Kernel Recursive Least Squares-Type Neuron for Nonlinear Equalization
2013 21ST IRANIAN CONFERENCE ON ELECTRICAL ENGINEERING (ICEE)(2013)
摘要
The nonlinear channel distotions and the nonminum phase channel characteristics modelling, are a significant part in channel equalization problems. on the other hand, the nonlinear system requiring equalization is often noninvertible, resulting in a drastic loss of information. So far, Hammerstein and wiener models, Artificial Neural Networks (ANN), radial basis function (RBF) have been widely used as nonlinear methods in different applications, such as equalization. The kernel methods are well known for their great modelling capacity of nonlinear systems in addition to their modest complexity. A new kernel recursive least square-type neuron (NKRLS) equalizer is proposed which improves aforementioned nonlinear methods problems such as, classical training algorithm drawbacks to parameter definition, slow convergence, local minima, non-convex optimization, loss of universal approximation. NKRLS does that thanks to its nonparametric and universal approximation properties. NKRLS cosnsists of Kenel recursive least square followed by a simple neuron. In the first part of paper the new proposed KRLS-type neuron algorithm is introduced. The second part of paper corroborates our results with simulation results.
更多查看译文
关键词
Reproducing Kernel Hilbert spaces,Kernel recursive least squares,Neural network,Equlization
AI 理解论文
溯源树
样例
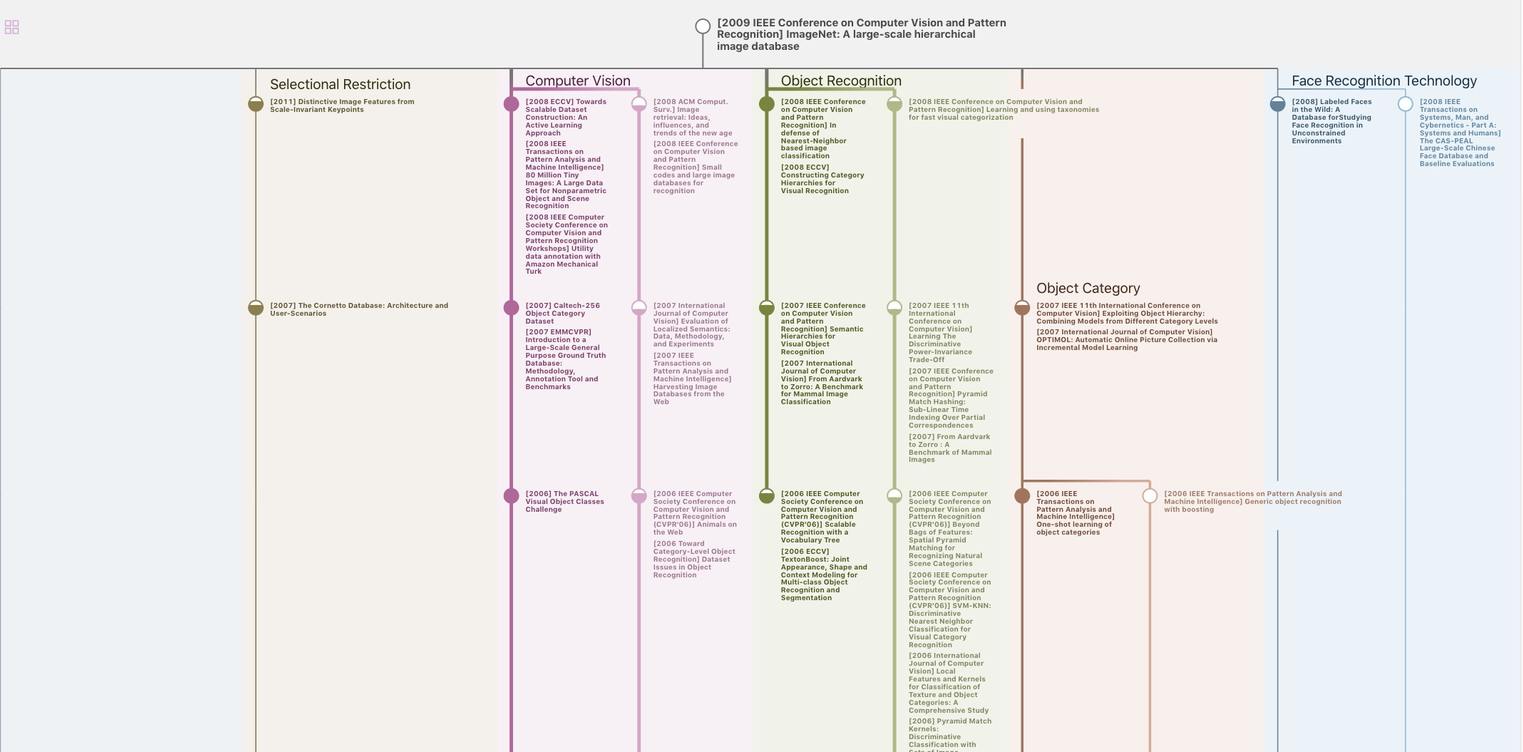
生成溯源树,研究论文发展脉络
Chat Paper
正在生成论文摘要