Weighted conditional mutual information based boosting for classification of imbalanced datasets
Pattern Recognition(2012)
摘要
This paper addresses the problem of binary classifier learning when the training data is imbalanced, i.e. the samples of the two classes have significantly different cardinality. We investigate two different cost-sensitive approaches in the conditional mutual information (CMI) based weak classifier selection procedure using histogram descriptors. The first method uses CMI for classifier selection, and cost factors are utilized in the construction of the final boosted classifier using support vector machine learning. In the second approach these costs are incorporated into the classifier selection step by weighting the CMI (wCMI). We evaluate the proposed methods in object recognition and detection tasks using two popular histogram-like descriptors. Extensive experiments showed that the proposed methods provide efficient tools to address both problems.
更多查看译文
关键词
image classification,learning (artificial intelligence),object detection,object recognition,support vector machines,CMI-based weak classifier selection procedure,binary classifier learning,boosted classifier,conditional mutual information-based weak classifier selection procedure,cost-sensitive approaches,histogram descriptors,histogram-like descriptors,imbalanced dataset classification,object detection,object recognition,support vector machine learning,training data,weighted conditional mutual information-based boosting
AI 理解论文
溯源树
样例
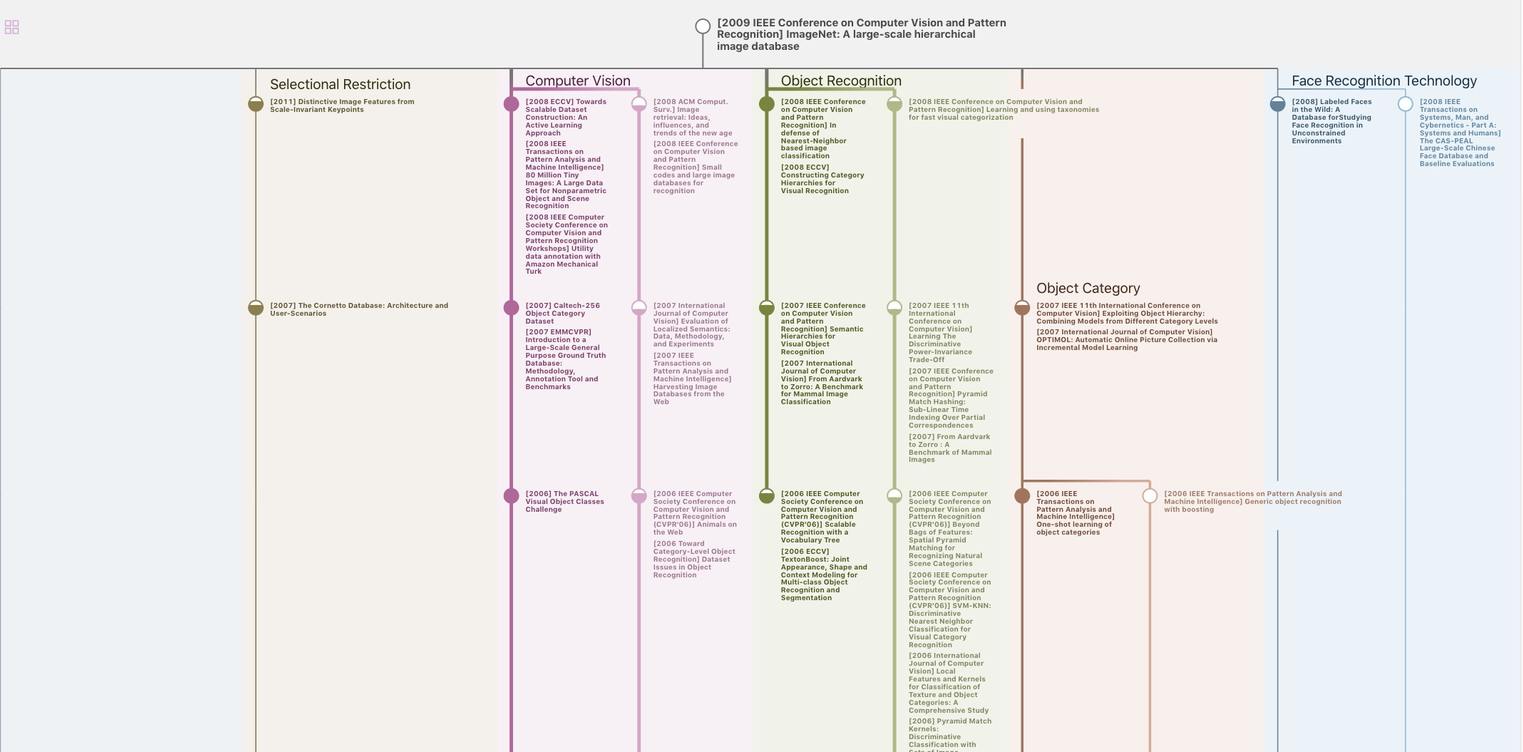
生成溯源树,研究论文发展脉络
Chat Paper
正在生成论文摘要