VarSight: Prioritizing Clinically Reported Variants with Binary Classification Algorithms
bioRxiv (Cold Spring Harbor Laboratory)(2019)
摘要
Abstract Motivation In genomic medicine for rare disease patients, the primary goal is to identify one or more variants that cause their disease. Typically, this is done through filtering and then prioritization of variants for manual curation. However, prioritization of variants in rare disease patients remains a challenging task due to the high degree of variability in phenotype presentation and molecular source of disease. Thus, methods that can identify and/or prioritize variants to be clinically reported in the presence of such variability are of critical importance. Results We tested the application of classification algorithms that ingest variant predictions along with phenotype information for predicting whether a variant will ultimately be clinically reported and returned to a patient. To test the classifiers, we performed a retrospective study on variants that were clinically reported to 237 patients in the Undiagnosed Diseases Network. We treated the classifiers as variant prioritization systems and compared them to another variant prioritization algorithm and two single-measure controls. We showed that these classifiers outperformed the other methods with the best classifier ranking 73% of all reported variants and 97% of reported pathogenic variants in the top 20. Availability The scripts used to generate results presented in this paper are available at https://github.com/HudsonAlpha/VarSight . Contact jholt@hudsonalpha.org
更多查看译文
关键词
prioritizing clinically,variants,binary classification algorithms,classification algorithms
AI 理解论文
溯源树
样例
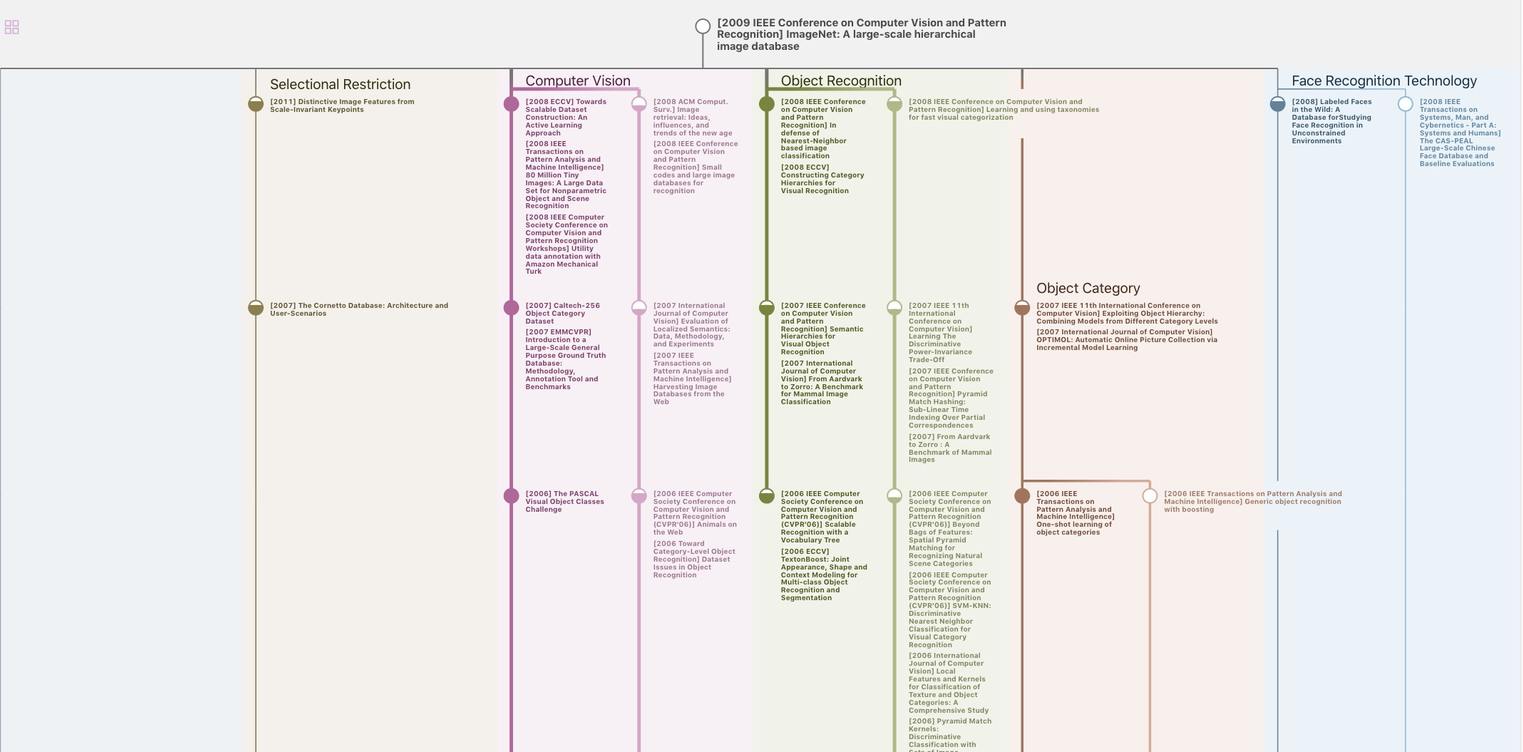
生成溯源树,研究论文发展脉络
Chat Paper
正在生成论文摘要