Boosting scalable gradient features for adaptive real-time tracking
Robotics and Automation(2011)
摘要
Recently, several image gradient and edge based features have been introduced. In unison, they all discovered that object shape is a strong cue for recognition and tracking. Generally their basic feature extraction relies on pixel-wise gradient or edge computation using discrete filter masks, while scale invariance is later achieved by higher level operations like accumulating histograms or abstracting edgels to line segments. In this paper we show a novel and fast way to compute region based gradient features which are scale invariant themselves. We developed specialized, quick learnable weak classifiers that are integrated into our adaptively boosted observation model for particle filter based tracking. With an ensemble of region based gradient features this observation model is able to reliably capture the shape of the tracked object. The observation model is adapted to new object and background appearances while tracking. Thus we developed advanced methods to decide when to update the model, or in other words, if the filter is on target or not. We evaluated our approach using the BoBoT1 as well as the PROST2 datasets.
更多查看译文
关键词
edge detection,feature extraction,gradient methods,image classification,object recognition,object tracking,particle filtering (numerical methods),BoBoT dataset,PROST dataset,adaptive real-time tracking,adaptively boosted observation model,background appearance,discrete filter masks,edge based features,edge computation,feature extraction,image gradient features,object recognition,object tracking,particle filter based tracking,pixel-wise gradient,region based gradient features,scalable gradient feature boosting,scale invariance,weak classifier
AI 理解论文
溯源树
样例
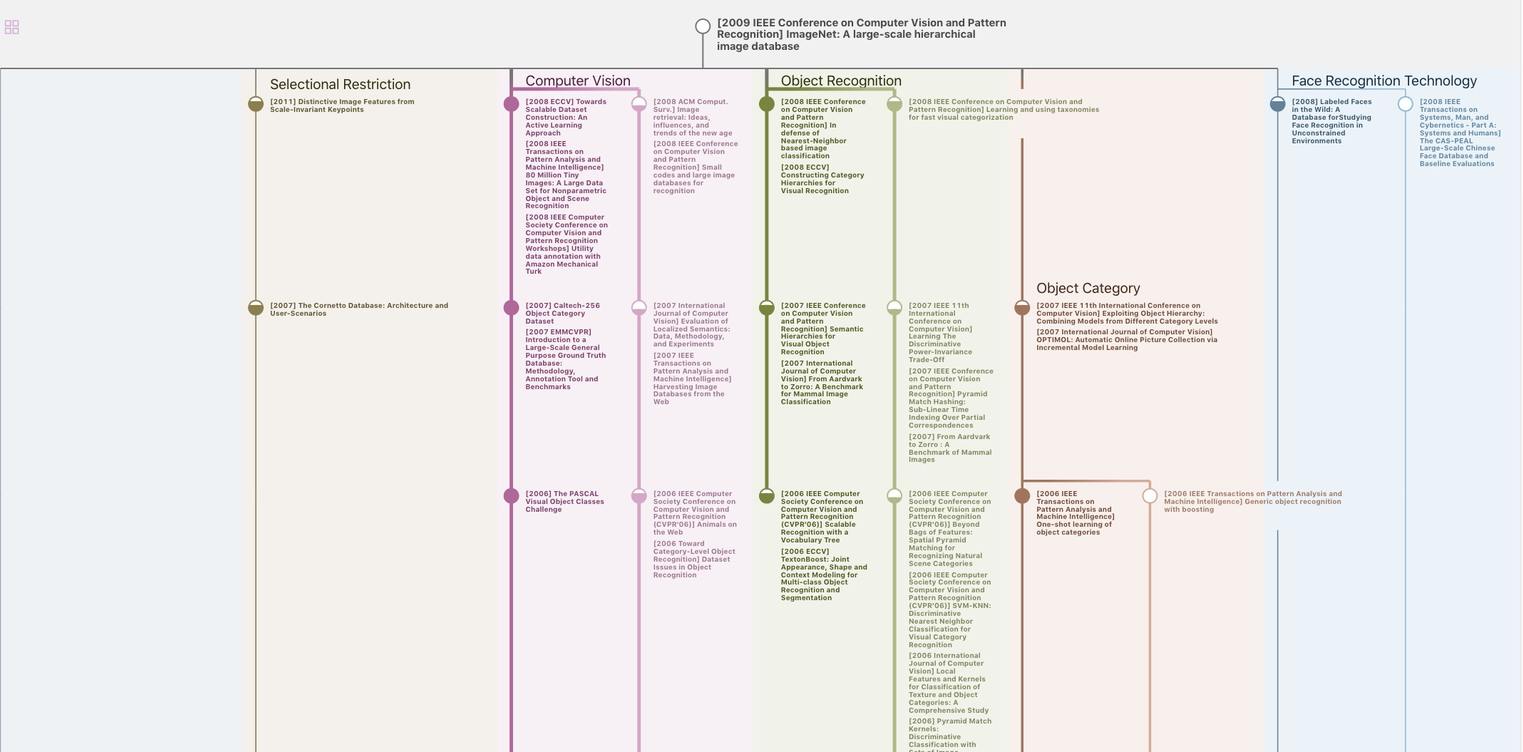
生成溯源树,研究论文发展脉络
Chat Paper
正在生成论文摘要