Fixed points of generalized approximate message passing with arbitrary matrices
Information Theory Proceedings(2016)
摘要
The estimation of a random vector with independent components passed through a linear transform followed by a componentwise (possibly nonlinear) output map arises in a range of applications. Approximate message passing (AMP) methods, based on Gaussian approximations of loopy belief propagation, have recently attracted considerable attention for such problems. For large random transforms, these methods exhibit fast convergence and admit precise analytic characterizations with testable conditions for optimality, even for certain non-convex problem instances. However, the behavior of AMP under general transforms is not fully understood. In this paper, we consider the generalized AMP (GAMP) algorithm and relate the method to more common optimization techniques. This analysis enables a precise characterization of the GAMP algorithm fixed-points that applies to arbitrary transforms. In particular, we show that the fixed points of the so-called max-sum GAMP algorithm for MAP estimation are critical points of a constrained maximization of the posterior density. The fixed-points of the sum-product GAMP algorithm for estimation of the posterior marginals can be interpreted as critical points of a certain mean-field variational optimization.
更多查看译文
关键词
Gaussian processes,approximation theory,convex programming,matrix algebra,message passing,AMP methods,GAMP algorithm,Gaussian approximations,arbitrary matrices,constrained maximization,fixed points,generalized AMP,generalized approximate message passing,independent components,linear transform,mean-field variational optimization,nonconvex problem,optimization techniques,output map,random vector estimation,ADMM,Belief propagation,message passing,variational optimization
AI 理解论文
溯源树
样例
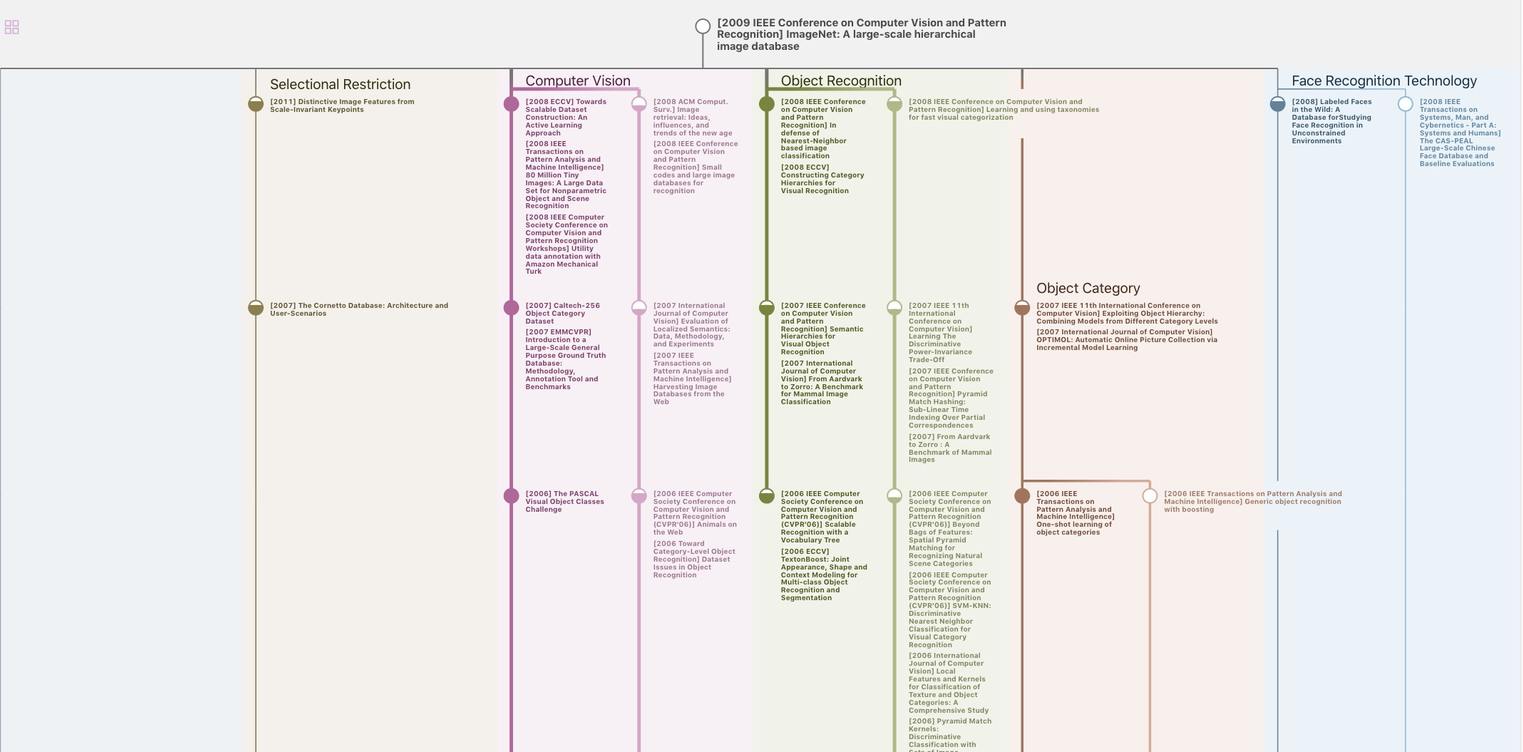
生成溯源树,研究论文发展脉络
Chat Paper
正在生成论文摘要