Most-Surely vs. Least-Surely Uncertain
Data Mining(2013)
摘要
Active learning methods aim to choose the most informative instances to effectively learn a good classifier. Uncertainty sampling, arguably the most frequently utilized active learning strategy, selects instances which are uncertain according to the model. In this paper, we propose a framework that distinguishes between two types of uncertainties: a model is uncertain about an instance due to strong and conflicting evidence (most-surely uncertain) vs. a model is uncertain about an instance because it does not have conclusive evidence (least-surely uncertain). We show that making a distinction between these uncertainties makes a huge difference to the performance of active learning. We provide a mathematical formulation to distinguish between these uncertainties for naive Bayes, logistic regression and support vector machines and empirically evaluate our methods on several real-world datasets.
更多查看译文
关键词
Bayes methods,learning (artificial intelligence),pattern classification,regression analysis,support vector machines,uncertain systems,active learning method,active learning strategy,least-surely uncertain,logistic regression,mathematical formulation,most-surely uncertain,naive Bayes method,real-world datasets,support vector machines,uncertainty sampling,Active learning,uncertainty sampling
AI 理解论文
溯源树
样例
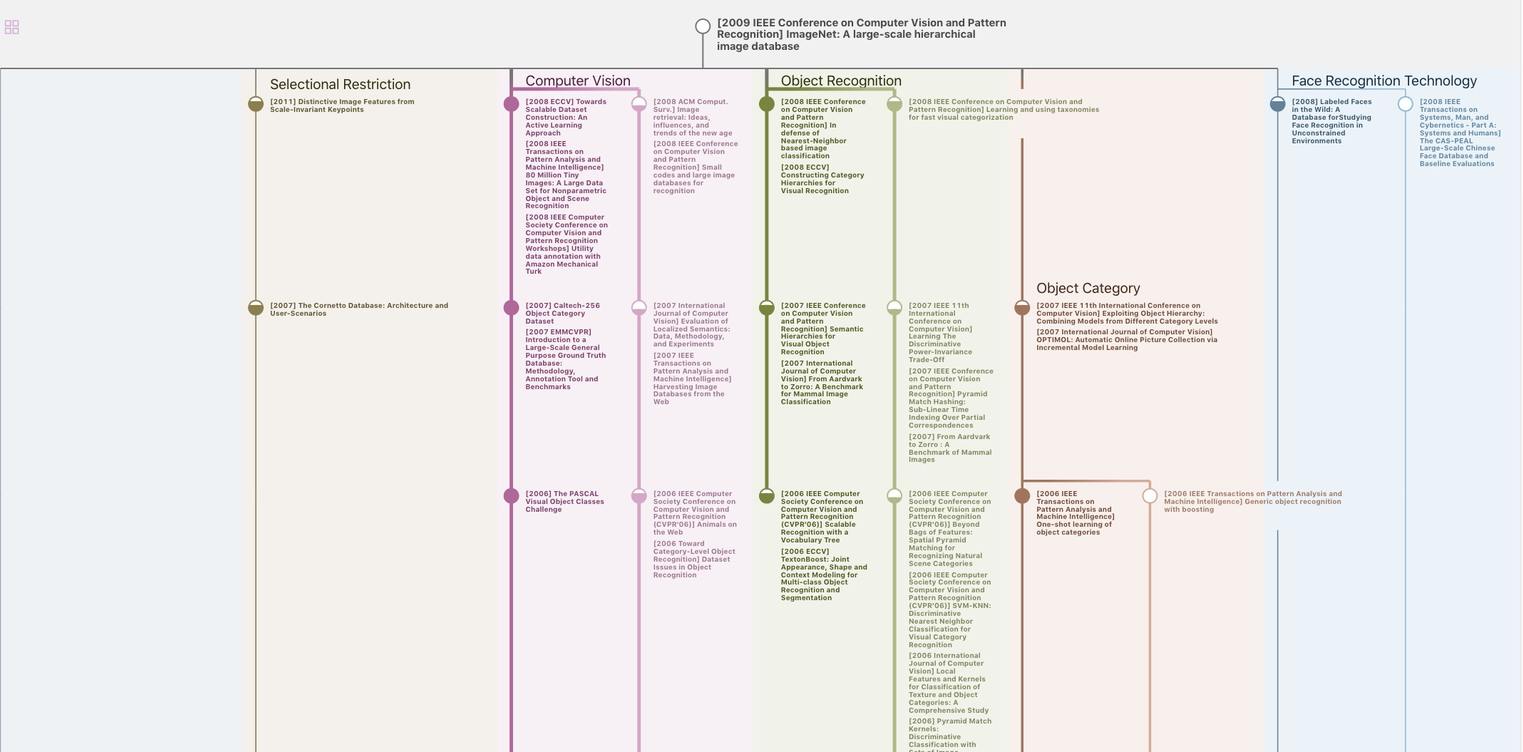
生成溯源树,研究论文发展脉络
Chat Paper
正在生成论文摘要