FACISME: Fuzzy associative classification using iterative scaling and maximum entropy
Fuzzy Systems(2010)
摘要
All associative classifiers developed till now are crisp in nature, and thus use sharp partitioning to transform numerical attributes to binary ones like “Income = [100K and above]”. On the other hand, the novel fuzzy associative classification algorithm called FACISME, which we propose in this paper, uses fuzzy logic to convert numerical attributes to fuzzy attributes, like “Income = High”, thus maintaining the integrity of information conveyed by such numerical attributes. Moreover, FACISME is based on maximum entropy, and uses iterative scaling, both of which lend a very strong theoretical foundation to the algorithm. Entropy is one of the best measures of information, and maximum-entropy-based algorithms do not assume independence of parameters in the classification process. Thus, FACISME provides very good accuracy, and can work with all types of datasets (irrespective of size and type of attributes - numerical or binary) and domains.
更多查看译文
关键词
data mining,entropy,fuzzy logic,iterative methods,classification process,dataset,fuzzy associative classification algorithm,fuzzy attribute,fuzzy logic,information integrity,iterative scaling,maximum entropy,numerical attribute,parameters independence
AI 理解论文
溯源树
样例
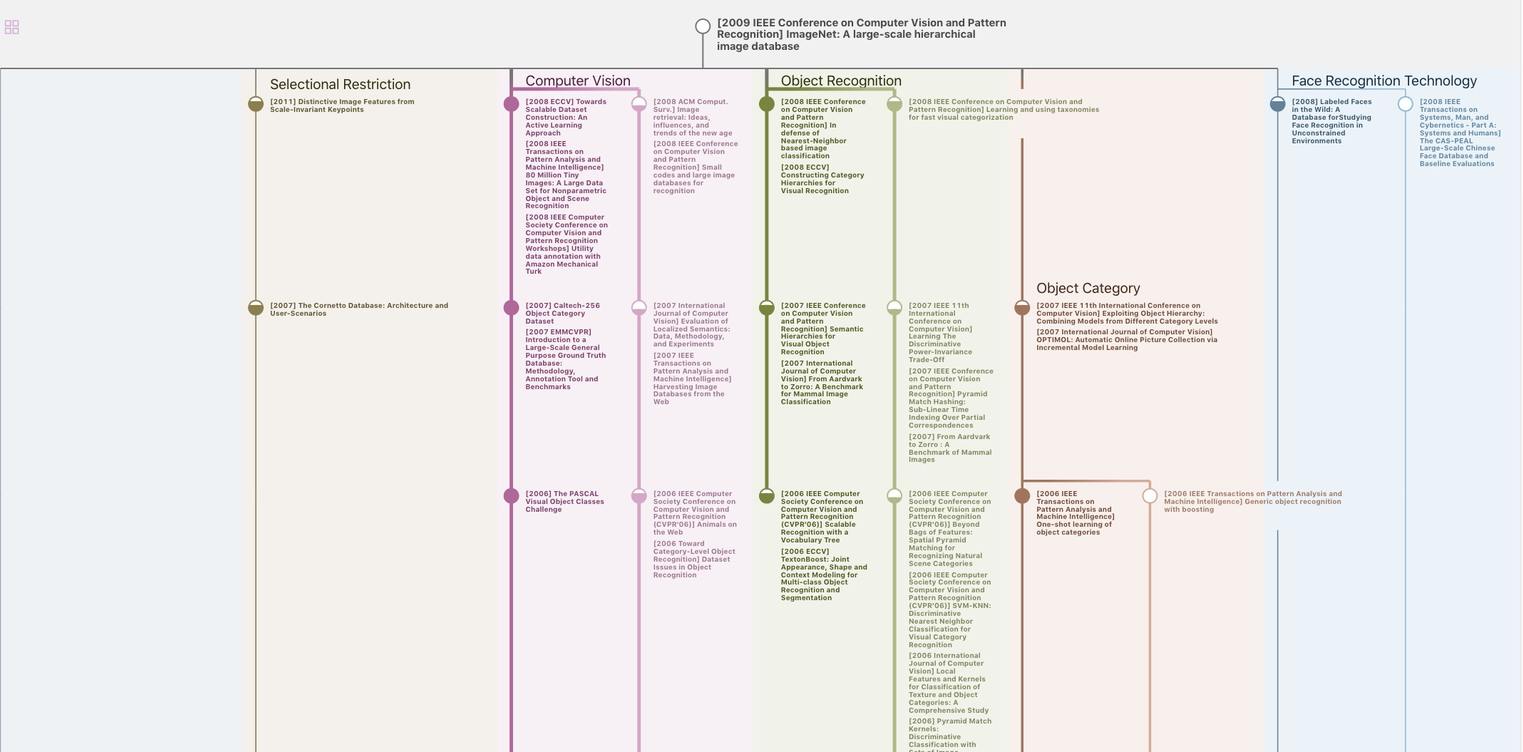
生成溯源树,研究论文发展脉络
Chat Paper
正在生成论文摘要