Automatic skill acquisition in Reinforcement Learning using connection graph stability centrality
Circuits and Systems(2010)
摘要
Reinforcement Learning (RL) is an approach for training agent's behavior through trial-and-error interactions with a dynamic environment. An important problem of RL is that in large domains an enormous number of decisions are to be made. Hence, instead of learning using individual primitive actions, an agent could learn much faster if it could form high level behaviors known as skills. Graph-based approach, that maps the RL problem to a graph, is one of the several approaches proposed to identify the skills to learn automatically. In this paper we propose a new centrality measure for identifying bottleneck nodes crucial to develop useful skills. We will show through simulations for two benchmark tasks, namely, “two-room grid” and “taxi driver” that a procedure based on the proposed measure performs better than the procedure based on closeness and node betweenness centrality.
更多查看译文
关键词
graph theory,learning (artificial intelligence),automatic skill acquisition,benchmark tasks,connection graph stability centrality,dynamic environment,high level behaviors,individual primitive actions,reinforcement learning,training agent behavior,trial-and-error interactions,two-room grid
AI 理解论文
溯源树
样例
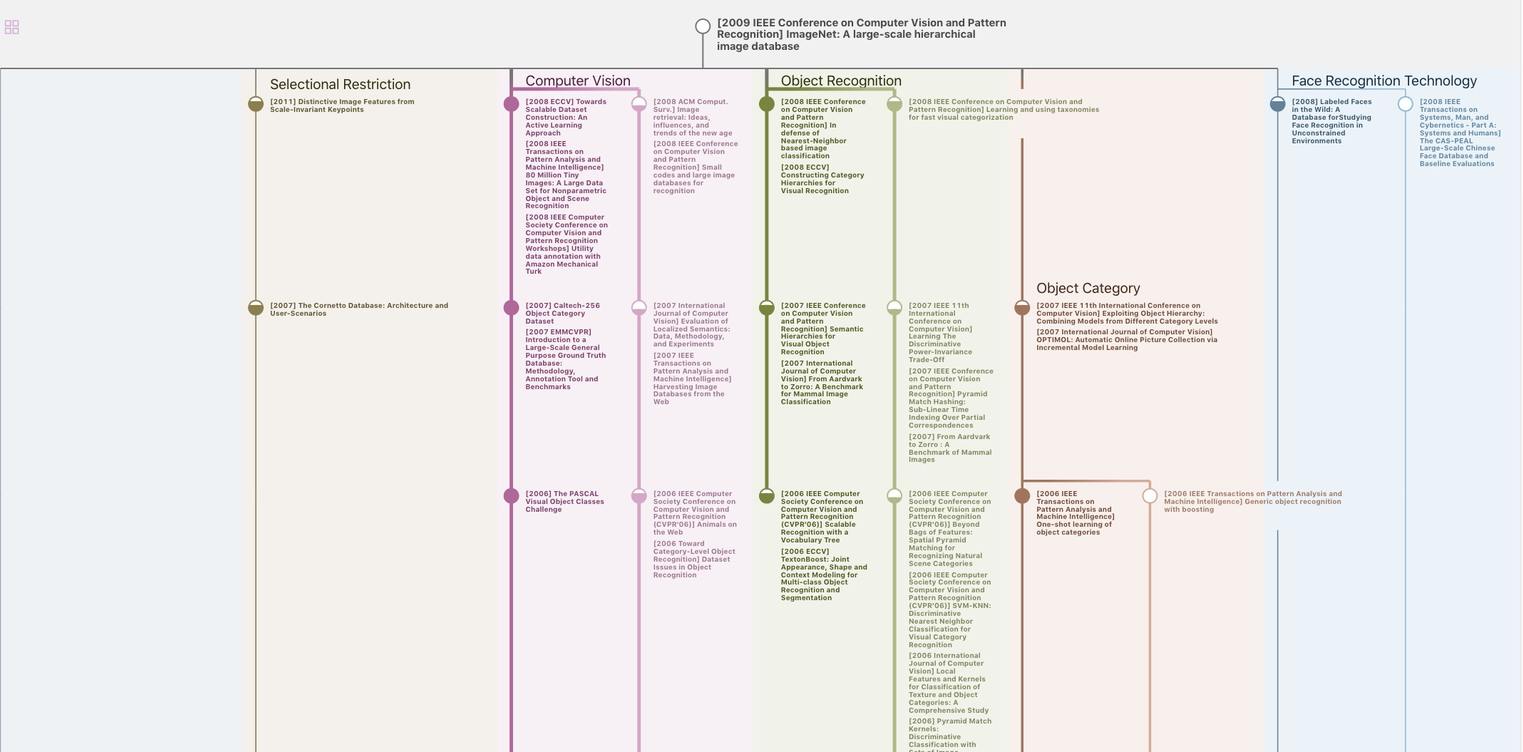
生成溯源树,研究论文发展脉络
Chat Paper
正在生成论文摘要