Super-Resolution Employing an Efficient Nonlocal Prior
Information Science and Cloud Computing Companion(2013)
摘要
In this paper, we propose a novel approach for multiframe super-resolution reconstruction by incorporating non-local prior in the maximum a posteriori (MAP) formulation. This prior expresses that recovered images tend to exhibit repetitive structures. A great deal of computation is required in the original non-local prior algorithm dealing with the huge amount of weight calculations. Techniques of weight symmetry, moving averaging filter, limited search window are adopted to speed up non-local filter. Meanwhile, Non-Linear Conjugated Gradient (NLCG) method is introduced to solve simultaneously the high-resolution (HR) image of optimization process and non-local prior adapted to the HR image. Experimental results on extensive synthetic and realistic images demonstrate the superiority of the proposed algorithm to representative algorithms both quantitatively and qualitatively.
更多查看译文
关键词
filtering theory,gradient methods,image reconstruction,image resolution,maximum likelihood estimation,optimisation,HR image,MAP,NLCG method,high-resolution image,limited search window,maximum a posteriori formulation,moving averaging filter,multiframe super-resolution reconstruction,nonlinear conjugated gradient method,nonlocal filter,nonlocal prior,optimization process,realistic images,synthetic images,weight symmetry,MAP,moving average filter,non-linear conjugated gradient,non-local means,non-local prior,super-resolution
AI 理解论文
溯源树
样例
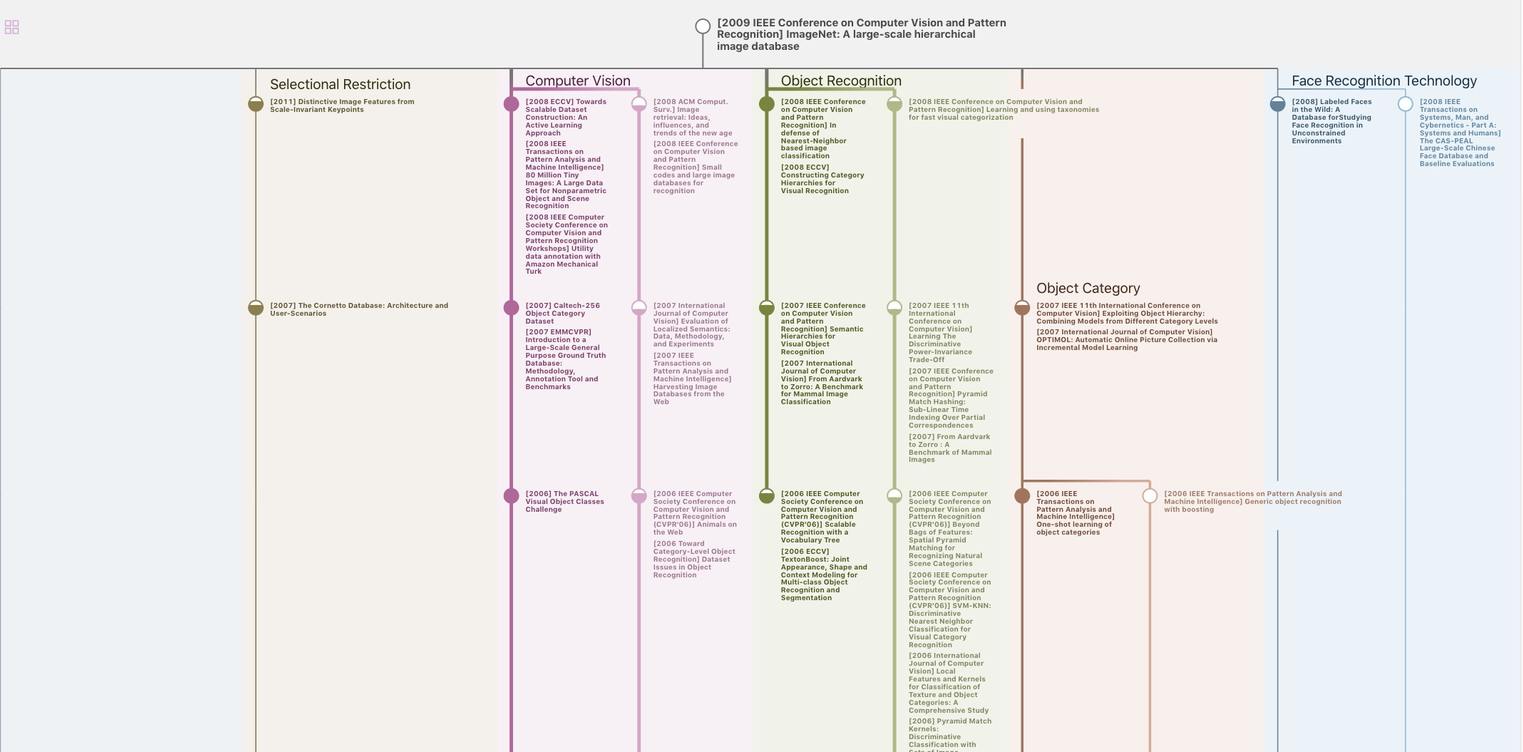
生成溯源树,研究论文发展脉络
Chat Paper
正在生成论文摘要