A-Optimal Projection for Image Representation
IEEE Trans. Pattern Anal. Mach. Intell.(2016)
摘要
We consider the problem of image representation from the perspective of statistical design. Recent studies have shown that images are possibly sampled from a low dimensional manifold despite of the fact that the ambient space is usually very high dimensional. Learning low dimensional image representations is crucial for many image processing tasks such as recognition and retrieval. Most of the existing approaches for learning low dimensional representations, such as Principal Component Analysis (PCA) and Locality Preserving Projections (LPP), aim at discovering the geometrical or discriminant structures in the data. In this paper, we take a different perspective from statistical experimental design, and propose a novel dimensionality reduction algorithm called A-Optimal Projection (AOP). AOP is based on a linear regression model. Specifically, AOP finds the optimal basis functions so that the expected prediction error of the regression model can be minimized if the new representations are used for training the model. Experimental results suggest that the proposed approach provides a better representation and achieves higher accuracy in image retrieval.
更多查看译文
关键词
dimensionality reduction,image representation,optimal design
AI 理解论文
溯源树
样例
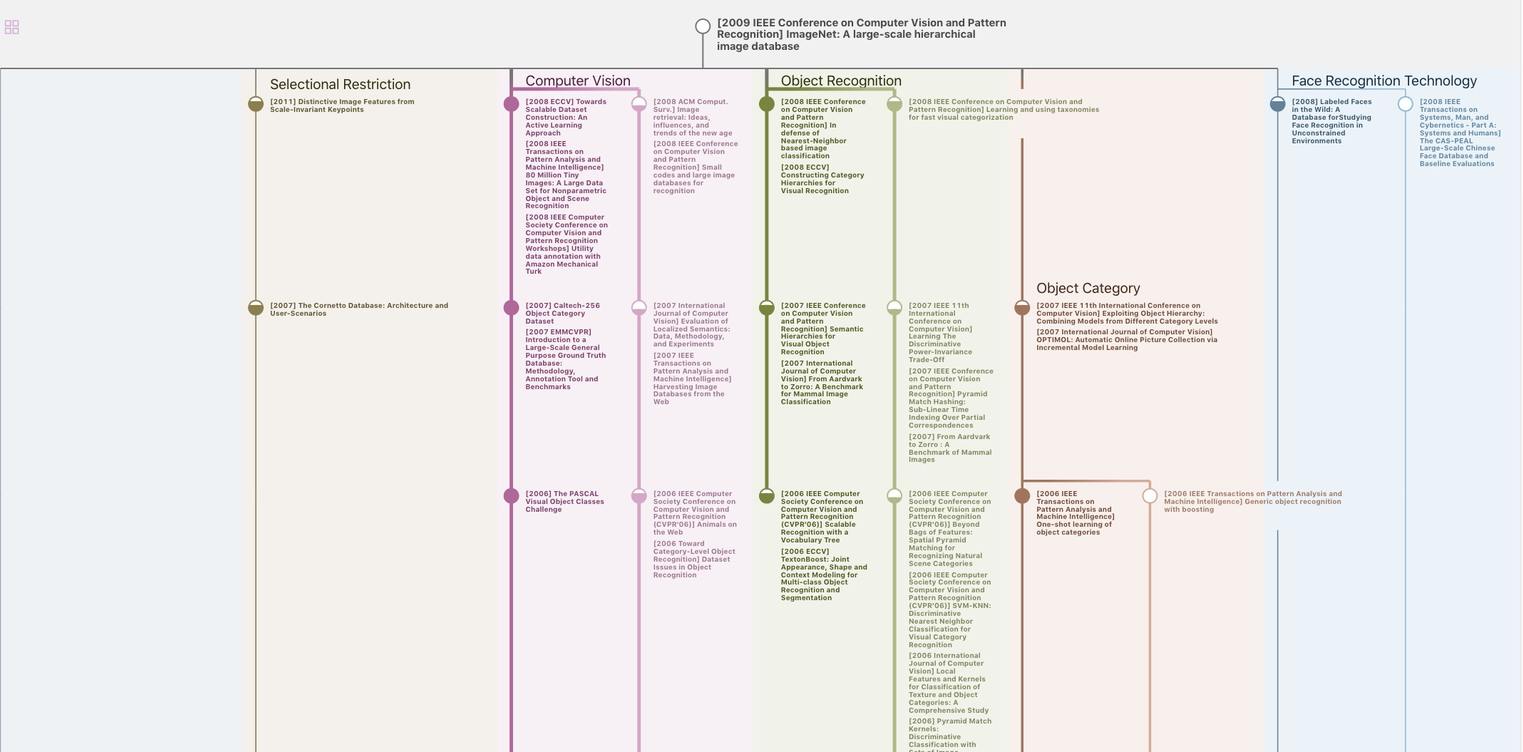
生成溯源树,研究论文发展脉络
Chat Paper
正在生成论文摘要