Sample-efficient learning with auxiliary class-label information.
AMIA ... Annual Symposium proceedings / AMIA Symposium. AMIA Symposium(2013)
摘要
Building classification models from clinical data collected for past patients often requires additional example labeling and annotation by a human expert. Since example labeling may require to review a complete electronic health record the process can be very time consuming and costly. To make the process more cost-efficient, the number of examples an expert needs to label should be reduced. We develop and test a new approach for the classification learning in which, in addition to class labels provided by an expert, the learner is provided with auxiliary information that reflects how strong the expert feels about the class label. We show that this information can be extremely useful for practical classification tasks based on human assessment and can lead to improved learning with a smaller number of examples. We develop a new classification approach based on the support vector machines and the learning to rank methodologies capable of utilizing the auxiliary information during the model learning process. We demonstrate the benefit of the approach on the problem of learning an alert model for Heparin Induced Thrombocytopenia (HIT) by showing an improved classification performance of the models that are trained on a smaller number of labeled examples.
更多查看译文
关键词
artificial intelligence,algorithms,classification,regression analysis,support vector machines
AI 理解论文
溯源树
样例
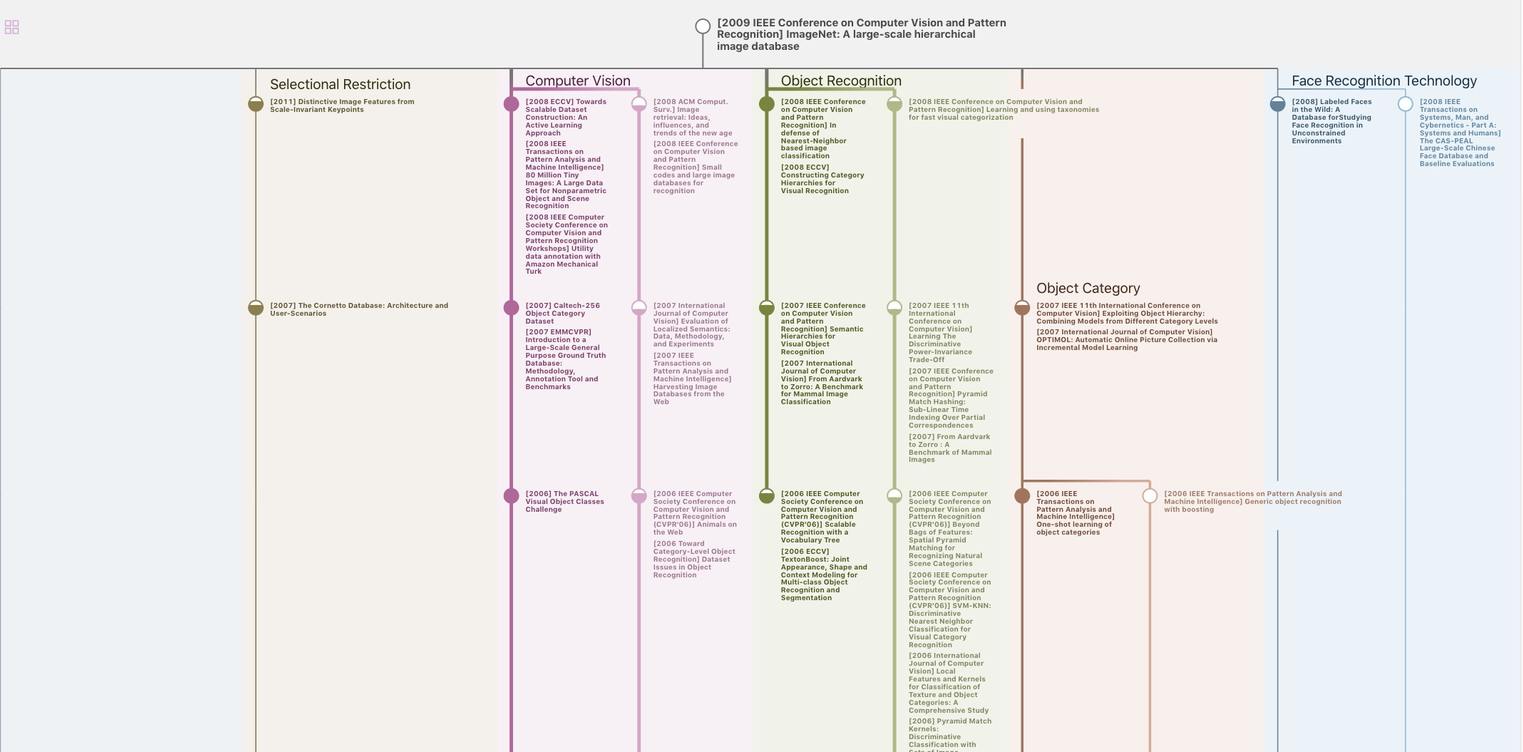
生成溯源树,研究论文发展脉络
Chat Paper
正在生成论文摘要