An efficient classifier ensemble using SVM
Delhi(2009)
摘要
Recently ensemble classification has attracted serious attention of machine learning community as a solution for improving classification accuracy. The effect of the strategies for generating the members, combining the predictions and the size of the ensemble on the accuracy of the ensemble are of utmost interest to the researchers. In this paper, we propose and empirically evaluate a novel method for generating members of ensemble based on 'learning-from-mistakes' paradigm. SVM is used as the base learner, and a series of dependent classifiers is obtained using model based instance selection method. In each iteration, all the wrongly classified records are merged with the support vectors to capture diversity. Classifiers with accuracy higher than the average of the series are selected for ensemble construction. Simple majority voting has been used to combine learners. The approach has been empirically found efficient as very few classifiers need to be generated and even fewer are selected. Accuracy on the test sets is comparable to bagging and boosting based methods.
更多查看译文
关键词
iterative methods,learning (artificial intelligence),pattern classification,support vector machines,svm,bagging based method,boosting based method,dependent classifiers,efficient classifier ensemble,iteration,learning-from-mistakes paradigm,machine learning community,model based instance seletion method,support vector machine,wrongly classified records,classifier ensemble,diversity,majority voting,learning artificial intelligence,machine learning,support vector
AI 理解论文
溯源树
样例
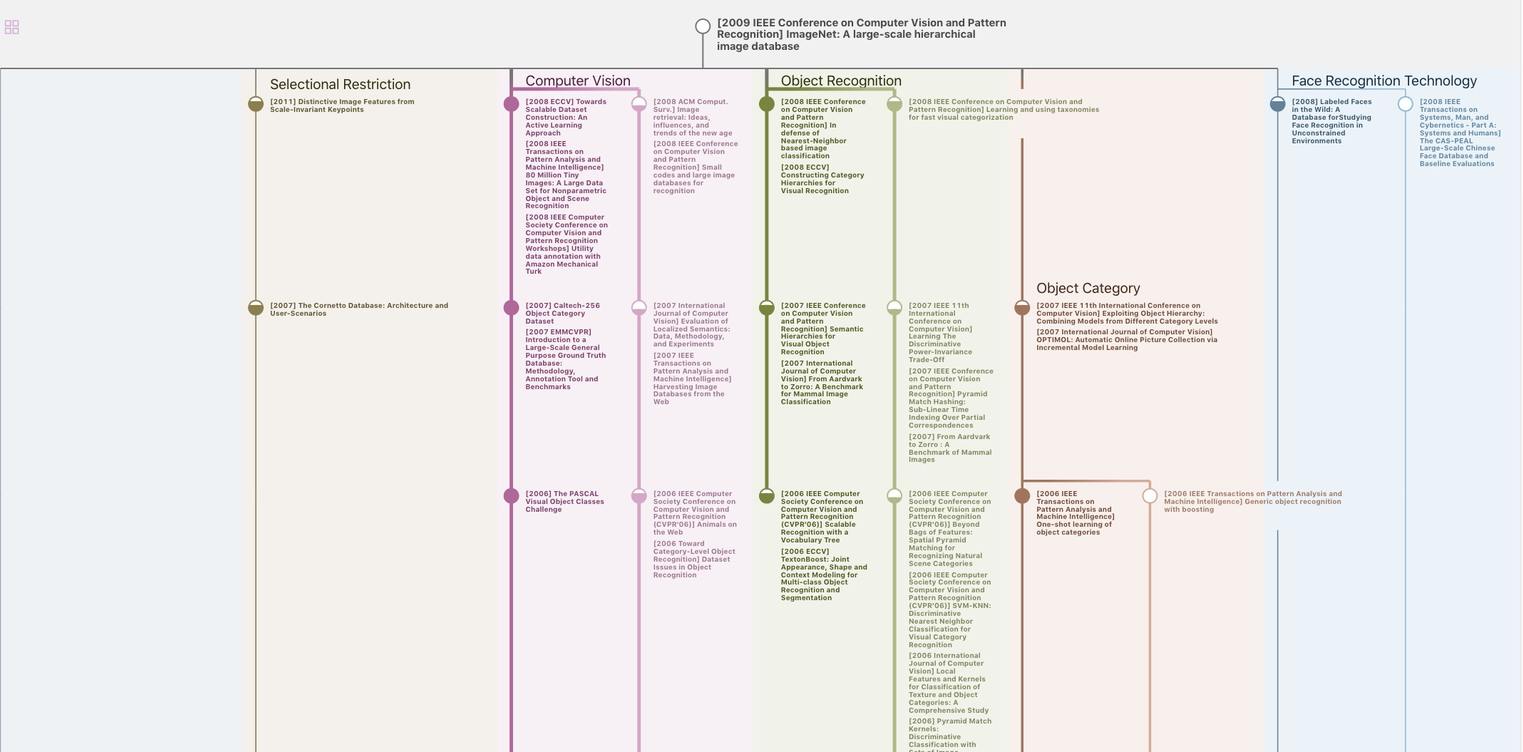
生成溯源树,研究论文发展脉络
Chat Paper
正在生成论文摘要