Exploring spatial characteristics of urban transportation networks
ITSC(2011)
摘要
It has been shown recently that a Macroscopic Fundamental Diagram (MFD) exists in urban transportation networks under certain conditions. However, MFD is not universally expected. Previous research demonstrates the existence of MFDs in homogeneous networks with similar link densities. More recent work focuses on the partitioning of a heterogeneous transportation network based on different congestion levels. A desired partitioning produces homogeneous regions with similar link densities to guarantee a well-defined MFD and spatially compact shapes to ease the implementation of control measurements [1]. Based on recently proposed partitioning mechanism, this paper further explores the spatial characteristics of sub-networks (sub-regions or clusters) in urban transportation networks. In this paper, a metric is defined to evaluate the spatial compactness of each cluster in the network. In order to obtain the metric, a fast graph traversal algorithm is proposed, which can produce a clockwise sequence for the spatially coordinated boundary nodes along a network. The algorithm takes O(n) and the effectiveness is proved and validated. By applying the boundary smoothness metric to our previous clustering results, we show that the spatial compactness is appropriately guaranteed for each region and the future control policies can therefore be easily implemented based on the partitioning and MFDs. The proposed algorithms can have more general applications in fields of network and graph theory.
更多查看译文
关键词
computational complexity,diagrams,graph theory,network theory (graphs),road traffic,roads,different congestion level,graph traversal algorithm,heterogeneous transportation network,homogeneous network,macroscopic fundamental diagram,network theory,partitioning mechanism,similar link density,spatial characteristics,spatially compact shape,spatially coordinated boundary node,urban transportation network,networks,algorithms,cluster analysis
AI 理解论文
溯源树
样例
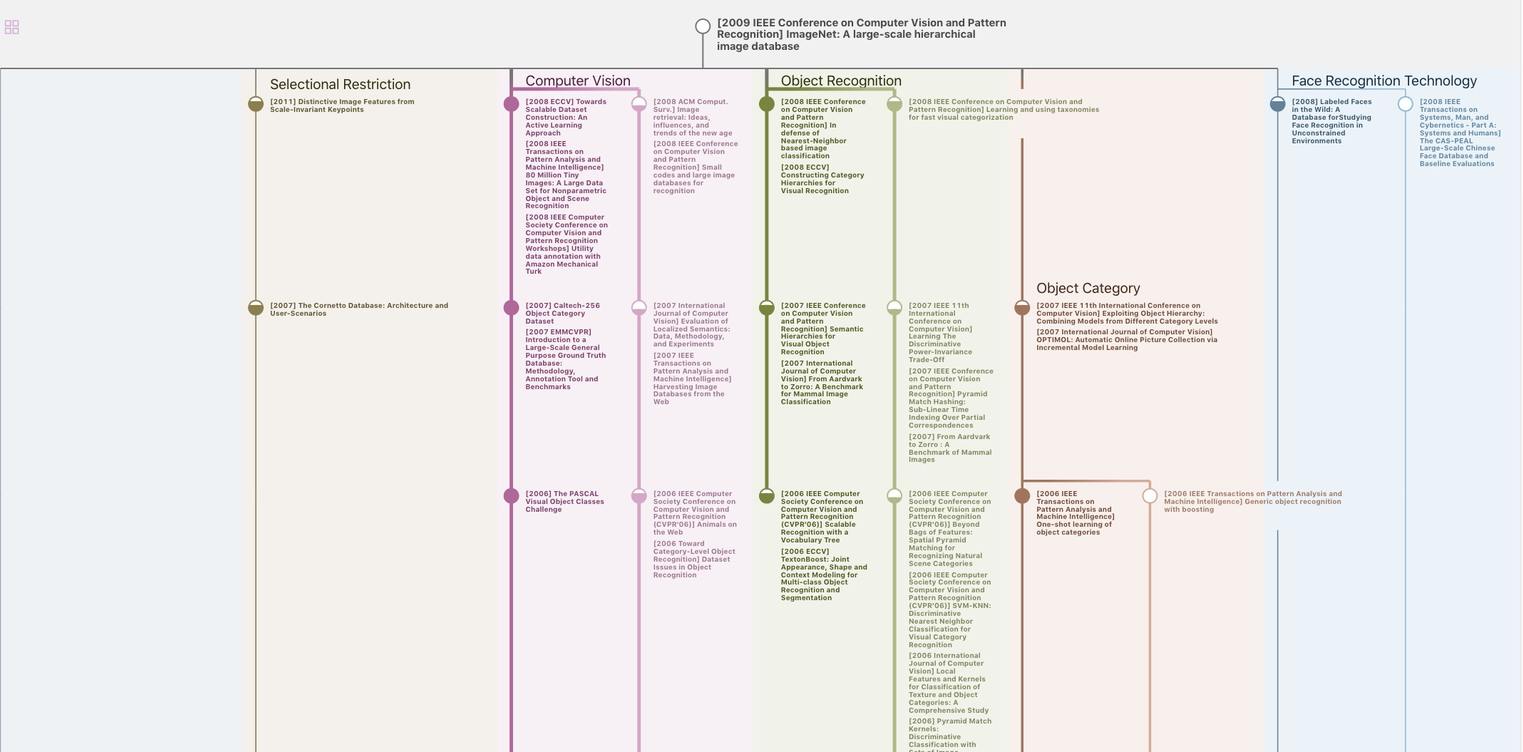
生成溯源树,研究论文发展脉络
Chat Paper
正在生成论文摘要