A population Monte Carlo method for Bayesian inference and its application to stochastic kinetic models
Barcelona(2011)
摘要
We introduce an extension of the population Monte Carlo (PMC) methodology to address the problem of Bayesian inference in high dimensional models. Specifically, we introduce a technique for the selection and update of importance functions based on the construction of Gaussian Bayesian networks. The structure of the latter graphical model enables a sequential sampling procedure that requires drawing only from unidimensional conditional distributions and leads to very efficient PMC algorithms. In order to illustrate the potential of the new technique we have considered the estimation of rate parameters in stochastic kinetic models (SKMs). SKMs are multivariate systems that model molecular interactions in biological and chemical problems. We present some numerical results based on a simple SKM known as predator-prey model.
更多查看译文
关键词
gaussian processes,monte carlo methods,belief networks,parameter estimation,predator-prey systems,sampling methods,bayesian inference,gaussian bayesian networks,pmc method,skm,high dimensional models,molecular interactions,multivariate systems,population monte carlo method,predator-prey model,rate parameter estimation,sequential sampling procedure,stochastic kinetic models,unidimensional conditional distributions,vectors,kinetic theory,sociology,stochastic processes
AI 理解论文
溯源树
样例
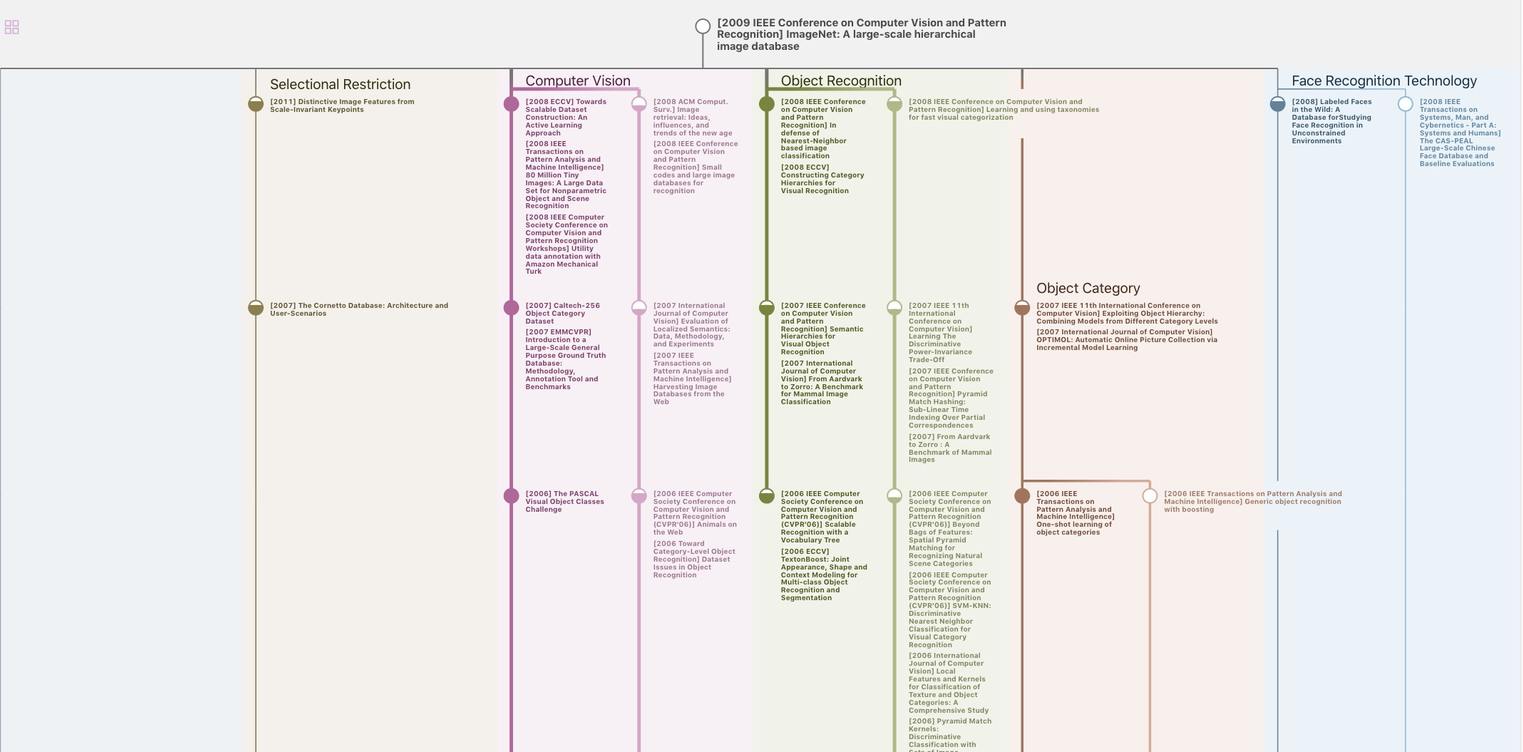
生成溯源树,研究论文发展脉络
Chat Paper
正在生成论文摘要