A compressed implicit Jacobian scheme for 3D regularized Gauss-Newton inversion
Antennas and Propagation(2011)
摘要
We present a compressed implicit Jacobian scheme for the regularized Gauss-Newton (GN) inversion algorithm for reconstructing three-dimensional conductivity distribution from electromagnetic data. In this scheme, the Jacobian matrix, whose storage usually requires a large amount of memory, is decomposed in terms of electric fields excited by sources located and oriented identically to the physical sources and receivers. As a result, the memory usage for the Jacobian matrix reduces from O (NSNRNP) to O [(NS + NR)NP], in which NS is the number of sources, NR is the number of receivers and NP is the number of parameters to be inverted. Further we applied the adaptive cross approximation (ACA) to compress these fields in order to further reduce the memory usage and to improve the efficiency of the GN method. In addition, the ACA error criterion is linked to the data misfit cost function so that we obtain a higher compression rate in early GN iterations. This compressed implicit Jacobian scheme provides a good balance between the memory usage and the computational time and makes the GN algorithm more efficient. We demonstrate the benefits of this scheme using synthetic and field data for geophysical electromagnetic applications.
更多查看译文
关键词
jacobian matrices,newton method,approximation theory,electromagnetic field theory,iterative methods,3d regularized gauss-newton inversion algorithm,aca error criterion,gn iterations,jacobian matrix,adaptive cross approximation,electric fields,electromagnetic data,geophysical electromagnetic applications,three-dimensional conductivity distribution reconstruction,conductivity,three dimensional,cost function,electromagnetics,computational complexity,electric field
AI 理解论文
溯源树
样例
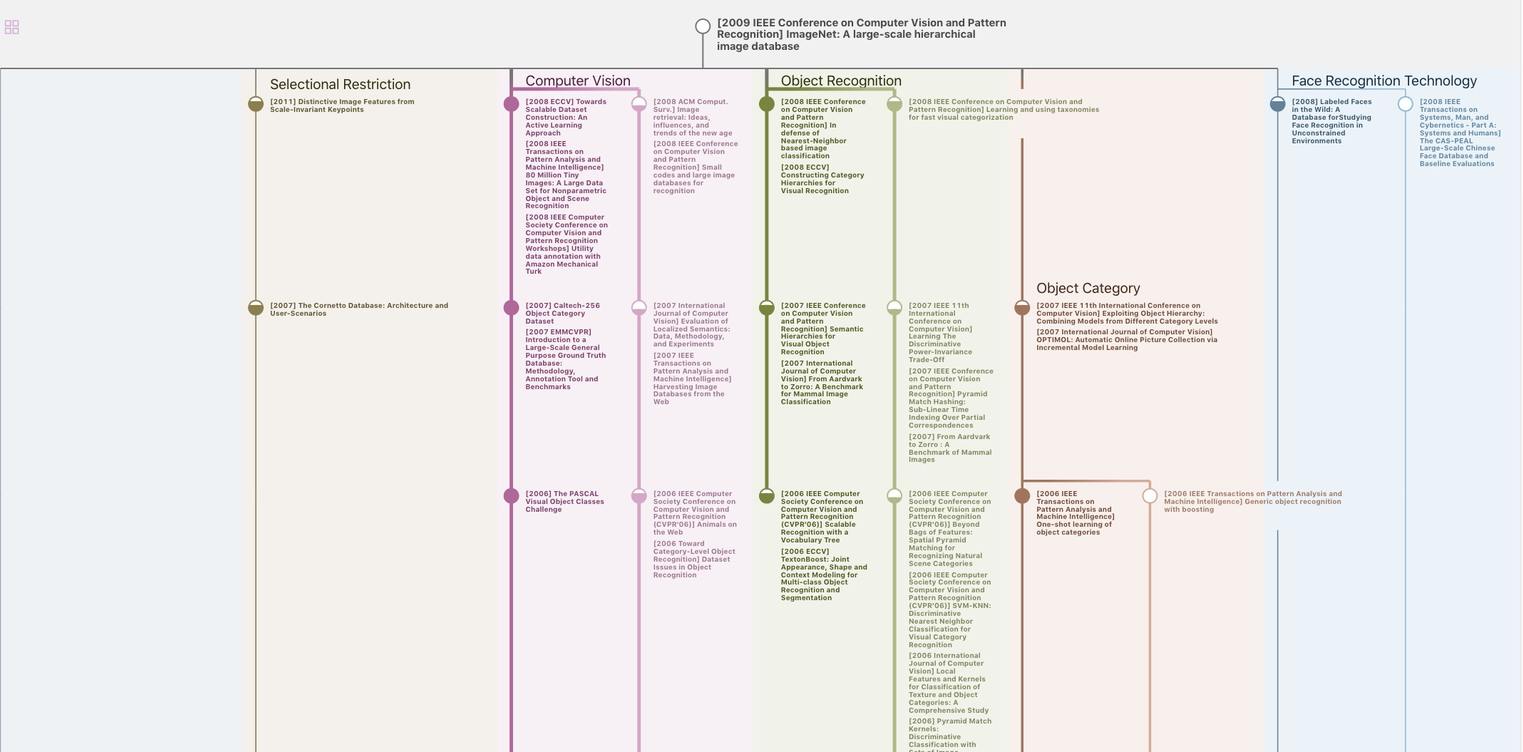
生成溯源树,研究论文发展脉络
Chat Paper
正在生成论文摘要