A supervised learning approach to far range depth estimation using a consumer-grade RGB-D camera
Electronics, Computing and Communication Technologies(2013)
摘要
This paper describes an approach to learn far range depth data from a consumer grade RGB-D sensor. Raw sensor depth data is limited to the near field and is used as ground truth by a supervised learning algorithm for predicting far range depth. A multiple hypothesis regression function is fit using the near range ground truth. A linear least squares solution determines the parameters of each function in the hypothesis vector. The “best fit” function is chosen as the one which minimizes the error between the predicted depth and the actual depth. This is used to populate a lookup table with learned far range depth data, extending sensor range. A novelty detection scheme estimates change in terrain inclination and is used to repopulate the lookup table of depth data for varying ground plane inclinations. Learning depth extension has been tested in heterogeneous indoor and outdoor environments, with diverse terrain type, varying terrain inclination and height, and under different obstacle field configurations.
更多查看译文
关键词
cameras,image colour analysis,image sensors,learning (artificial intelligence),least squares approximations,mobile robots,regression analysis,robot vision,table lookup,terrain mapping,actual depth,best fit function,consumer-grade rgb-d camera,far range depth estimation,ground plane inclinations,heterogeneous indoor environments,heterogeneous outdoor environments,hypothesis vector,linear least squares solution,lookup table,multiple hypothesis regression function,near range ground truth,novelty detection scheme,obstacle field configurations,predicted depth,raw sensor depth data,supervised learning algorithm,supervised learning approach,terrain inclination,autonomous robots,rgb-d camera,range extension,supervised learning,learning artificial intelligence
AI 理解论文
溯源树
样例
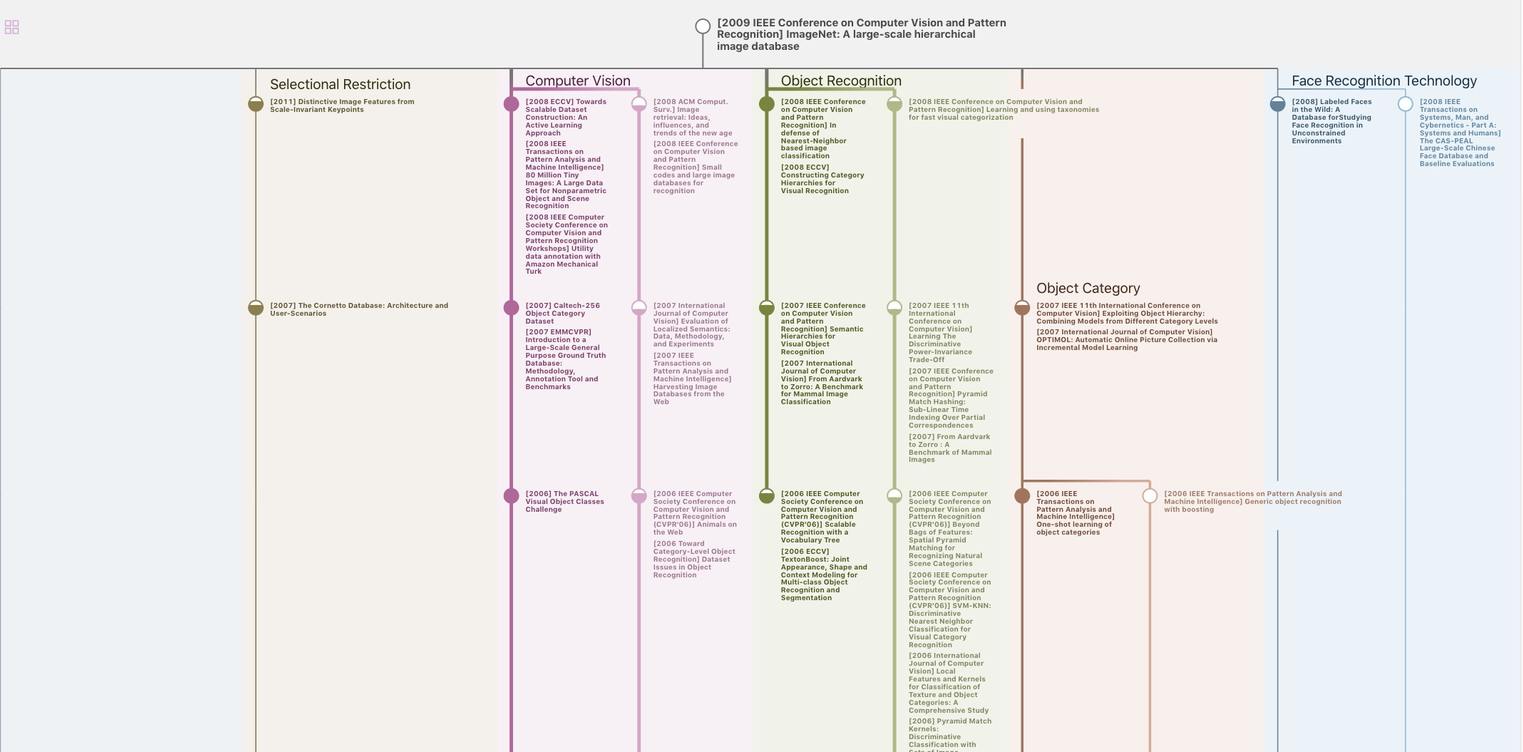
生成溯源树,研究论文发展脉络
Chat Paper
正在生成论文摘要