Improving automatic sound-based fall detection using iVAT clustering and GA-based feature selection.
EMBC(2012)
摘要
Falls represent an important health problem for older adults. This issue continues to generate interest in the research and development of fall detection systems. In previous work we proposed an acoustic fall detection system (acoustic-FADE) that employs an 8-microphone circular array to automatically detect falls. Acoustic-FADE has achieved encouraging results: 100% detection at 3% false alarm rate in laboratory tests. In this paper, we use a dataset from previous work to investigate how to further improve AFADE performance. To analyze the relationship between fall and non-fall signatures we used the improved visual assessment of tendency (iVAT) clustering algorithm in conjunction with a nearest neighbor based distance to find the most challenging false alarms. Then, we employed a genetic algorithm (GA) framework to perform feature selection and find the mel-frequency cepstral coefficients (MFCC) that improve the classification performance. We found that using only three MFCC coefficients (1, 28, 29) instead of our previous choice (1,2,3,4,5,6) improves the classification performance.
更多查看译文
关键词
biomechanics,ivat clustering,automatic sound-based fall detection,geriatrics,microphones,medical signal processing,mel-frequency cepstral coefficients,visual assessment of tendency clustering algorithm,feature extraction,acoustic fall detection system,eight microphone circular array,genetic algorithm,nearest neighbor based distance,signal classification,genetic algorithms,ga-based feature selection,classification performance,older adults,ergonomics,suicide prevention,human factors,occupational safety,injury prevention
AI 理解论文
溯源树
样例
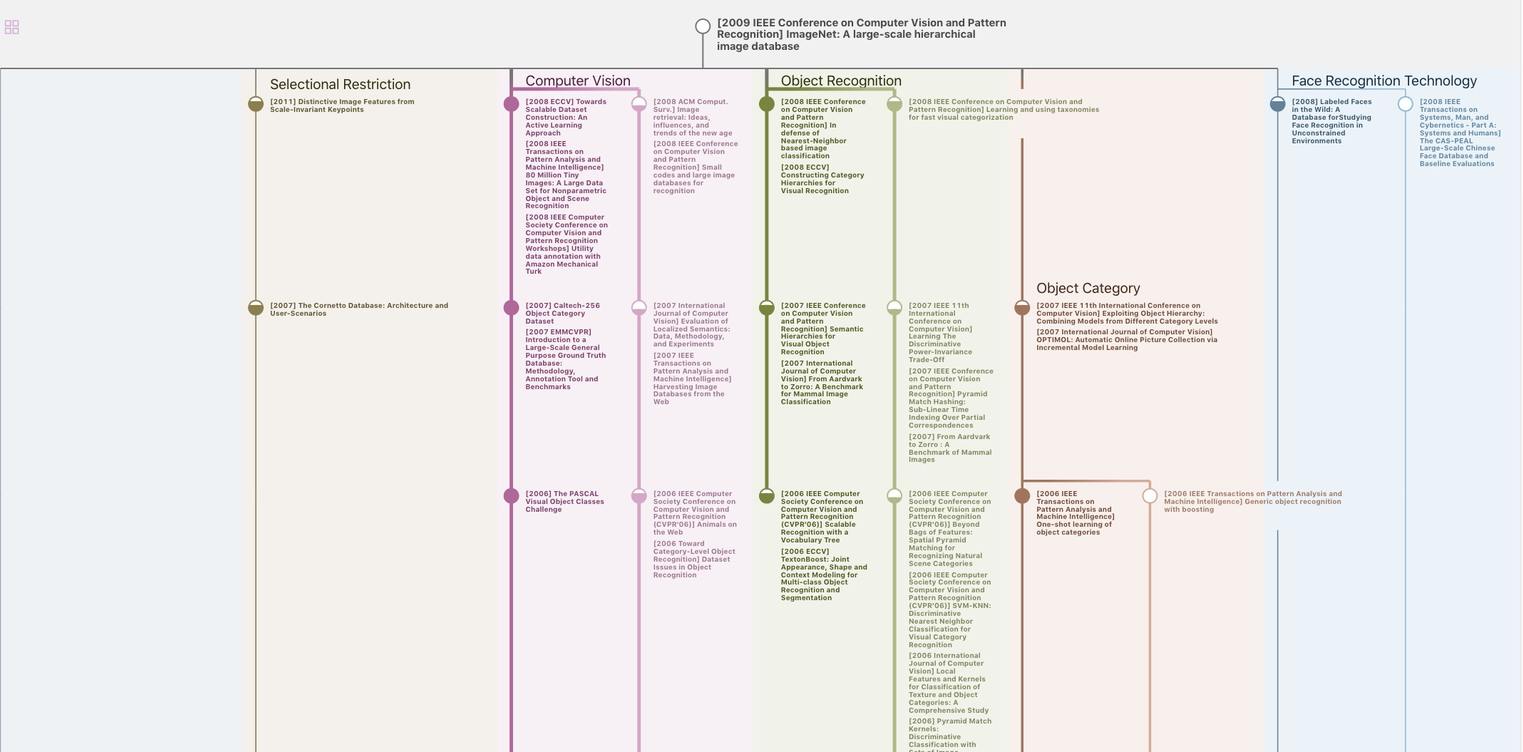
生成溯源树,研究论文发展脉络
Chat Paper
正在生成论文摘要