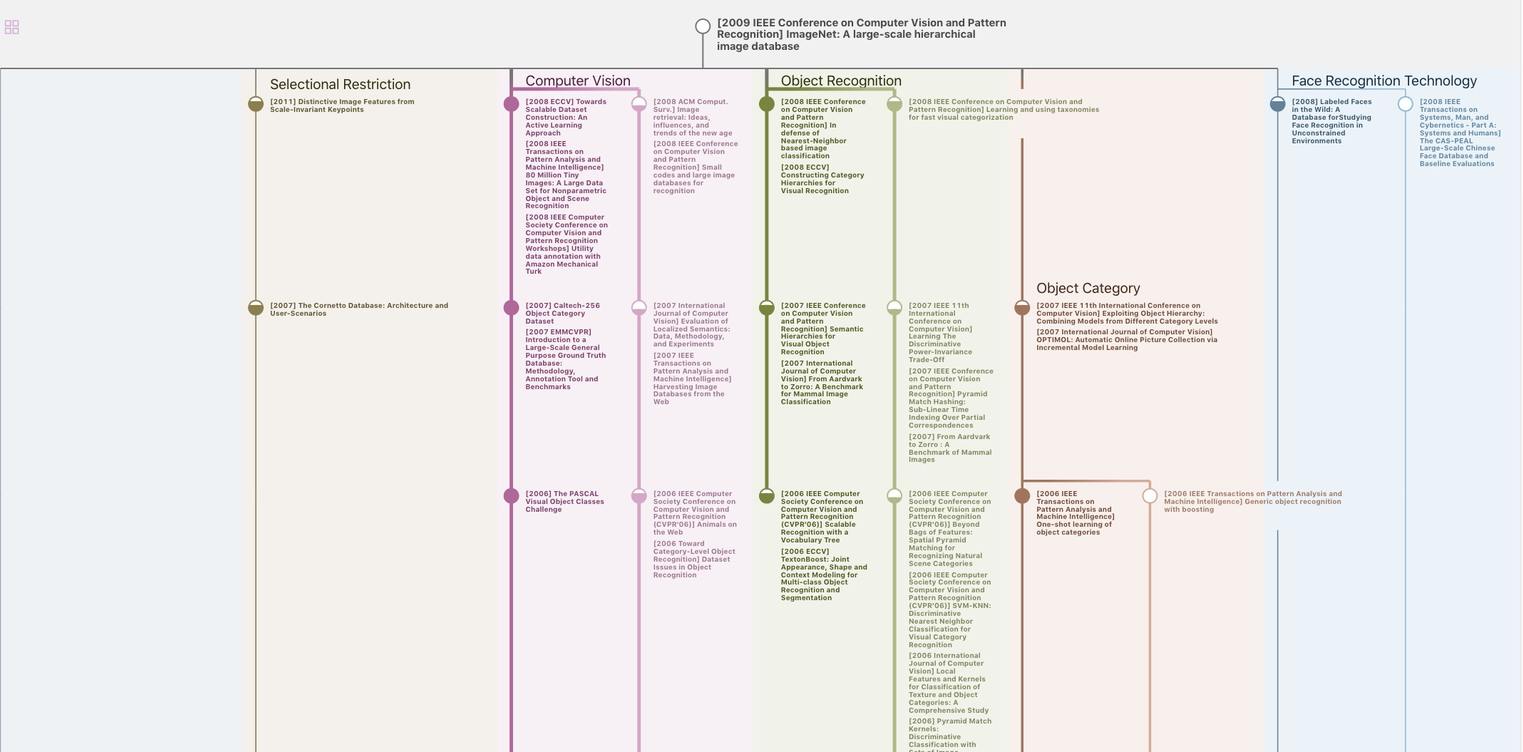
生成溯源树,研究论文发展脉络
Chat Paper
正在生成论文摘要