Efficient Use of Geographically Spread Cloud Resources
Cluster, Cloud and Grid Computing(2013)
摘要
The demand for cloud services in each geographical location changes over time depending on the time of the day. Thus, when one data center (say in the east coast of the US) experiences peak load, other data centers (say in Europe) experience lower load. This paper addresses the efficiency of load sharing between geographically spread cloud resources. We observe that despite the network latency, for several common services it is very beneficial to share the load across two or more data centers, each located in a different time zone. We rigorously analyze a simple setting in which customers can be redirected between two servers, each experiencing a different local load. We show that a threshold-based load sharing scheme, in which loads are redirected when exceeding some threshold, is significantly more efficient than a static load sharing scheme, where loads are redirected independently of the current state. Our load sharing techniques can reduce the average service time by 40%during peak demand in typical service scenarios. Looking at the same result from a different perspective, we show that (in the same setting) deploying our geographically based load sharing scheme can provide similar user experience with 15%-20% less resources. To further validate our approach, we deployed Wikipedia instances on Amazon EC2 both in Europe and the US and tested our techniques using real Wikimedia access logs. Our results show that threshold-based load sharing between the US and Europe, achieves an improvement of up to 32% in average service time over these logs.
更多查看译文
关键词
Web sites,cloud computing,computer centres,resource allocation,Amazon EC2,Europe,US,Wikimedia access log,Wikipedia,cloud service,data center,geographical location,geographically based load sharing scheme,geographically spread cloud resource,local load,network latency,static load sharing,threshold-based load sharing,Cloud computing,Delay aware load sharing,Resource allocation
AI 理解论文
溯源树
样例
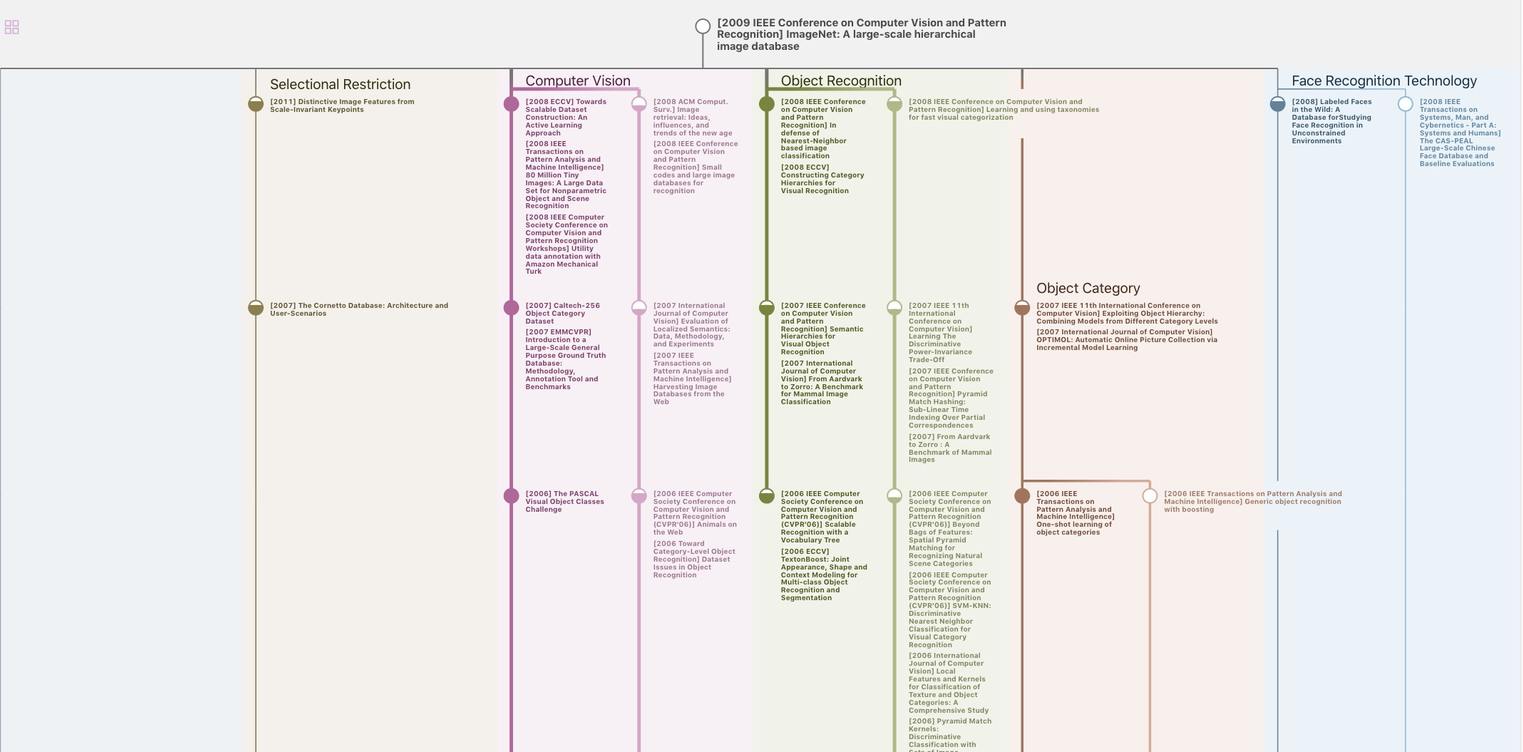
生成溯源树,研究论文发展脉络
Chat Paper
正在生成论文摘要