Signal Processing Approaches to Minimize or Suppress Calibration Time in Oscillatory Activity-Based Brain–Computer Interfaces
Proceedings of the IEEE(2015)
摘要
One of the major limitations of brain-computer interfaces (BCI) is their long calibration time, which limits their use in practice, both by patients and healthy users alike. Such long calibration times are due to the large between-user variability and thus to the need to collect numerous training electroencephalography (EEG) trials for the machine learning algorithms used in BCI design. In this paper, we first survey existing approaches to reduce or suppress calibration time, these approaches being notably based on regularization, user-to-user transfer, semi-supervised learning and a priori physiological information. We then propose new tools to reduce BCI calibration time. In particular, we propose to generate artificial EEG trials from the few EEG trials initially available, in order to augment the training set size. These artificial EEG trials are obtained by relevant combinations and distortions of the original trials available. We propose three different methods to do so. We also propose a new, fast and simple approach to perform user-to-user transfer for BCI. Finally, we study and compare offline different approaches, both old and new ones, on the data of 50 users from three different BCI data sets. This enables us to identify guidelines about how to reduce or suppress calibration time for BCI.
更多查看译文
关键词
brain-computer interfaces,calibration,electroencephalography,learning (artificial intelligence),medical signal processing,bci calibration time reduction,eeg,machine learning algorithms,oscillatory activity-based brain-computer interfaces,priori physiological information,semisupervised learning,signal processing approaches,user-to-user transfer,brain–computer interfaces (bci),brain???computer interfaces (bci),electroencephalography (eeg),machine learning,signal processing,small sample settings,learning artificial intelligence,training data,brain computer interfaces,band pass filters
AI 理解论文
溯源树
样例
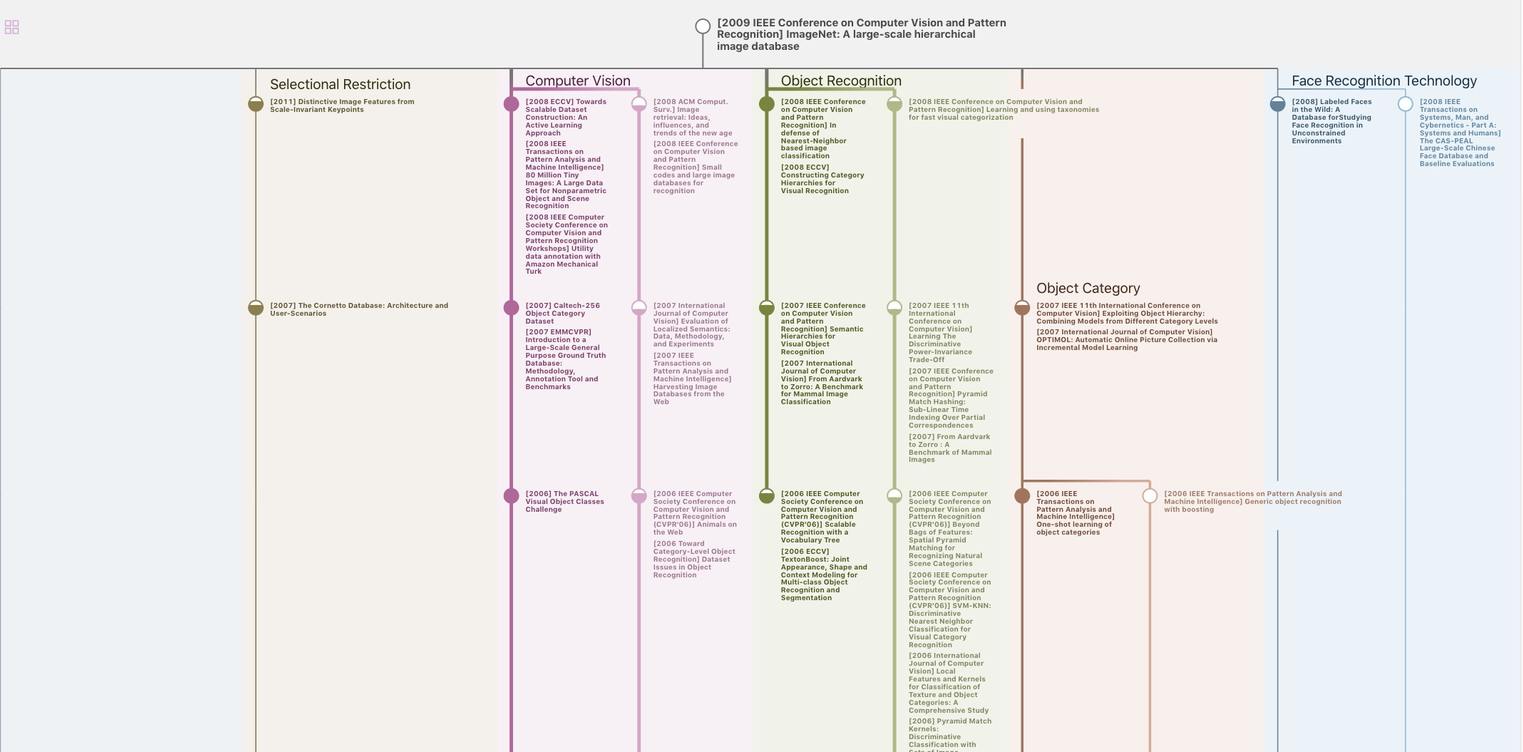
生成溯源树,研究论文发展脉络
Chat Paper
正在生成论文摘要