Optimization of emergency response using higher order learning and clustering of 911 text messages
Technologies for Homeland Security(2013)
摘要
In real-time emergency response an accurate picture of the situation is needed quickly. Often during large-scale disasters, cell towers become overloaded, and the only way of communication is through text messages. It becomes important to gather information from text messages sent to emergency numbers in order to respond quickly and efficiently with life-saving efforts. In addition, responders are unable to manually handle the large volume of incoming texts. To add to this difficult problem, these data sources tend to be microtext. This research developed a methodology to summarize text messages sent during an emergency, including analysis of locations. The real-time disaster needs were then input into a mixed integer programming resource allocation model for distribution of resources for disaster aid. Prior research included resource allocation and text modeling, but the combination of the two is a novel application not only in this arena, but more broadly across domains.
更多查看译文
关键词
emergency management,integer programming,learning (artificial intelligence),pattern clustering,resource allocation,disaster aid,emergency numbers,emergency response optimization,higher order learning,information gathering,mixed integer programming resource allocation model,text message clustering,text modeling,ho-lda,honb,higher order latent dirichlet allocation,higher order naïve bayes,higher order topic modeling,lda,latent dirichlet allocation,disaster response,machine learning,mixed integer programming,operations research,optimization,topic modeling,learning artificial intelligence
AI 理解论文
溯源树
样例
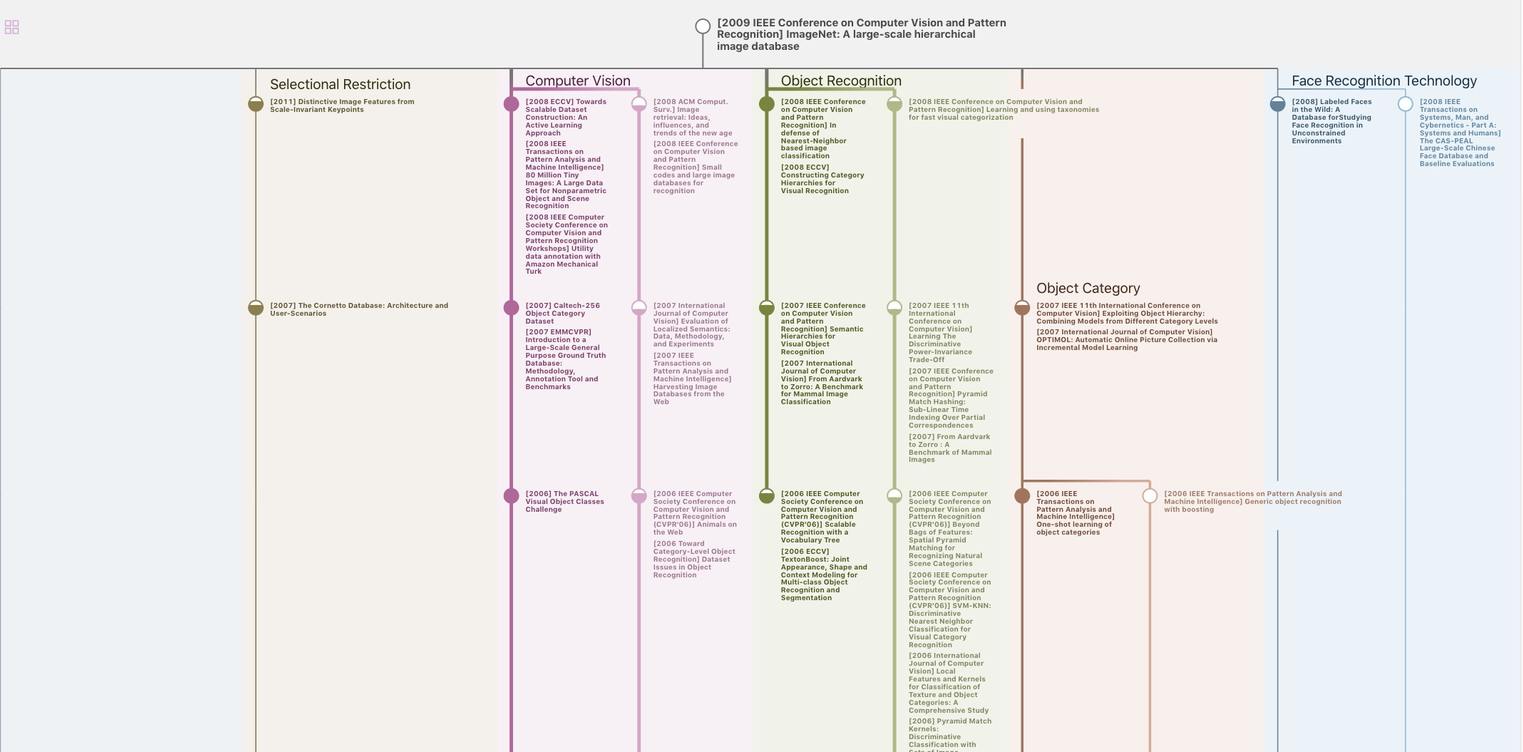
生成溯源树,研究论文发展脉络
Chat Paper
正在生成论文摘要