Efficient temporal decomposition of local field potentials
Machine Learning for Signal Processing(2011)
摘要
Local field potentials (LFPs) arise from dendritic currents that are summed by the brain tissue's impedance. By assuming that the rhythms existing in the LFPs result from the coordinated neural activity of sparse and transient neural assemblies transformed by the neural tissue, we propose to recover these neural assemblies sources using an independent component analysis on segments of a single LFP channel. The corresponding source signals and the set of temporal filters that operate on them constitute an efficient time-frequency decomposition of the LFP. This decomposition has the potential to identify sources that are more statistically dependent with stimuli or single-cell activity than the raw signal. In this work we show preliminary results on a synthetic dataset and a real dataset recorded from a rats nucleus accumbens during a reward administering experiment. When compared with the standard time-frequency analysis, this computational model for LFP analysis is totally data-driven because the filters, which form the basis for the decomposition, are estimated directly from the data.
更多查看译文
关键词
bioelectric potentials,biological tissues,brain,cellular biophysics,decomposition,electric impedance,independent component analysis,medical signal processing,neurophysiology,time-frequency analysis,lfp channel,brain tissue impedance,dendritic current,local field potential,neural activity,neural tissue,nucleus accumbens,single cell activity,sparse neural assembly,stimuli,synthetic dataset,temporal decomposition,temporal filter,time frequency analysis,time frequency decomposition,transient neural assembly,ica,multiscale neural signal analysis,statistical decoding,computer model,time frequency,signal analysis
AI 理解论文
溯源树
样例
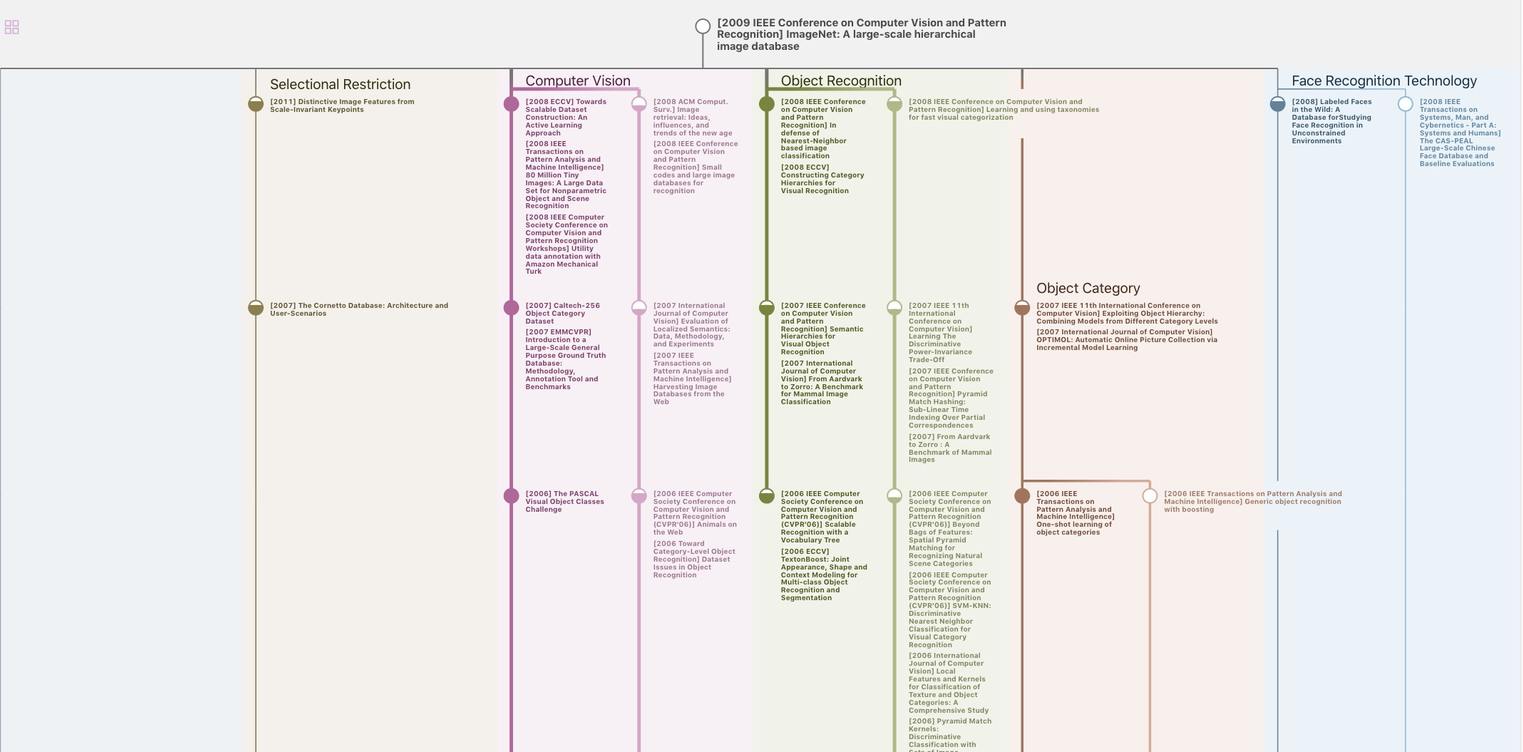
生成溯源树,研究论文发展脉络
Chat Paper
正在生成论文摘要