Asymptotically minimax regret by Bayes mixtures for non-exponential families
Information Theory Workshop(2013)
摘要
We study the problems of data compression, gambling and prediction of a sequence xn = x1x2...xn from an alphabet X, in terms of regret with respect to various families of probability distributions. It is known that the regret of the Bayes mixture with respect to a general exponential families asymptotically achieves the minimax value when variants of Jeffreys prior are used, under the condition that the maximum likelihood estimate is in the interior of the parameter space. We discuss a modification of Jeffreys prior which has measure outside the given family of densities, to achieve minimax regret with respect to non-exponential type families, e.g. curved exponential families and mixture families. These results also provide characterization of Rissanen's stochastic complexity for those classes.
更多查看译文
关键词
Bayes methods,data compression,maximum likelihood estimation,minimax techniques,sequences,statistical distributions,stochastic processes,Bayes mixtures,Jeffreys prior,Rissanen's stochastic complexity,alphabet,asymptotically minimax regret,data compression,gambling,maximum likelihood estimation,nonexponential families,prediction,probability distributions,sequence
AI 理解论文
溯源树
样例
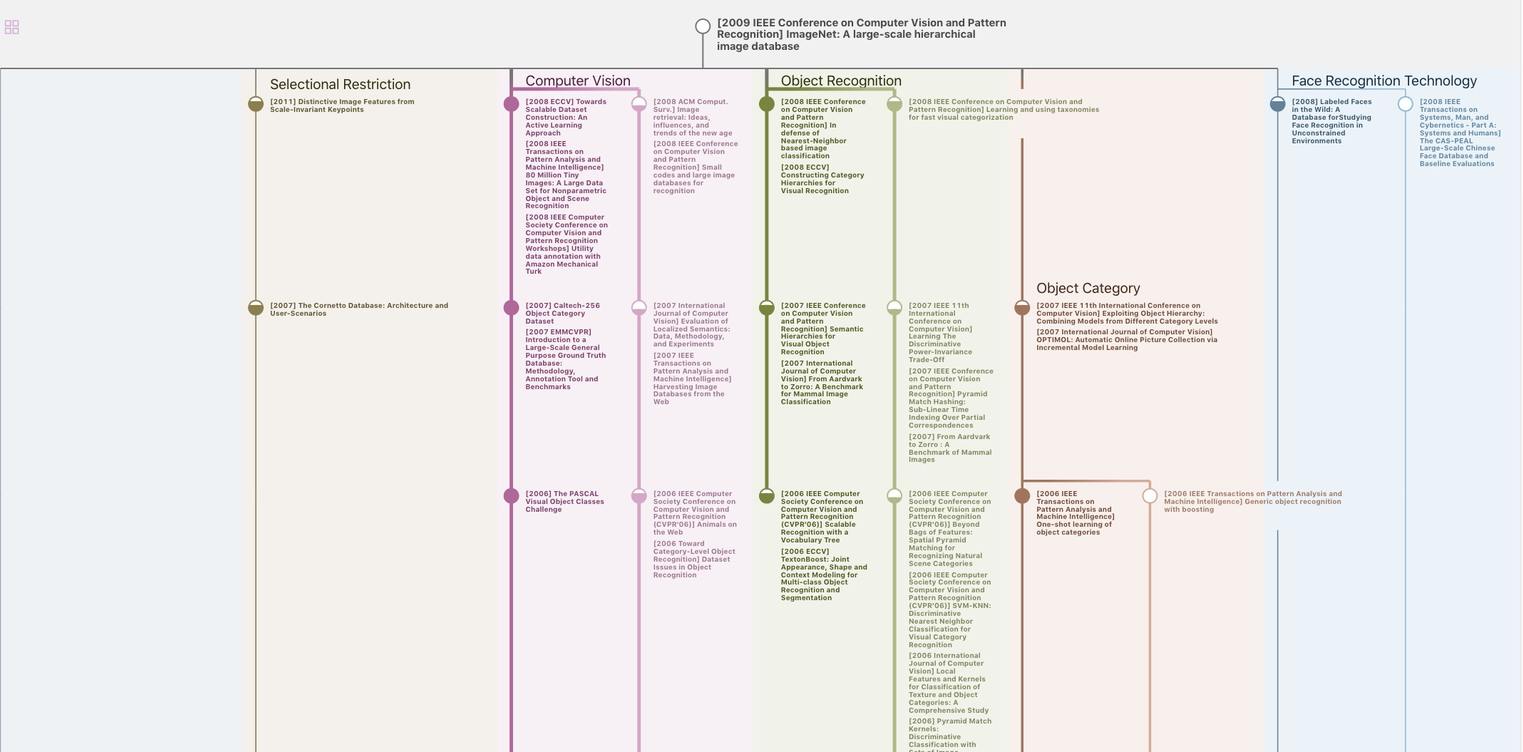
生成溯源树,研究论文发展脉络
Chat Paper
正在生成论文摘要