EM-style optimization of hidden conditional random fields for grapheme-to-phoneme conversion
Acoustics, Speech and Signal Processing(2011)
摘要
We have recently proposed an EM-style algorithm to optimize log-linear models with hidden variables. In this paper, we use this algorithm to optimize a hidden conditional random field, i.e., a conditional random field with hidden variables. Similar to hidden Markov models, the alignments are the hidden variables in the examples considered. Here, EM-style algorithms are iterative optimization algorithms which are guaranteed to improve the training criterion in each iteration without the need for tuning step sizes, sophisticated update schemes or numerical line optimization (with hardly predictable complexity). This is a rather strong property which conventional gradient-based optimization algorithms do not have. We present experimental results for a grapheme-to-phoneme conversion task and compare the convergence behavior of the EM-style algorithm with L-BFGS and Rprop.
更多查看译文
关键词
hidden Markov models,iterative methods,optimisation,speech recognition,EM-style optimization,L-BFGS,Rprop,conventional gradient-based optimization algorithms,grapheme-to-phoneme conversion task,hidden Markov models,hidden conditional random fields,iterative optimization algorithms,numerical line optimization,EM-style optimization,grapheme-to-phoneme conversion,hidden conditional random fields
AI 理解论文
溯源树
样例
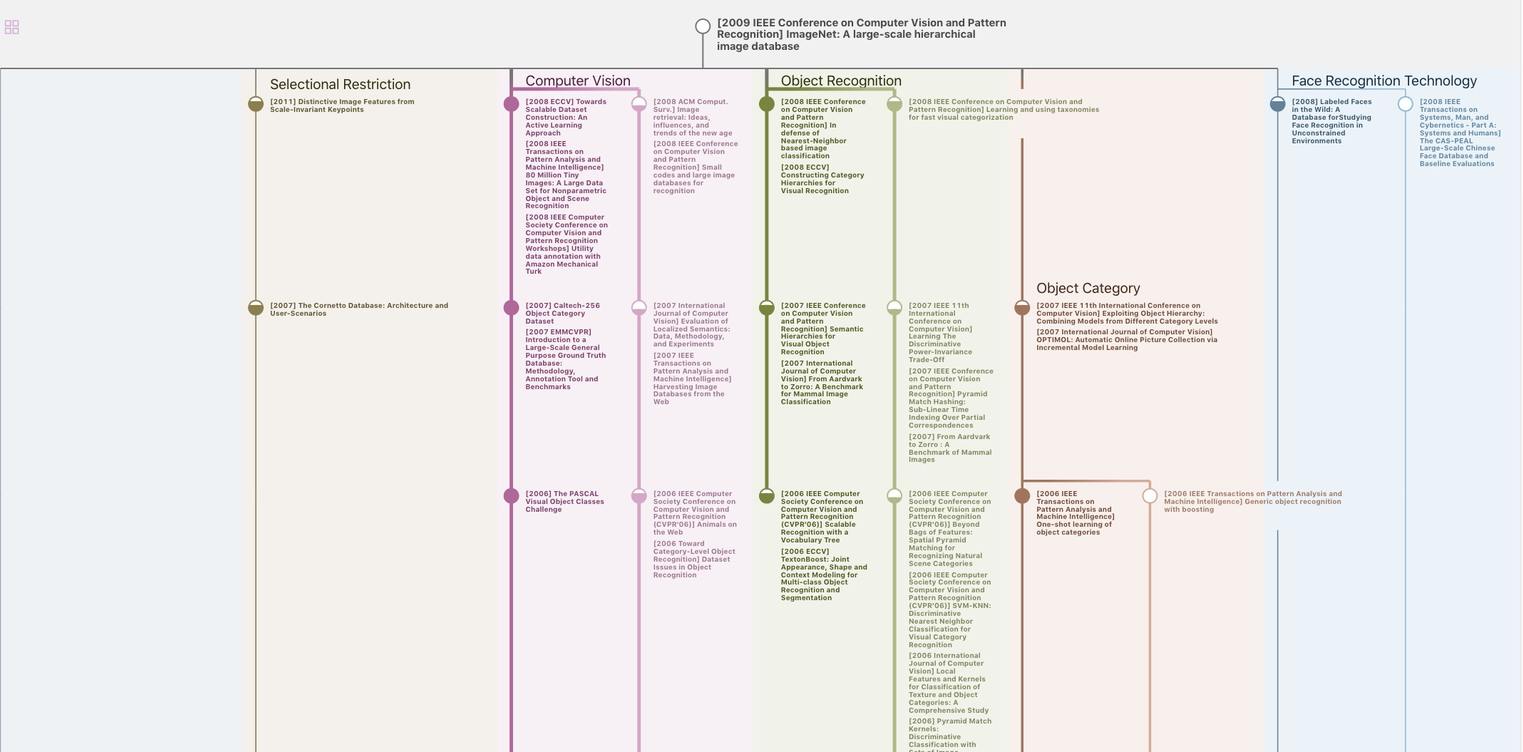
生成溯源树,研究论文发展脉络
Chat Paper
正在生成论文摘要