PSO Based Neural Networks Vs. Traditional Statistical Models for Seasonal Time Series Forecasting
Advance Computing Conference(2013)
摘要
Seasonality is a distinctive characteristic which is often observed in many practical time series. Artificial Neural Networks (ANNs) are a class of promising models for efficiently recognizing and forecasting seasonal patterns. In this paper, the Particle Swarm Optimization (PSO) approach is used to enhance the forecasting strengths of feedforward ANN (FANN) as well as Elman ANN (EANN) models for seasonal data. Three widely popular versions of the basic PSO algorithm, viz. Trelea-I, Trelea-II and Clerc-Type1 are considered here. The empirical analysis is conducted on three real-world seasonal time series. Results clearly show that each version of the PSO algorithm achieves notably better forecasting accuracies than the standard Backpropagation (BP) training method for both FANN and EANN models. The neural network forecasting results are also compared with those from the three traditional statistical models, viz. Seasonal Autoregressive Integrated Moving Average (SARIMA), Holt-Winters (HW) and Support Vector Machine (SVM). The comparison demonstrates that both PSO and BP based neural networks outperform SARIMA, HW and SVM models for all three time series datasets. The forecasting performances of ANNs are further improved through combining the outputs from the three PSO based models.
更多查看译文
关键词
feedforward neural nets,forecasting theory,particle swarm optimisation,pattern recognition,statistical analysis,time series,Clerc-Type1 algorithm,EANN model,Elman ANN model,FANN,PSO-based neural networks,Trelea-I algorithm,Trelea-II algorithm,artificial neural networks,feedforward ANN,forecasting performance improvement,forecasting strengths,particle swarm optimization,seasonal data,seasonal pattern forecasting,seasonal pattern recognition,seasonal time series forecasting,traditional statistical models,ANN,Box-Jenkins models,Elman ANN,particle swarm optimization,seasonality,time series forecasting
AI 理解论文
溯源树
样例
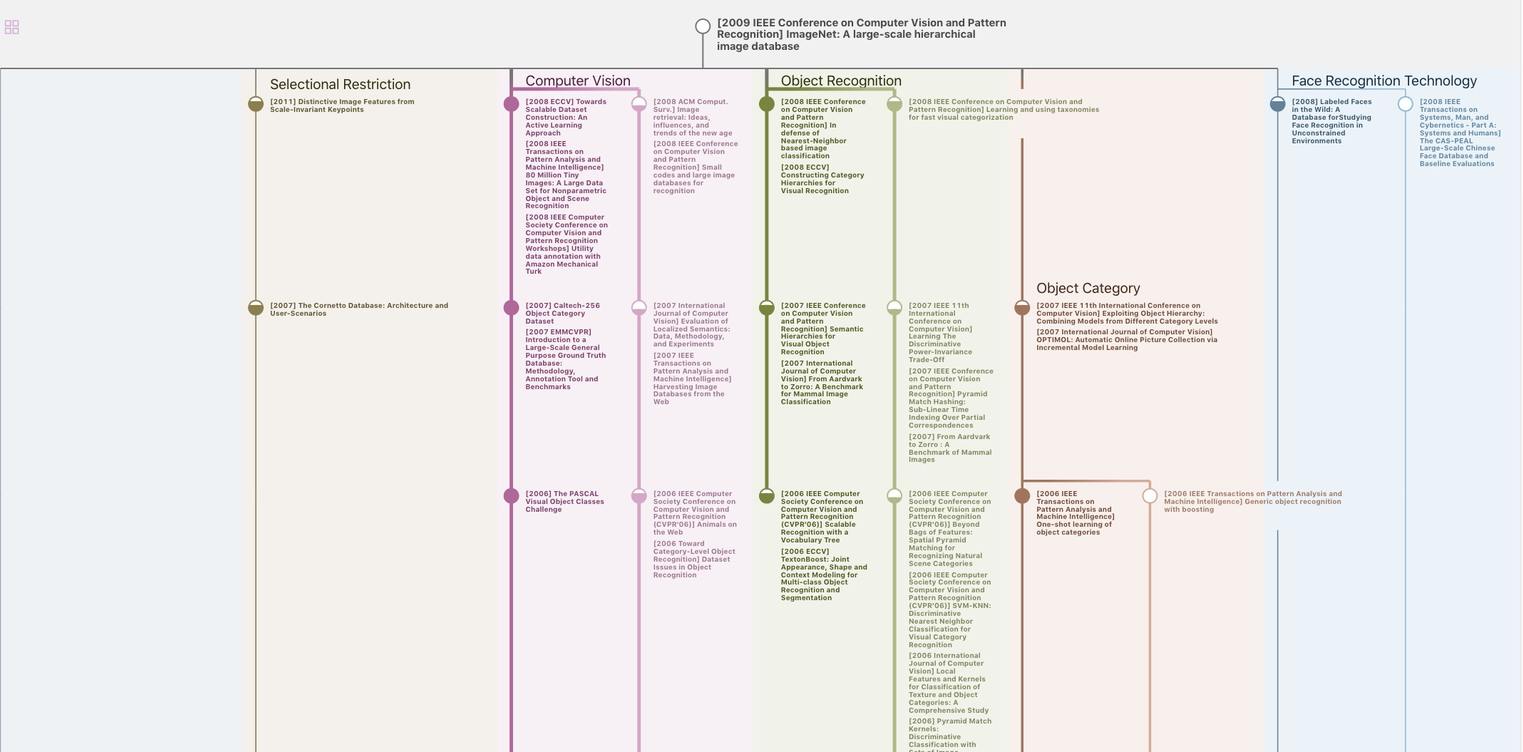
生成溯源树,研究论文发展脉络
Chat Paper
正在生成论文摘要