TOTAL VARIATION DENOISING USING POSTERIOR EXPECTATION
Lausanne(2008)
摘要
Total Variation image denoising, generally formulated in a variational setting, can be seen as a Maximum A Posteri- ori (MAP) Bayesian estimate relying on a simple explicit image prior. In this formulation, the denoised image is the most likely image of the posterior distribution, which fa- vors regularity and produces staircasing artifacts: in regions where smooth-varying intensities would be expected, con- stant zones appear separated by artificial boundaries. In this paper, we propose to use the Least Square Error (LSE) cri- terion instead of the MAP. This leads to a new denoising method called TV-LSE, that produces more realistic images by computing the expectation of the posterior distribution. We describe a Monte-Carlo Markov Chain algorithm based on Metropolis scheme, and provide an efficient convergence criterion. We also discuss the properties of TV-LSE, and show in particular that it does not suffer from the staircas- ing effect.
更多查看译文
关键词
image restoration,monte carlo markov chain,posterior distribution,total variation
AI 理解论文
溯源树
样例
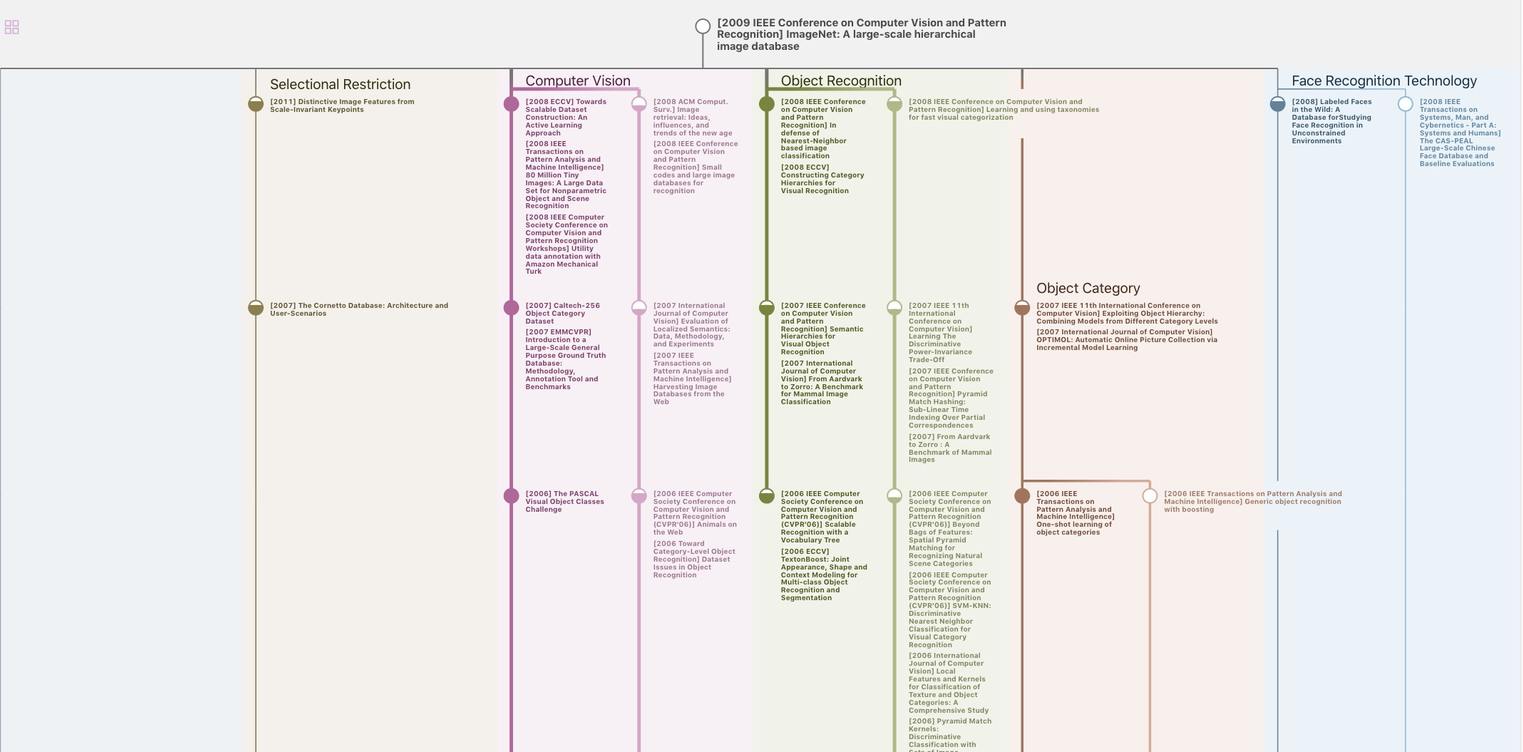
生成溯源树,研究论文发展脉络
Chat Paper
正在生成论文摘要