Fast computation of hemodynamic sensitivity to lumen segmentation uncertainty
Medical Imaging, IEEE Transactions(2015)
摘要
Patient-specific blood flow modeling combining imaging data (such as CT scans) and computational fluid dynamics can aid in the assessment of functional significance of coronary artery disease. Accurate coronary segmentation and realistic physiologic modeling of boundary conditions are important steps to ensure a high diagnostic performance. Segmentation of the coronary arteries can be constructed by a combination of automated algorithms with human review and editing. However, blood pressure and flow are not impacted equally by different local sections of the coronary artery tree. Focusing human review and editing towards regions that will most affect the subsequent simulations can significantly accelerate the review process. We define geometric sensitivity as the standard deviation in hemodynamics-derived metrics due to uncertainty in lumen segmentation. We develop a machine learning framework for estimating the geometric sensitivity in real time. Features used in the machine learning algorithm are split into geometric, clinical, and reduced-order models. We develop an anisotropic kernel regression method for assessment of lumen narrowing score, which is used as a feature in the machine learning algorithm. A multi-resolution sensitivity algorithm is introduced to hierarchically refine regions of high sensitivity so that we can quantify sensitivities to a desired spatial resolution. We show that the mean absolute error of the machine learning algorithm compared to 3D simulations is less than 0.01. We further demonstrate that sensitivity is not predicted simply by anatomic reduction but also encodes information about hemodynamics which in turn depends on downstream boundary conditions. We then calculate the dependence of allowable coefficient of variation in lumen area to maximum allowable sensitivity. This sensitivity approach can be extended to other systems such as cerebral flow, electro-mechanical simulations, etc.
更多查看译文
关键词
blood flow simulations,machine learning,multi-resolution analysis,segmentation accuracy,sensitivity analysis,uncertainty,geometry,sensitivity,stochastic processes,bifurcation
AI 理解论文
溯源树
样例
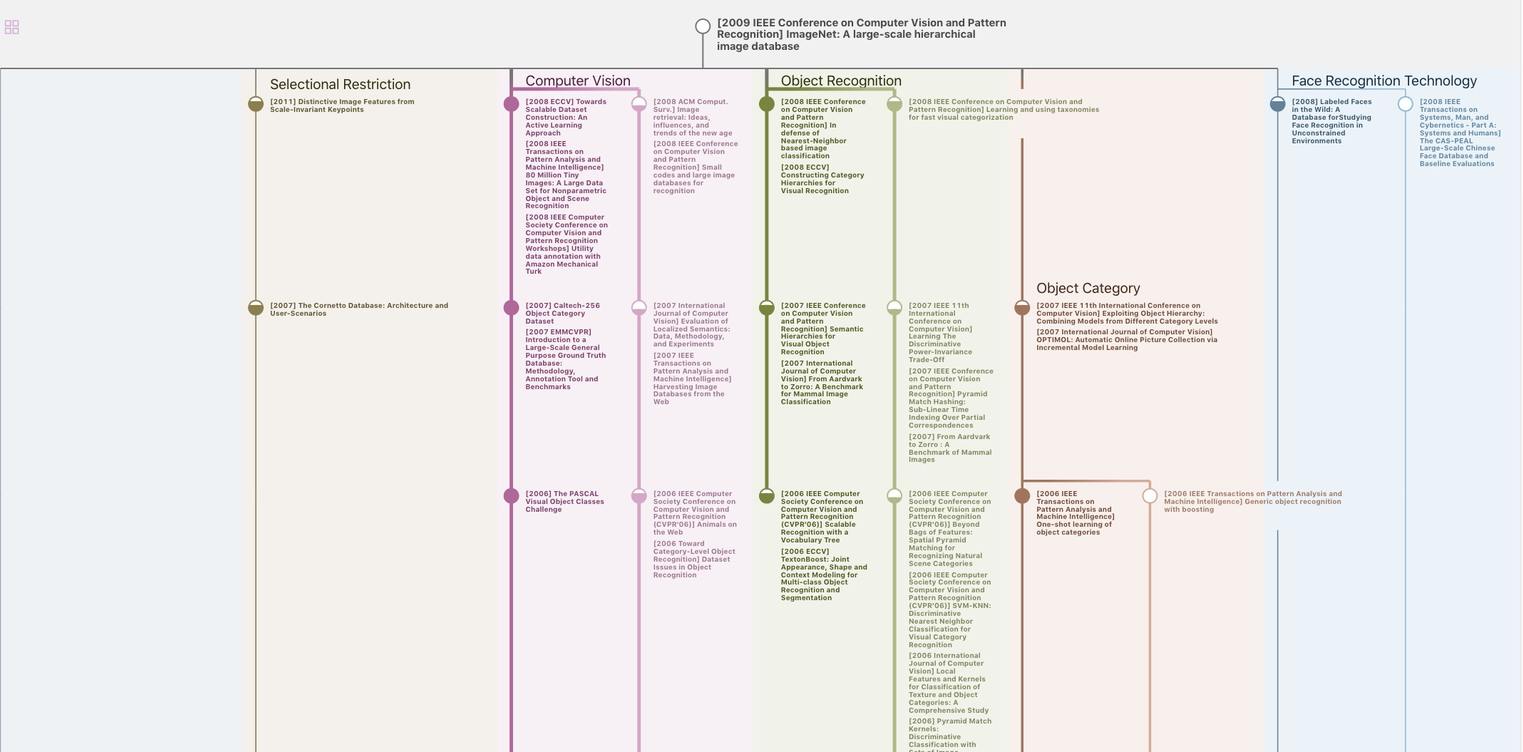
生成溯源树,研究论文发展脉络
Chat Paper
正在生成论文摘要