Unsupervised topic modeling for leader detection in spoken discourse
Acoustics, Speech and Signal Processing(2012)
摘要
In this paper, we describe a method for leader detection in multi-party spoken discourse that relies on unsupervised topic modeling to segment the discourse automatically. Latent Dirichlet allocation is applied to sliding temporal windows of utterances, resulting in a topic model which captures the fluid transitions from topic to topic which occur in multi-party discourse. Further processing discretizes the continuous topic mixtures into sequential topic segments. Features are extracted from topic shift regions and used to train a binary role classifier. The added topic shift features significantly improve the baseline performance on two corpora, demonstrating both the value of the features and the robustness of the unsupervised segmentation. Furthermore, our classification results on the ICSI corpus, using automatically segmented topics, are better than the results using ground truth segmentations.
更多查看译文
关键词
feature extraction,speech processing,ICSI corpus,binary role classifier,discourse segmentation,feature extraction,fluid transitions,ground truth segmentations,latent Dirichlet allocation,leader detection,multiparty spoken discourse,sliding temporal windows,unsupervised segmentation,unsupervised topic modeling,Social role classification,latent Dirichlet allocation,leader detection,speaker role labeling,topic shift analysis
AI 理解论文
溯源树
样例
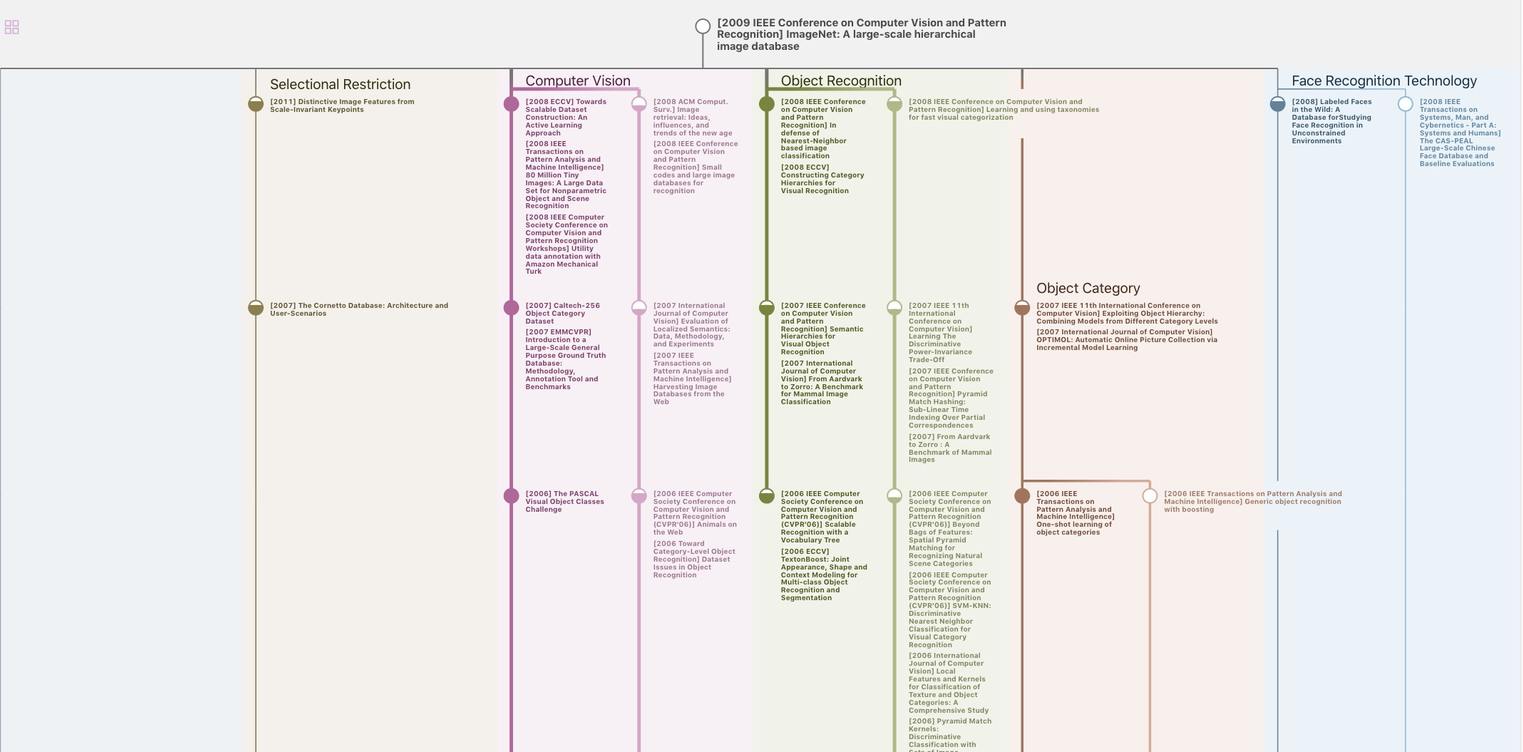
生成溯源树,研究论文发展脉络
Chat Paper
正在生成论文摘要